Distributionally Preference Robust Optimization in Multi-Attribute Decision Making
arXiv (Cornell University)(2022)
摘要
Utility preference robust optimization (PRO) has recently been proposed to deal with optimal decision making problems where the decision maker's (DM) preference over gains and losses is ambiguous. In this paper, we take a step further to investigate the case that the DM's preference is not only ambiguous but also potentially inconsistent or even displaying some kind of randomness. We propose a distributionally preference robust optimization (DPRO) approach where the DM's preference is represented by a random utility function and the ambiguity is described by a set of probability distributions of the random utility. An obvious advantage of the new DPRO model is that it no longer concerns the DM's preference inconsistency. In the case when the random utility functions are of piecewise linear structure, we propose two statistical methods for constructing the ambiguity set: an ellipsoidal method and a bootstrap method both of which are fundamentally based on the idea of confidence region with the sample mean of the random parameters, and demonstrate how the resulting DPRO can be solved by a cutting surface algorithm and an MISOCP respectively. We also show how the DPRO models with general random utility functions may be approximated by those with piecewise linear random utility functions. Finally, we apply the proposed DPRO model in car manufacturing and facility location planning and show how the random samples may be extracted by multinomial logit method and conjoint analysis/machine learning. The paper is the first attempt to use distributionally robust optimization methods for PRO.
更多查看译文
关键词
preference,optimization,decision making,multi-attribute
AI 理解论文
溯源树
样例
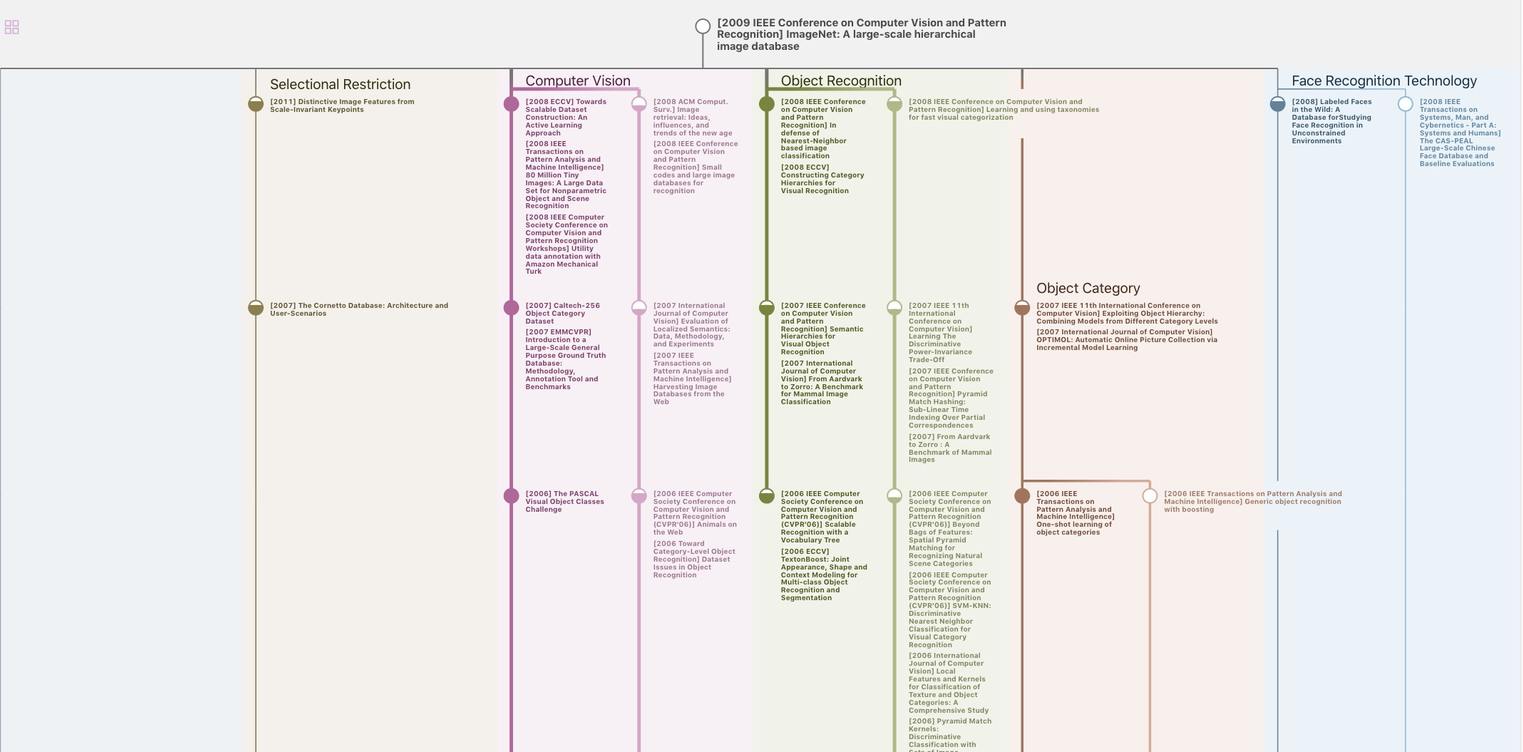
生成溯源树,研究论文发展脉络
Chat Paper
正在生成论文摘要