Abstract 12739: Evaluation of Quantitative Decision-Making for Rhythm-Management of Atrial Fibrillation Using Tabular Q-Learning
Circulation(2022)
摘要
Introduction: Rhythm-management is among the most complex decisions for patients diagnosed with atrial fibrillation (AF). While clinical trials have identified subsets of patients who might have a benefit from a given rhythm-management strategy, for individual patients it is not always clear which strategy is expected to have the greatest mortality benefit or durability. Hypothesis: Combined application of unsupervised machine learning and reinforcement learning can assist with optimizing individualized treatment strategies for patients with AF. Methods: In this investigation of over 50K subjects with a new AF diagnosis between the dates of October 11, 2010 and October 26, 2020, we examined the application of a type of artificial intelligence called tabular Q-learning to identify the optimal initial rhythm-management strategy, based on a composite outcome of mortality, change in treatment, and sustainability of the given treatment, termed the reward function. Results: We first applied an unsupervised learning algorithm using a variational autoencoder with K-means clustering to cluster AF patients into 8 distinct phenotypes, which matched what has been observed in clinical practice. We then fit a Q-learning algorithm to predict the best outcome for each cluster, in which we found that while a rate-control strategy was most frequently selected by treating providers, the outcome was superior for rhythm-control strategies across all cluster groups, with those subjects in whom provider-selected treatment matched what the Q table recommended having fewer total deaths [4 (8.5%) vs 473 (22.4%), Odds ratio = 0.32, P = 0.02] and a greater reward [-0.0115±0.26 vs -0.392±2.06, P = 4.8x10 -6 ]. We then demonstrated application of dynamic learning by updating the Q table prospectively using batch gradient descent, in which the optimal strategy in some clusters changed from cardioversion to ablation, although the reward was greatest when the largest amount of data was used for model fitting. Conclusions: We found that tabular Q-learning provides a dynamic and interpretable approach to apply artificial intelligence to clinical decision making for AF. Further work is needed to examine application of Q-learning prospectively in clinical patients.
更多查看译文
关键词
atrial fibrillation,decision-making,rhythm-management,q-learning
AI 理解论文
溯源树
样例
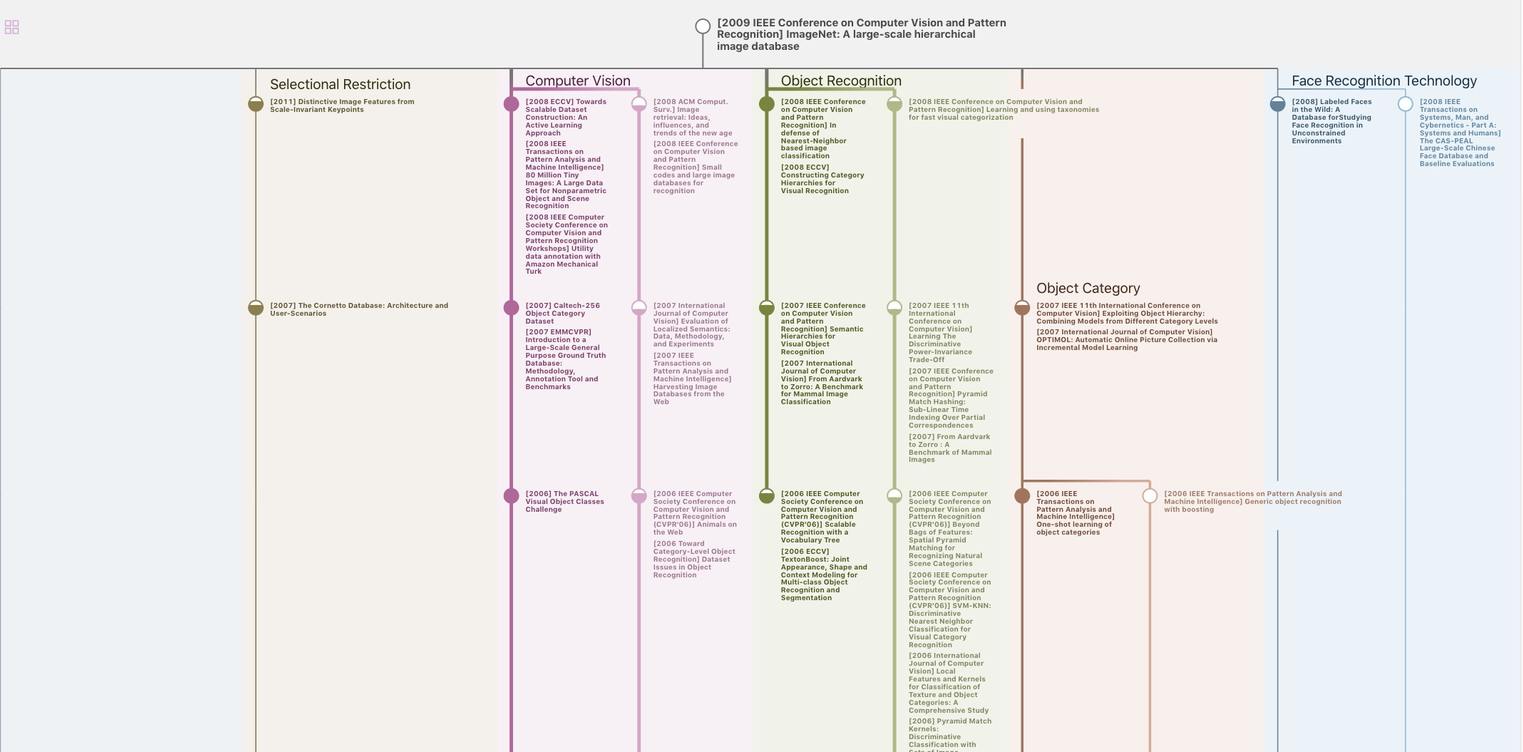
生成溯源树,研究论文发展脉络
Chat Paper
正在生成论文摘要