Gaussian Process Surrogate Models for Neural Networks
arXiv (Cornell University)(2022)
摘要
Not being able to understand and predict the behavior of deep learning systems makes it hard to decide what architecture and algorithm to use for a given problem. In science and engineering, modeling is a methodology used to understand complex systems whose internal processes are opaque. Modeling replaces a complex system with a simpler, more interpretable surrogate. Drawing inspiration from this, we construct a class of surrogate models for neural networks using Gaussian processes. Rather than deriving kernels for infinite neural networks, we learn kernels empirically from the naturalistic behavior of finite neural networks. We demonstrate our approach captures existing phenomena related to the spectral bias of neural networks, and then show that our surrogate models can be used to solve practical problems such as identifying which points most influence the behavior of specific neural networks and predicting which architectures and algorithms will generalize well for specific datasets.
更多查看译文
关键词
neural networks,gaussian,models,process
AI 理解论文
溯源树
样例
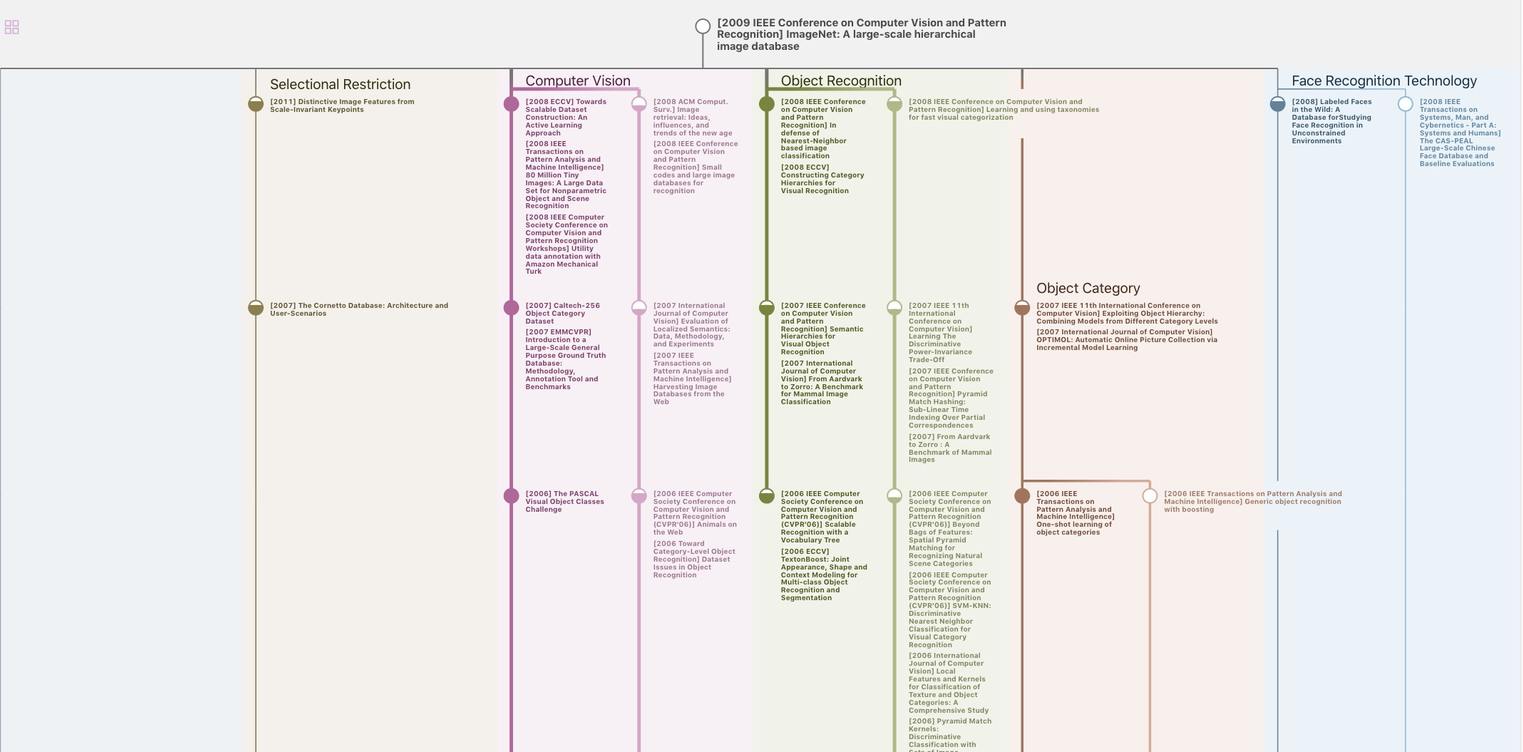
生成溯源树,研究论文发展脉络
Chat Paper
正在生成论文摘要