Concentration inequalities for correlated network-valued processes with applications to community estimation and changepoint analysis
arXiv (Cornell University)(2022)
摘要
Network-valued time series are currently a common form of network data. However, the study of the aggregate behavior of network sequences generated from network-valued stochastic processes is relatively rare. Most of the existing research focuses on the simple setup where the networks are independent (or conditionally independent) across time, and all edges are updated synchronously at each time step. In this paper, we study the concentration properties of the aggregated adjacency matrix and the corresponding Laplacian matrix associated with network sequences generated from lazy network-valued stochastic processes, where edges update asynchronously, and each edge follows a lazy stochastic process for its updates independent of the other edges. We demonstrate the usefulness of these concentration results in proving consistency of standard estimators in community estimation and changepoint estimation problems. We also conduct a simulation study to demonstrate the effect of the laziness parameter, which controls the extent of temporal correlation, on the accuracy of community and changepoint estimation.
更多查看译文
关键词
community estimation,concentration,processes,analysis,network-valued
AI 理解论文
溯源树
样例
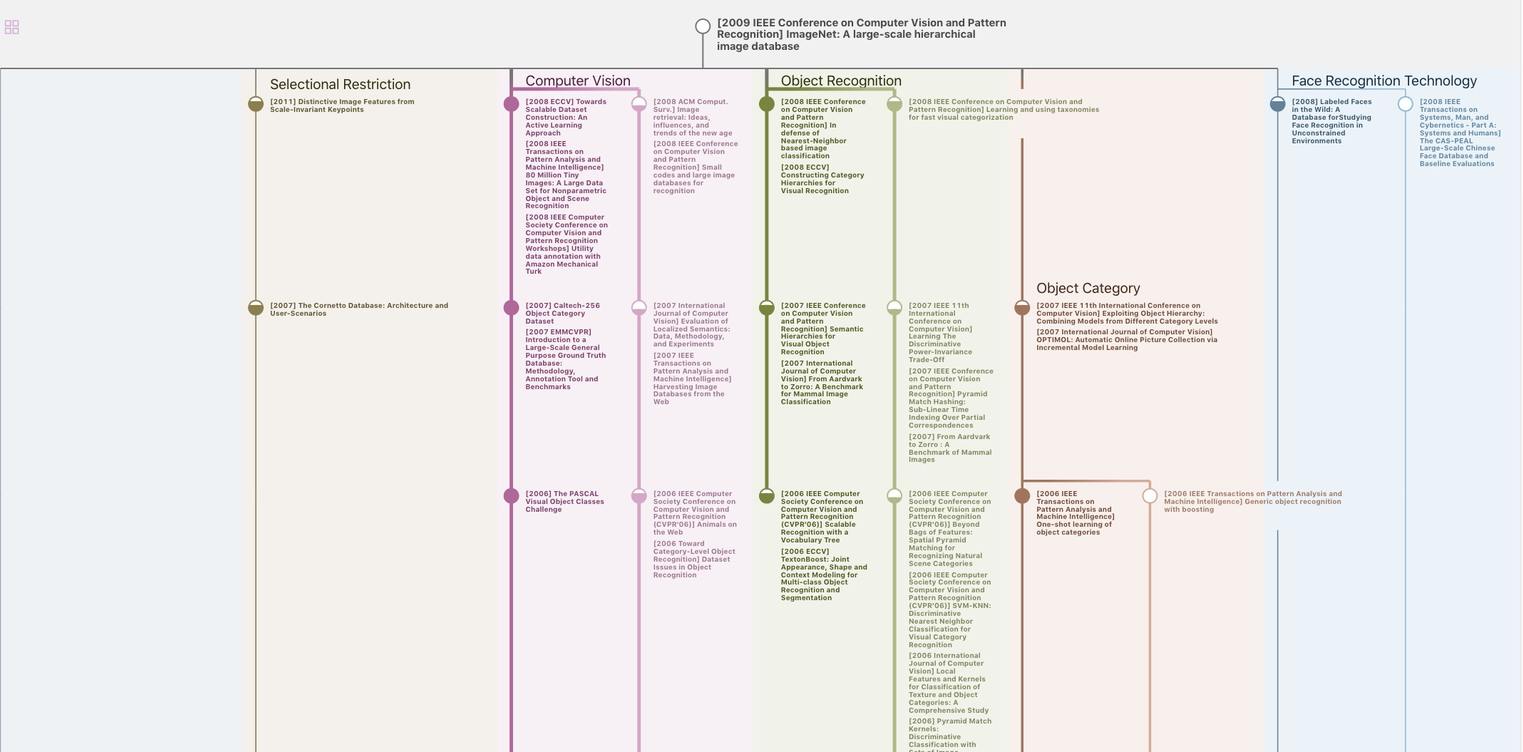
生成溯源树,研究论文发展脉络
Chat Paper
正在生成论文摘要