Deep learning-based prognosis prediction among preeclamptic pregnancies using electronic health record data
medRxiv (Cold Spring Harbor Laboratory)(2022)
摘要
Background Preeclampsia (PE) is one of the leading factors in maternal and perinatal mortality and morbidity worldwide. Delivery timing is key to balancing the risk between severe maternal and neonatal morbidities in pregnancies complicated by PE. Method In this study, we constructed and validated first-of-their-kind deep learning models that can forecast the time to delivery among patients with PE using electronic health records (EHR) data. The discovery cohort consisted of 1,533 preeclamptic pregnancies, including 374 cases of early-onset preeclampsia (EOPE), that were delivered at University of Michigan Health System (UM) between 2015 and 2021. The validation cohort contained 2,172 preeclamptic pregnancies (including 547 EOPE) from University of Florida Health System (UF) in the same period. Using Cox-nnet, a neural network-based prognosis prediction algorithm, we built baseline models of all PE patients and of the subset of EOPE patients, using 47 features on demographics, medical history, comorbidities, the severity of PE, and gestational age of initial PE diagnosis. We also built full models using 62 features, combining those in baseline models and additional features on lab tests and vital signs, on the same PE patients and EOPE subset. The models were re-trained and re-validated using reduced sets of the most important features, to improve their interpretability and clinical applicability. Findings The 7-feature baseline models on all PE patients reached C-indices of 0.73, 0.74 and 0.73 on UM training, hold-out testing and UF validation dataset respectively, whereas the 12-feature full model had improved C-indices of 0.78, 0.79 and 0.74 on the same datasets. For the EOPE cases, the 6-feature baseline model achieved C-indices of 0.67, 0.68 and 0.63 on the training, hold-out testing and UF validation dataset respectively, while its 13-feature full model counterpart reached C-indices of 0.74, 0.76 and 0.67 in the same datasets. Besides confirming the prognostic importance of gestational age at the time of diagnosis and of sPE status, all four models identified parity and PE in prior pregnancies as important features, which are not in the current guidelines for PE delivery timing. Laboratory results and vital signs such as platelet count, the standard deviation of respiratory rate within a 5-day observation window, and mean diastolic blood pressure are critical to increase the accuracy of predicting time to delivery, in addition to testing aspartate aminotransferase and creatinine levels. For EOPE time to delivery prediction, comorbidities such as pulmonary circulation disorders and coagulopathy as defined in Elixhauser Comorbidity Index are important to consider. Interpretation We set up a user-friendly web interface to allow personalized PE time to delivery prediction. The app is available at http://garmiregroup.org/PE-prognosis-predictor/app These actionable models may help providers to plan antepartum care in these pregnancies and significantly improve the management/clinical outcomes of pregnancies affected by PE. Funding This study is funded by the National Institutes of Health
更多查看译文
关键词
preeclamptic pregnancies,prognosis prediction,learning-based
AI 理解论文
溯源树
样例
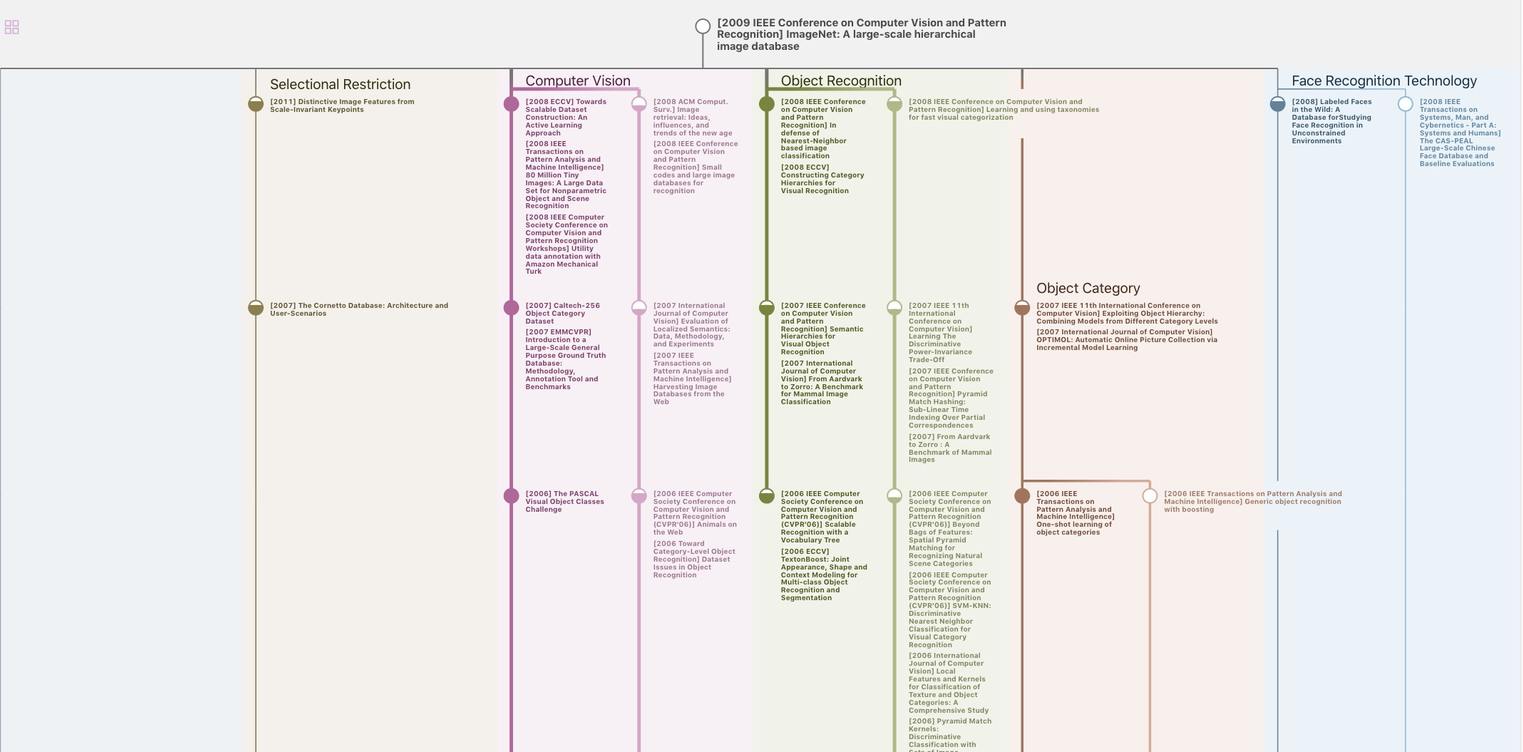
生成溯源树,研究论文发展脉络
Chat Paper
正在生成论文摘要