代理表現によるデータ駆動型トポロジーデザインの強化に関する研究
Saitekika Shinpojiumu koen ronbunshu(2022)
摘要
Data-driven topology design is a method that enables gradient-free topology optimization like evolutionary algorithms. However, the dimension of design variables that can be handled by this method is limited to about 104 due to the fact that the deep generative model is used as the driving force for the solution search. Therefore, for high-resolution problems with large numbers of design variables, it is necessary to use some method to reduce the dimensionality of the design variables handled by the deep generative model. In this study, we propose a new framework that incorporates an interpolation function-based surrogate representation into the design process and discuss its applicability to high-resolution problems. We demonstrate that the performance of solutions obtained using the proposed method can almost achieve that of the existing method under a lower computational cost by demonstrating the effectiveness of the proposed method.
更多查看译文
AI 理解论文
溯源树
样例
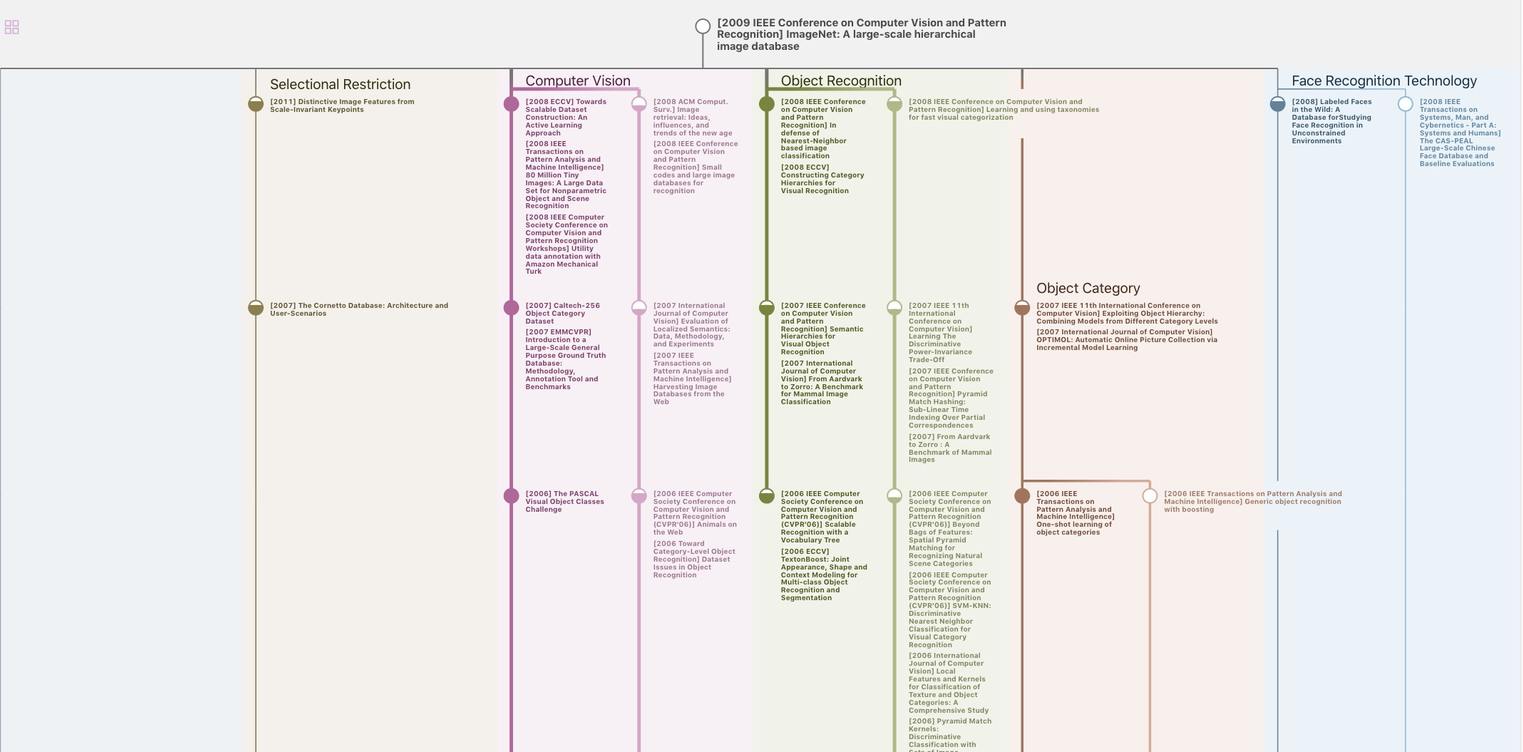
生成溯源树,研究论文发展脉络
Chat Paper
正在生成论文摘要