Learning from Students: Online Contrastive Distillation Network for General Continual Learning
Proceedings of the Thirty-First International Joint Conference on Artificial Intelligence(2022)
摘要
The goal of General Continual Learning (GCL) is to preserve learned knowledge and learn new knowledge with constant memory from an infinite data stream where task boundaries are blurry. Distilling the model's response of reserved samples between the old and the new models is an effective way to achieve promise performance on GCL. However, it accumulates the inherent old model's response bias and is not robust to model changes. To this end, we propose an Online Contrastive Distillation Network (OCD-Net) to tackle these problems, which explores the merit of the student model in each time step to guide the training process of the student model. Concretely, the teacher model is devised to help the student model to consolidate the learned knowledge, which is trained online via integrating the model weights of the student model to accumulate the new knowledge. Moreover, our OCD-Net incorporates both relation and adaptive response to help the student model alleviate the catastrophic forgetting, which is also beneficial for the teacher model preserves the learned knowledge. Extensive experiments on six benchmark datasets demonstrate that our proposed OCD-Net significantly outperforms state-of-the-art approaches in 3.26%~8.71% with various buffer sizes. Our code is available at https://github.com/lijincm/OCD-Net.
更多查看译文
关键词
online contrastive distillation network,continual learning,students
AI 理解论文
溯源树
样例
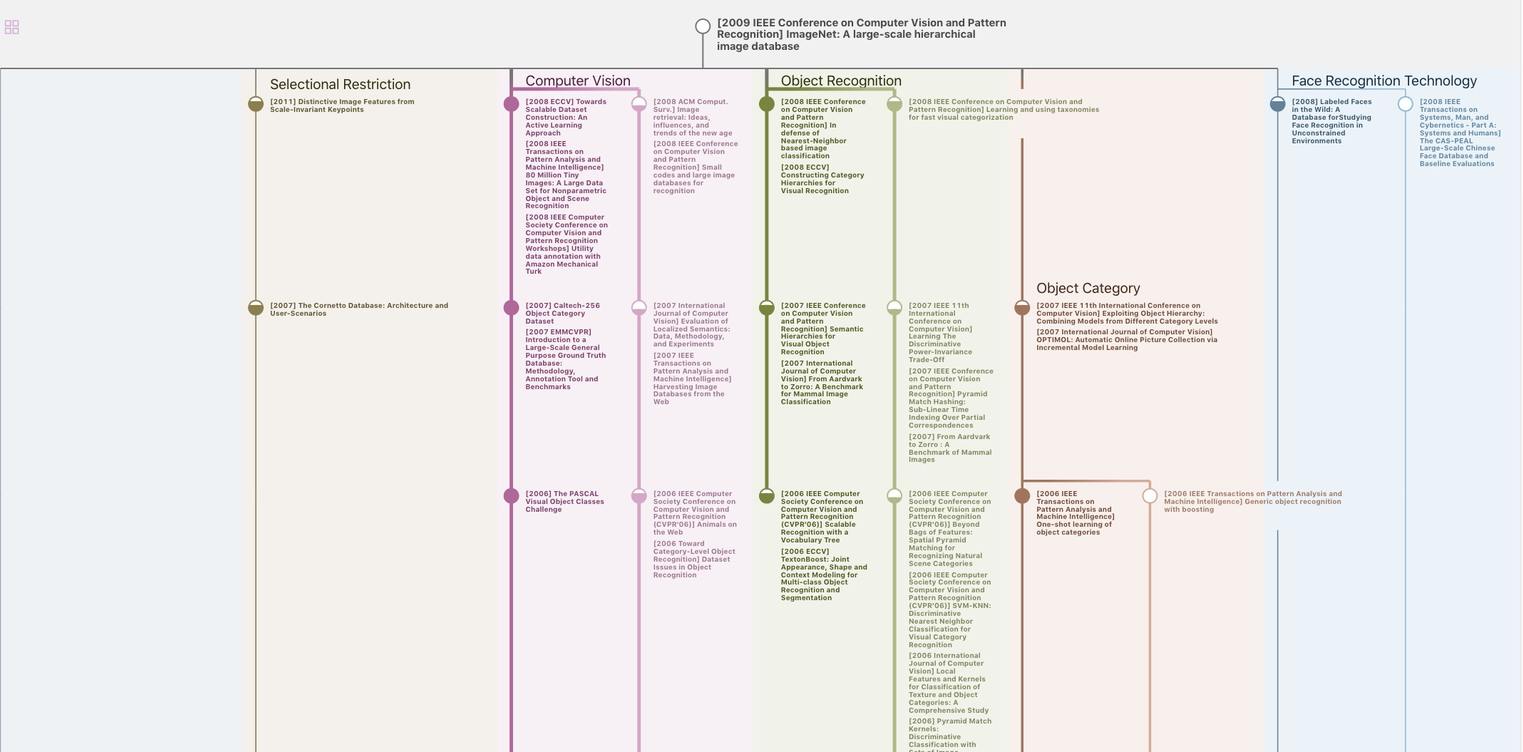
生成溯源树,研究论文发展脉络
Chat Paper
正在生成论文摘要