Geo6D: Geometric Constraints Learning for 6D Pose Estimation
arXiv (Cornell University)(2022)
摘要
Numerous 6D pose estimation methods have been proposed that employ end-to-end regression to directly estimate the target pose parameters. Since the visible features of objects are implicitly influenced by their poses, the network allows inferring the pose by analyzing the differences in features in the visible region. However, due to the unpredictable and unrestricted range of pose variations, the implicitly learned visible feature-pose constraints are insufficiently covered by the training samples, making the network vulnerable to unseen object poses. To tackle these challenges, we proposed a novel geometric constraints learning approach called Geo6D for direct regression 6D pose estimation methods. It introduces a pose transformation formula expressed in relative offset representation, which is leveraged as geometric constraints to reconstruct the input and output targets of the network. These reconstructed data enable the network to estimate the pose based on explicit geometric constraints and relative offset representation mitigates the issue of the pose distribution gap. Extensive experimental results show that when equipped with Geo6D, the direct 6D methods achieve state-of-the-art performance on multiple datasets and demonstrate significant effectiveness, even with only 10% amount of data.
更多查看译文
关键词
geo6d pose estimation,geometric constraints learning
AI 理解论文
溯源树
样例
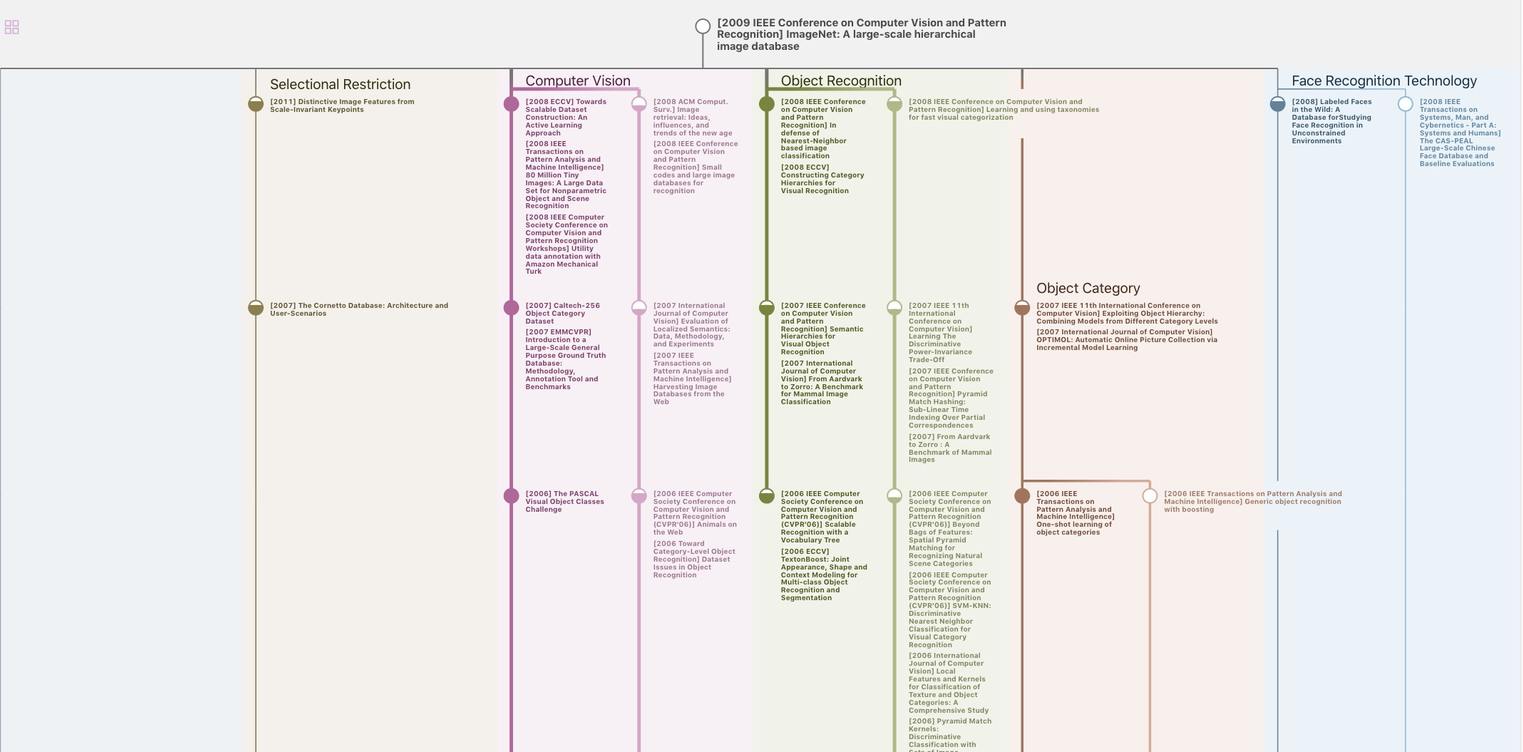
生成溯源树,研究论文发展脉络
Chat Paper
正在生成论文摘要