Neural Copula: A unified framework for estimating generic high-dimensional Copula functions
arXiv (Cornell University)(2022)
摘要
The Copula is widely used to describe the relationship between the marginal distribution and joint distribution of random variables. The estimation of high-dimensional Copula is difficult, and most existing solutions rely either on simplified assumptions or on complicating recursive decompositions. Therefore, people still hope to obtain a generic Copula estimation method with both universality and simplicity. To reach this goal, a novel neural network-based method (named Neural Copula) is proposed in this paper. In this method, a hierarchical unsupervised neural network is constructed to estimate the marginal distribution function and the Copula function by solving differential equations. In the training program, various constraints are imposed on both the neural network and its derivatives. The Copula estimated by the proposed method is smooth and has an analytic expression. The effectiveness of the proposed method is evaluated on both real-world datasets and complex numerical simulations. Experimental results show that Neural Copula's fitting quality for complex distributions is much better than classical methods. The relevant code for the experiments is available on GitHub. (We encourage the reader to run the program for a better understanding of the proposed method).
更多查看译文
关键词
neural copula,high-dimensional
AI 理解论文
溯源树
样例
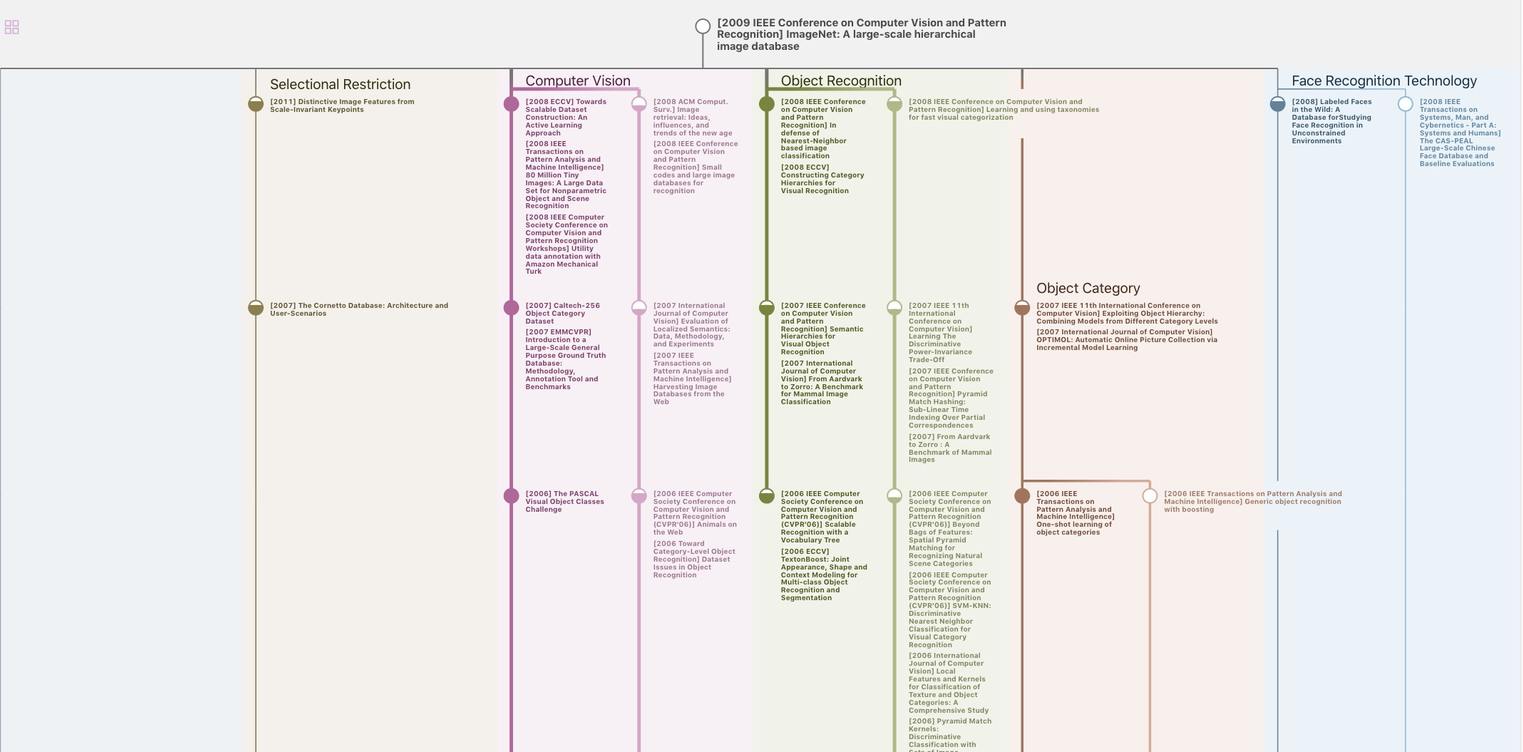
生成溯源树,研究论文发展脉络
Chat Paper
正在生成论文摘要