Visualizing hierarchies in scRNA-seq data using a density tree-biased autoencoder
HAL (Le Centre pour la Communication Scientifique Directe)(2022)
摘要
Single cell RNA sequencing (scRNA-seq) data makes studying the development of cells possible at unparalleled resolution. Given that many cellular differentiation pro- cesses are hierarchical, their scRNA-seq data is expected to be approximately tree-shaped in gene expression space. Inference and representation of this tree-structure in two dimensions is highly desirable for biological interpretation and exploratory analysis. Our two contributions are an approach for identifying a meaningful tree structure from high-dimensional scRNA-seq data, and a visualization method respecting the tree-structure. We extract the tree structure by means of a density based minimum spanning tree on a vector quantization of the data and show that it captures biological information well. We then introduce DTAE, a tree-biased autoencoder that emphasizes the tree structure of the data in low dimensional space. We com- pare to other dimension reduction methods and demonstrate the success of our method experimentally. Our implementation relying on PyTorch and Higra is available at github.com/hci-unihd/DTAE.
更多查看译文
关键词
hierarchies,scrna-seq,tree-biased
AI 理解论文
溯源树
样例
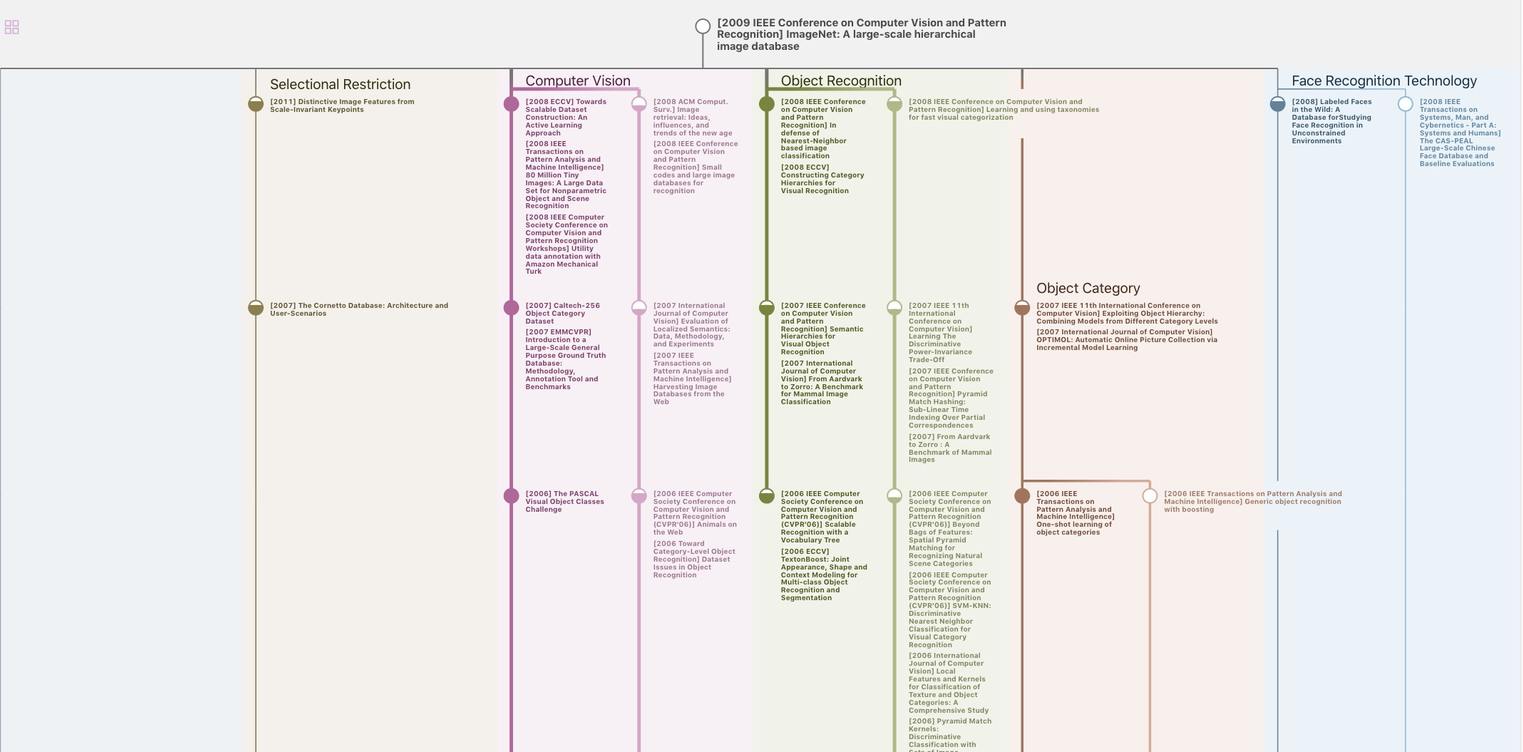
生成溯源树,研究论文发展脉络
Chat Paper
正在生成论文摘要