Understanding Rare Spurious Correlations in Neural Networks
arXiv (Cornell University)(2022)
摘要
Neural networks are known to use spurious correlations such as background information for classification. While prior work has looked at spurious correlations that are widespread in the training data, in this work, we investigate how sensitive neural networks are to rare spurious correlations, which may be harder to detect and correct, and may lead to privacy leaks. We introduce spurious patterns correlated with a fixed class to a few training examples and find that it takes only a handful of such examples for the network to learn the correlation. Furthermore, these rare spurious correlations also impact accuracy and privacy. We empirically and theoretically analyze different factors involved in rare spurious correlations and propose mitigation methods accordingly. Specifically, we observe that $\ell_2$ regularization and adding Gaussian noise to inputs can reduce the undesirable effects. Code available at https://github.com/yangarbiter/rare-spurious-correlation.
更多查看译文
关键词
rare spurious correlations,neural networks
AI 理解论文
溯源树
样例
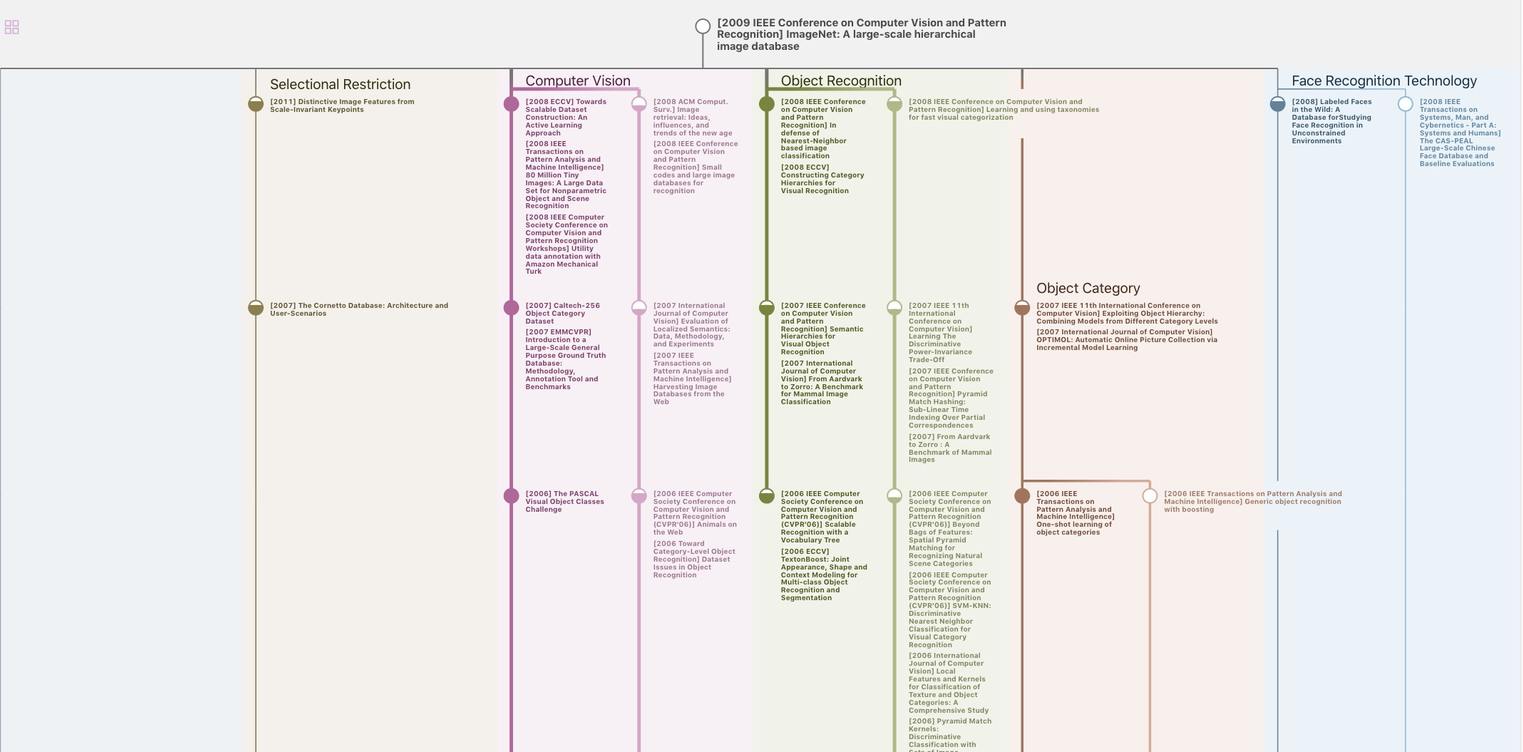
生成溯源树,研究论文发展脉络
Chat Paper
正在生成论文摘要