Unleashing the Power of Compiler Intermediate Representation to Enhance Neural Program Embeddings
arXiv (Cornell University)(2022)
摘要
Neural program embeddings have demonstrated considerable promise in a range of program analysis tasks, including clone identification, program repair, code completion, and program synthesis. However, most existing methods generate neural program embeddings directly from the program source codes, by learning from features such as tokens, abstract syntax trees, and control flow graphs. This paper takes a fresh look at how to improve program embeddings by leveraging compiler intermediate representation (IR). We first demonstrate simple yet highly effective methods for enhancing embedding quality by training embedding models alongside source code and LLVM IR generated by default optimization levels (e.g., -O2). We then introduce IRGen, a framework based on genetic algorithms (GA), to identify (near-)optimal sequences of optimization flags that can significantly improve embedding quality.
更多查看译文
关键词
compiler intermediate representation,program
AI 理解论文
溯源树
样例
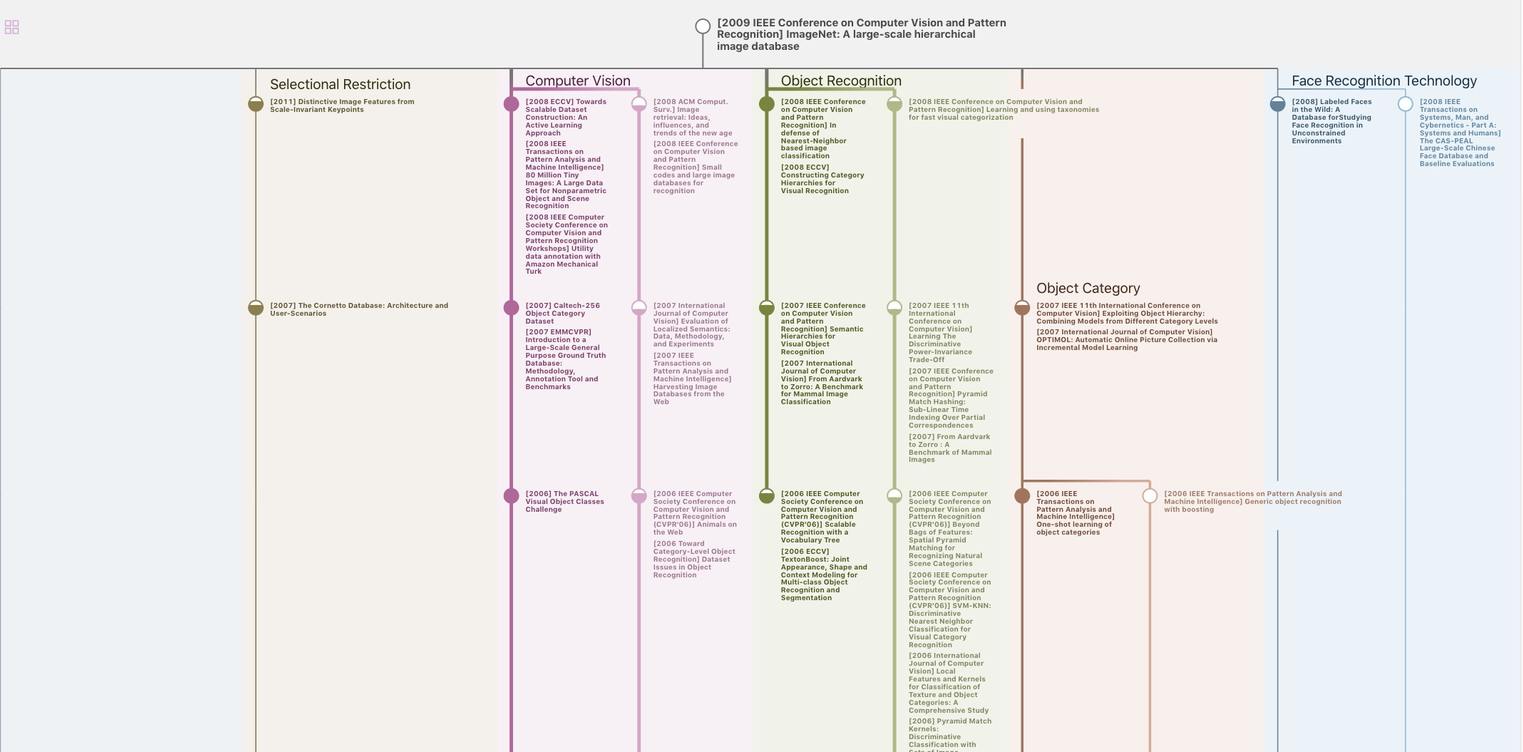
生成溯源树,研究论文发展脉络
Chat Paper
正在生成论文摘要