Deep Learning Closure Models for Large-Eddy Simulation of Flows around Bluff Bodies
arXiv (Cornell University)(2022)
摘要
A deep learning (DL) closure model for large-eddy simulation (LES) is developed and evaluated for incompressible flows around a rectangular cylinder at moderate Reynolds numbers. Near-wall flow simulation remains a central challenge in aerodynamic modeling: RANS predictions of separated flows are often inaccurate, while LES can require prohibitively small near-wall mesh sizes. The DL-LES model is trained using adjoint PDE optimization methods to match, as closely as possible, direct numerical simulation (DNS) data. It is then evaluated out-of-sample (i.e., for new aspect ratios and Reynolds numbers not included in the training data) and compared against a standard LES model (the dynamic Smagorinsky model). The DL-LES model outperforms dynamic Smagorinsky and is able to achieve accurate LES predictions on a relatively coarse mesh (downsampled from the DNS grid by a factor of four in each Cartesian direction). We study the accuracy of the DL-LES model for predicting the drag coefficient, mean flow, and Reynolds stress. A crucial challenge is that the LES quantities of interest are the steady-state flow statistics; for example, the time-averaged mean velocity $\bar{u}(x) = \displaystyle \lim_{t \rightarrow \infty} \frac{1}{t} \int_0^t u(s,x) ds$. Calculating the steady-state flow statistics therefore requires simulating the DL-LES equations over a large number of flow times through the domain; it is a non-trivial question whether an unsteady partial differential equation model whose functional form is defined by a deep neural network can remain stable and accurate on $t \in [0, \infty)$. Our results demonstrate that the DL-LES model is accurate and stable over large physical time spans, enabling the estimation of the steady-state statistics for the velocity, fluctuations, and drag coefficient of turbulent flows around bluff bodies relevant to aerodynamic applications.
更多查看译文
关键词
deep learning closure models,deep learning,flows,simulation,large-eddy
AI 理解论文
溯源树
样例
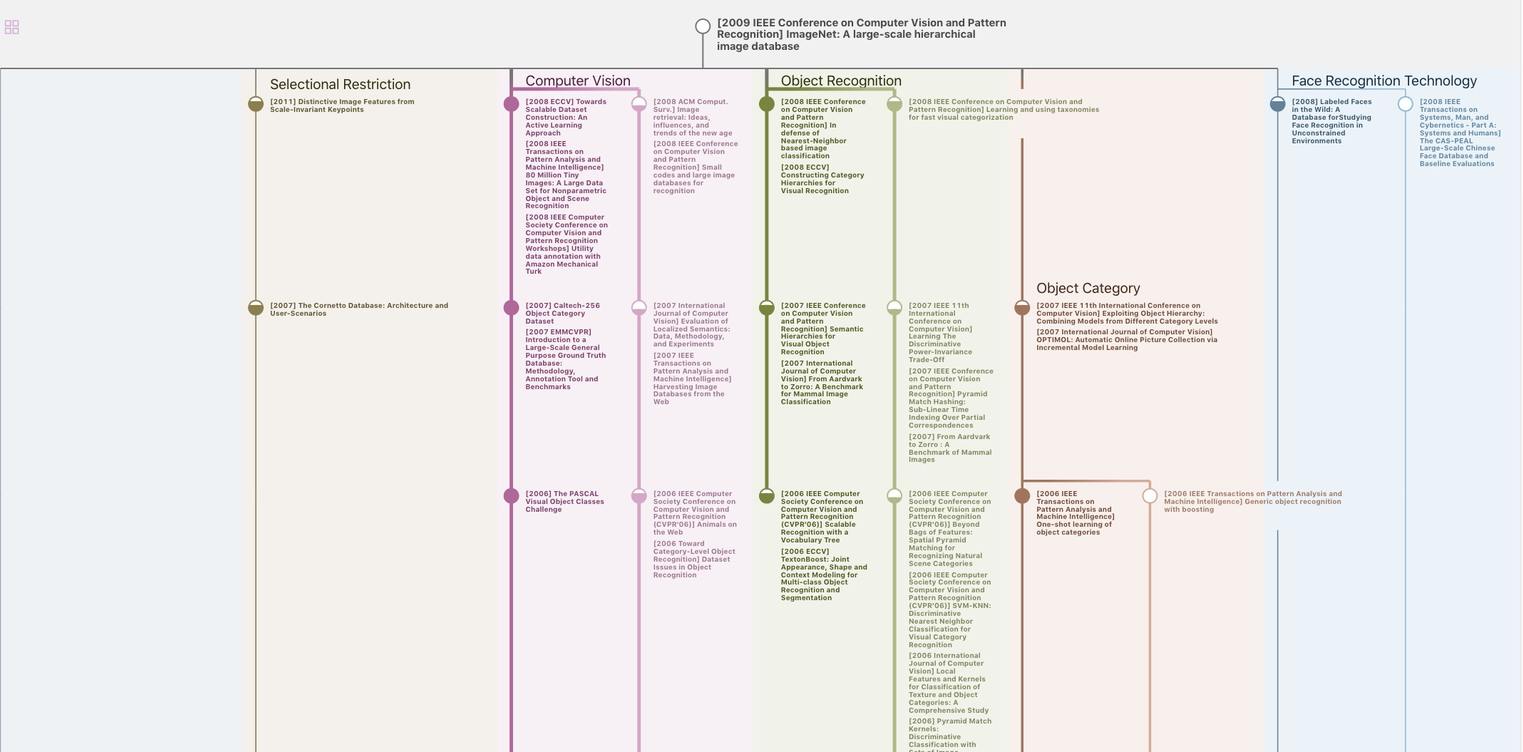
生成溯源树,研究论文发展脉络
Chat Paper
正在生成论文摘要