Slowly Changing Adversarial Bandit Algorithms are Efficient for Discounted MDPs
arXiv (Cornell University)(2022)
摘要
Reinforcement learning generalizes bandit problems with additional difficulties on longer planning horizon and unknown transition kernel. We show that, under some mild assumptions, *any* slowly changing adversarial bandit algorithm enjoys optimal regret in adversarial bandits can achieve optimal (in the dependency of $T$) expected regret in infinite-horizon discounted MDPs, without the presence of Bellman backups. The slowly changing property required by our generalization is mild, which is also marked by the online Markov decision process literature. We also examine the applicability of our reduction to a well-known adversarial bandit algorithm, EXP3.
更多查看译文
关键词
adversarial bandit algorithms
AI 理解论文
溯源树
样例
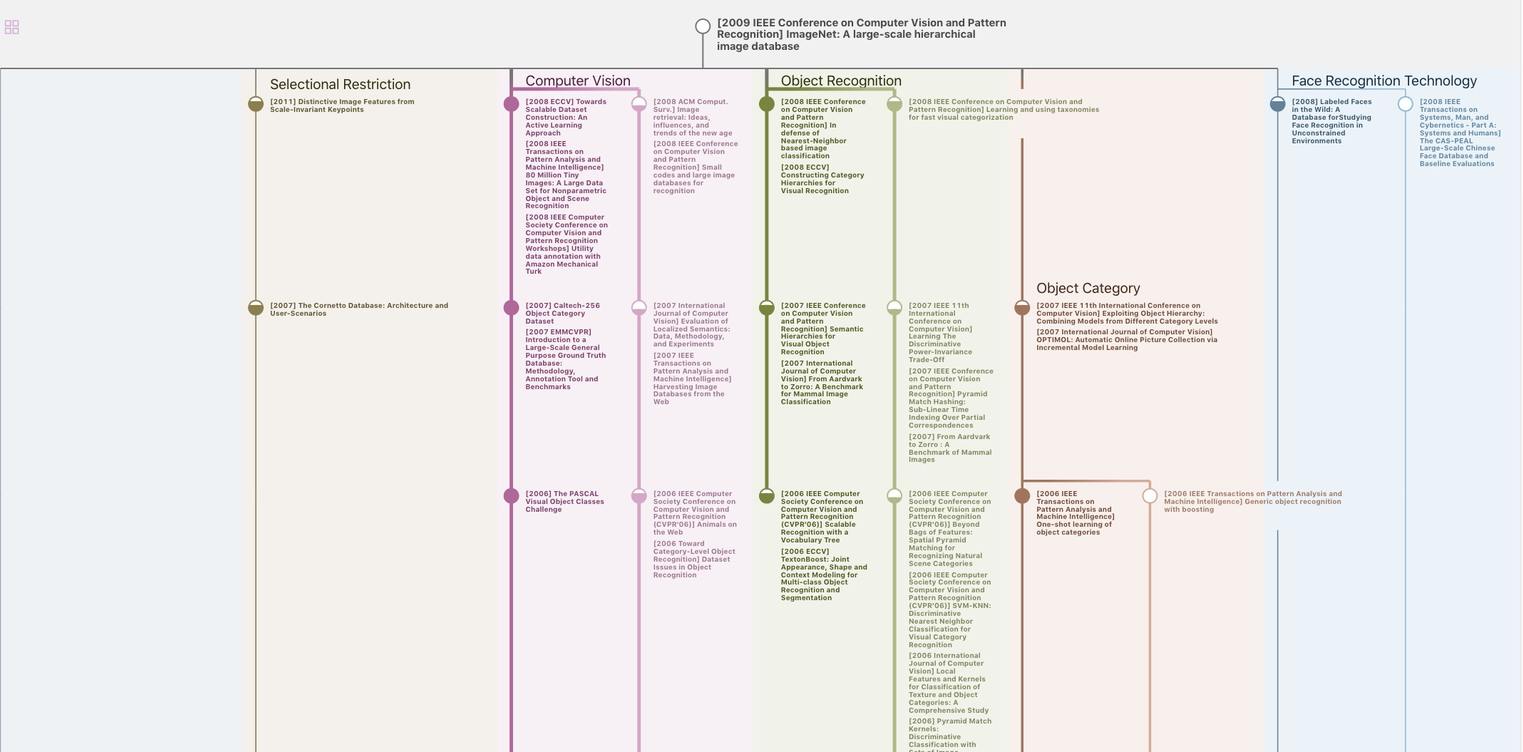
生成溯源树,研究论文发展脉络
Chat Paper
正在生成论文摘要