End-to-End Training for Back-Translation with Categorical Reparameterization Trick
arXiv (Cornell University)(2022)
摘要
Back-translation is an effective semi-supervised learning framework in neural machine translation (NMT). A pre-trained NMT model translates monolingual sentences and makes synthetic bilingual sentence pairs for the training of the other NMT model, and vice versa. Understanding the two NMT models as inference and generation models, respectively, previous works applied the training framework of variational auto-encoder (VAE). However, the discrete property of translated sentences prevents gradient information from flowing between the two NMT models. In this paper, we propose a categorical reparameterization trick that makes NMT models generate differentiable sentences so that the VAE's training framework can work in the end-to-end fashion. Our experiments demonstrate that our method effectively trains the NMT models and achieves better BLEU scores than the previous baseline on the datasets of the WMT translation task.
更多查看译文
关键词
categorical
AI 理解论文
溯源树
样例
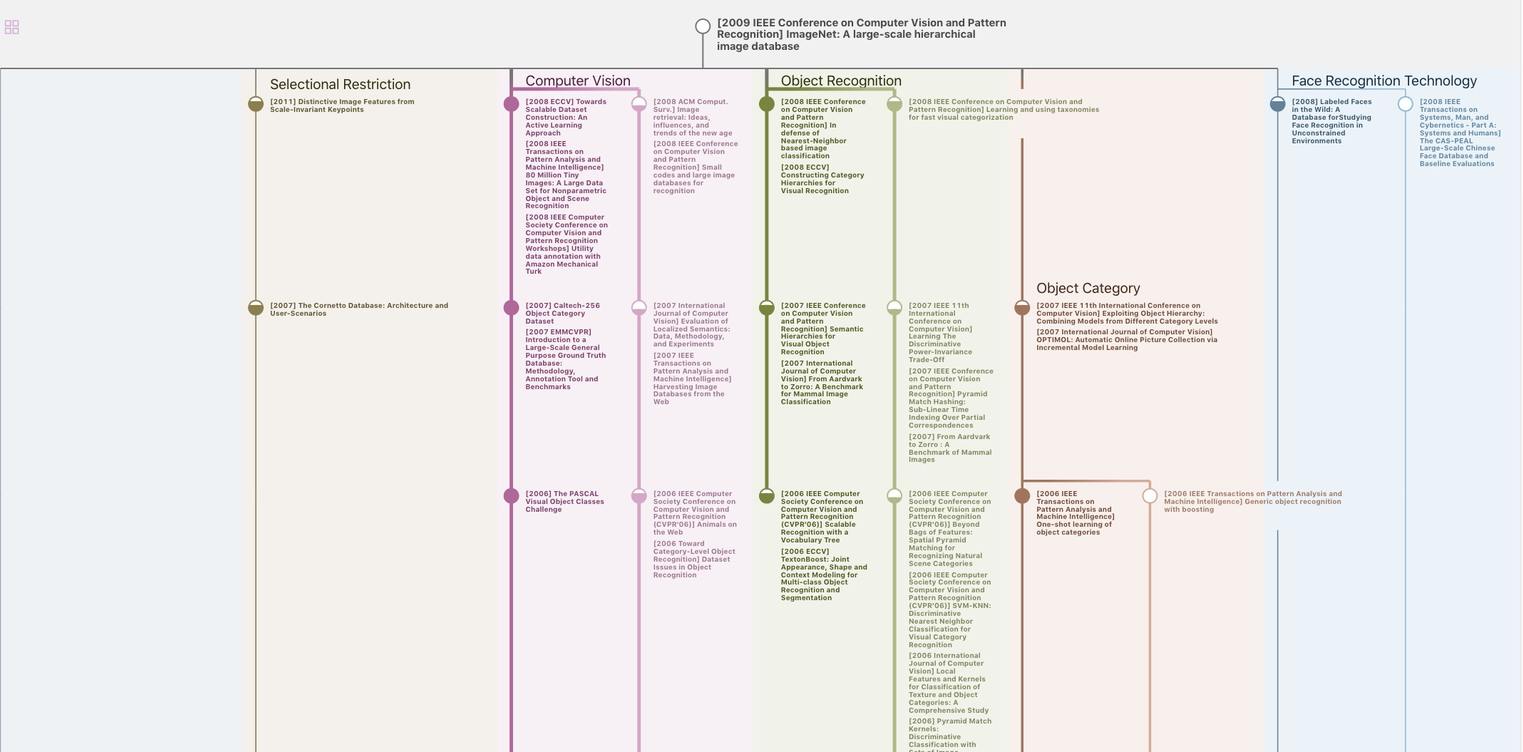
生成溯源树,研究论文发展脉络
Chat Paper
正在生成论文摘要