Detection of Word Adversarial Examples in Text Classification: Benchmark and Baseline via Robust Density Estimation
arXiv (Cornell University)(2022)
摘要
Word-level adversarial attacks have shown success in NLP models, drastically decreasing the performance of transformer-based models in recent years. As a countermeasure, adversarial defense has been explored, but relatively few efforts have been made to detect adversarial examples. However, detecting adversarial examples may be crucial for automated tasks (e.g. review sentiment analysis) that wish to amass information about a certain population and additionally be a step towards a robust defense system. To this end, we release a dataset for four popular attack methods on four datasets and four models to encourage further research in this field. Along with it, we propose a competitive baseline based on density estimation that has the highest AUC on 29 out of 30 dataset-attack-model combinations. Source code is available in https://github.com/anoymous92874838/text-adv-detection.
更多查看译文
关键词
word adversarial examples,text classification,detection
AI 理解论文
溯源树
样例
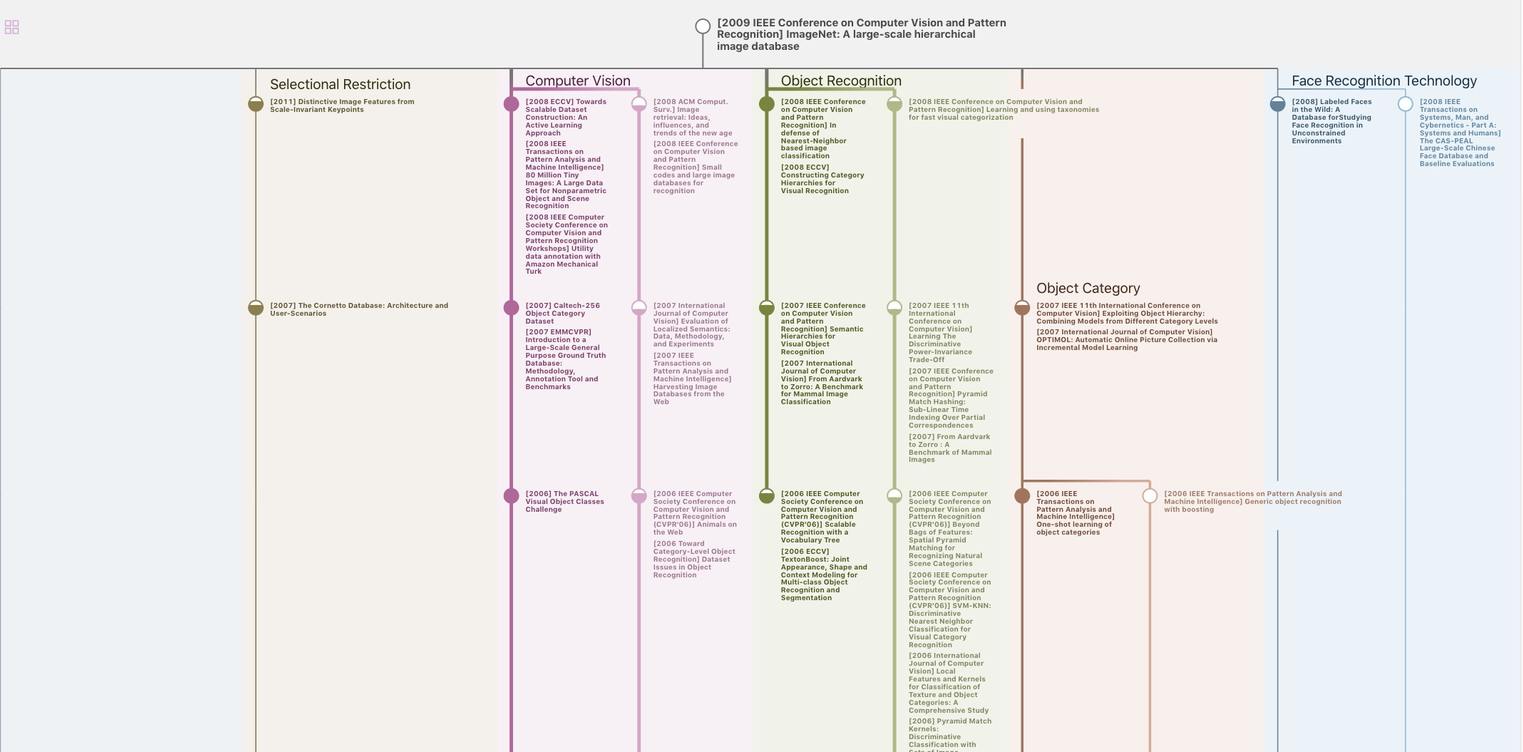
生成溯源树,研究论文发展脉络
Chat Paper
正在生成论文摘要