Abstract 9903: Artificial Intelligence Driven Wearable 2-minute Seismocardiography Test for Detection of Aortic Valve Stenosis Severity
Circulation(2022)
摘要
Introduction: Standard diagnosis of aortic valve stenosis (AS) requires clinical imaging by echocardiography or cardiac MRI. The purpose of this study was to test the performance of easy to use, artificial intelligent (AI) driven seismocardiogram (SCG) chest acceleration recordings for point of care screening and identification of AS. Hypothesis: SCG measurements can be used by a low dimensional convolutional neural network (CNN) to accurately predict presence or absence of aortic valve stenosis (AS) and severity of dysfunction. Methods: This IRB approved study included 45 controls (age 46±17y, 20 F, no known cardiovascular disease) and 30 aortic valve disease (AVD) patients (age 30±20y, 7 F, 21 bicuspid). A wearable SCG device (integrated ECG/accelerometer microelectronics) was placed for 2min chest acceleration recordings on the same day as standard of care echocardiography or cardiac MRI, which was used as ground truth data to classify valve function (normal: AS-, mild AS: AS+, moderate/severe AS: AS++). A deep learning CNN (2х2 kernel, softmax/cross entropy classification) was configured to predict/detect degree of AS from spectrally decomposed SCG measurements. Randomized 50% split of subjects was used for CNN training/testing. Performance detecting AS was evaluated for classification accuracy. Results: AI driven SCG detection of valve function abnormality had high sensitivity/specificity (90%, 67%) with a net area under curve (AUC) 0.86. Correct classification of the degree of AS was achieved in 76% of subjects. Normal (AS-) vs abnormal function (AS+ or AS++) was accurately identified by AI driven SCG for 86% of subjects. Conclusions: This feasibility study demonstrates that the combination of easily measured 2 minute SCG with a deep processing pipeline could successfully detect the presence and severity of AS, supporting clinical promise for potential use as a low cost, front line screening tool to identify patients with aortic valve dysfunction.
更多查看译文
关键词
artificial intelligence
AI 理解论文
溯源树
样例
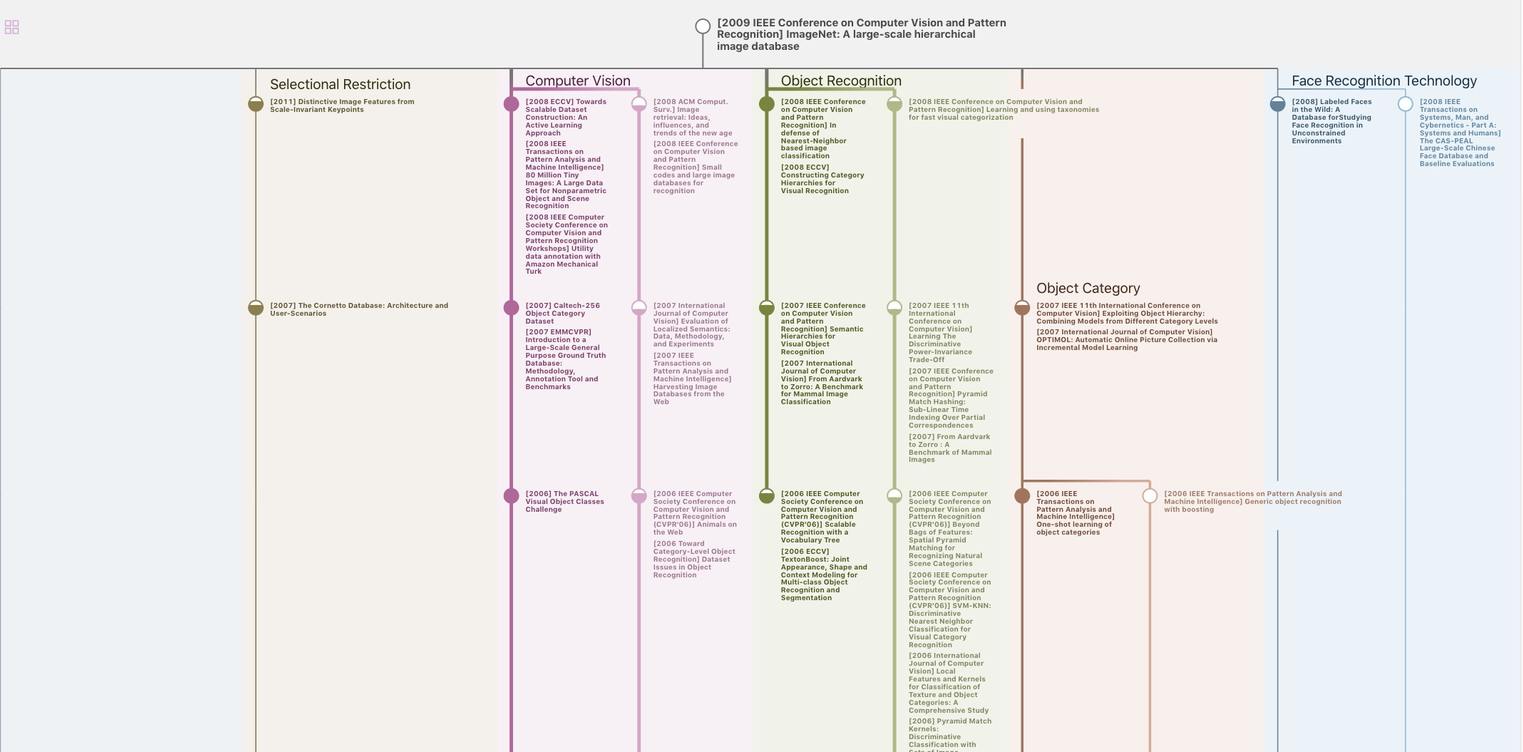
生成溯源树,研究论文发展脉络
Chat Paper
正在生成论文摘要