Mapping pareto fronts for efficient multi-objective materials discovery
Authorea (Authorea)(2023)
摘要
With advancements in automation and high-throughput techniques, we can tackle more complex multi-objective materials discovery problems requiring a higher evaluation budget. Given that experimentation is greatly limited by evaluation budget, maximizing sample efficiency of optimization becomes crucial. We discuss the limitations of using hypervolume as a performance indicator and propose new metrics relevant to materials experimentation: such as the ability to perform well for complex high-dimensional problems, minimizing wastage of evaluations, consistency/robustness of optimization, and ability to scale well to high throughputs. With these metrics, we perform an empirical study of two conceptually different and state-of-the-art algorithms (Bayesian and Evolutionary) on synthetic and real-world datasets. We discuss the merits of both approaches with respect to exploration and exploitation, where fully resolving the Pareto Front provides more knowledge of the best material.
更多查看译文
关键词
mapping pareto fronts,discovery,materials,multi-objective
AI 理解论文
溯源树
样例
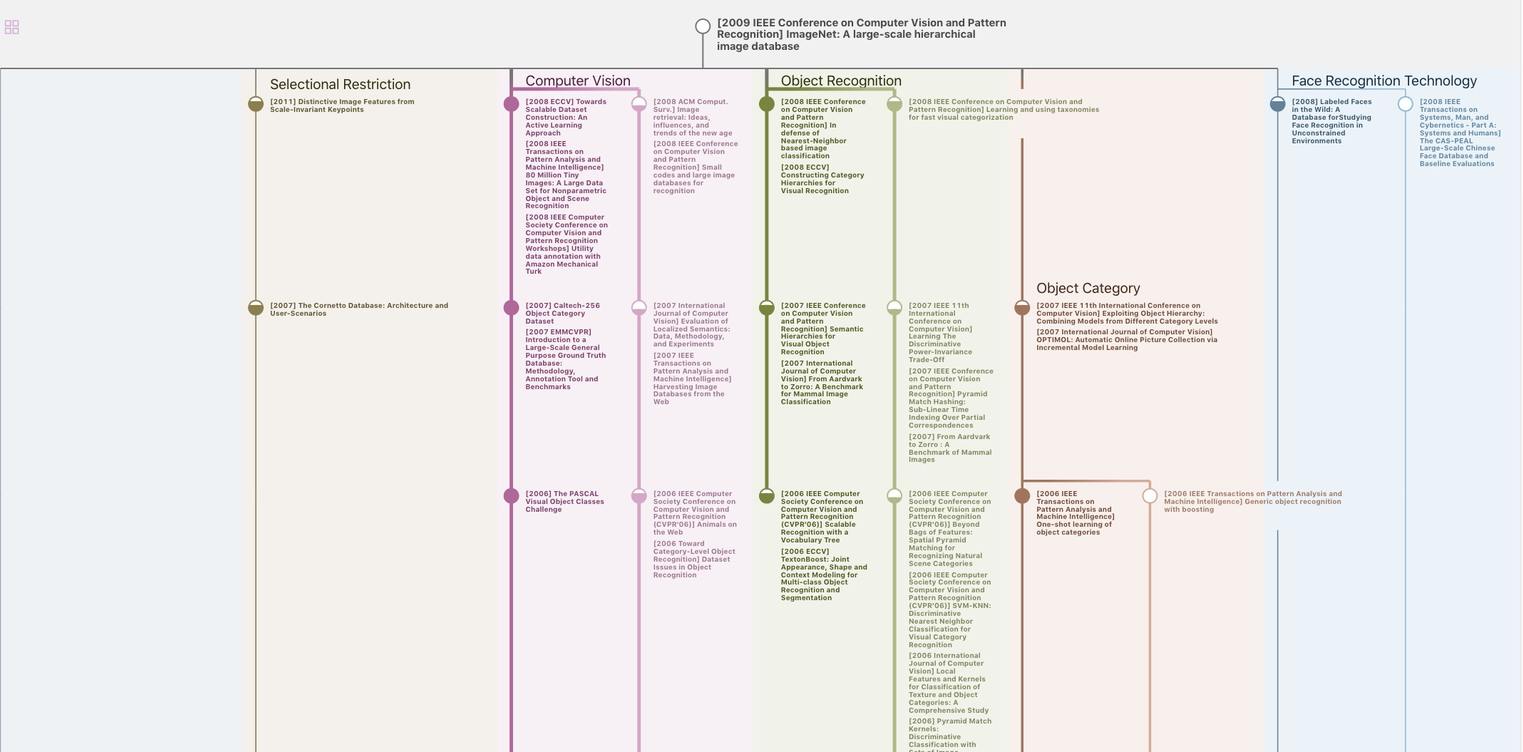
生成溯源树,研究论文发展脉络
Chat Paper
正在生成论文摘要