ETAD: Training Action Detection End to End on a Laptop
arXiv (Cornell University)(2022)
摘要
Temporal action detection (TAD) with end-to-end training often suffers from the pain of huge demand for computing resources due to long video duration. In this work, we propose an efficient temporal action detector (ETAD) that can train directly from video frames with extremely low GPU memory consumption. Our main idea is to minimize and balance the heavy computation among features and gradients in each training iteration. We propose to sequentially forward the snippet frame through the video encoder, and backward only a small necessary portion of gradients to update the encoder. To further alleviate the computational redundancy in training, we propose to dynamically sample only a small subset of proposals during training. Moreover, various sampling strategies and ratios are studied for both the encoder and detector. ETAD achieves state-of-the-art performance on TAD benchmarks with remarkable efficiency. On ActivityNet-1.3, training ETAD in 18 hours can reach 38.25% average mAP with only 1.3 GB memory consumption per video under end-to-end training. Our code will be publicly released.
更多查看译文
关键词
training action detection end,etad
AI 理解论文
溯源树
样例
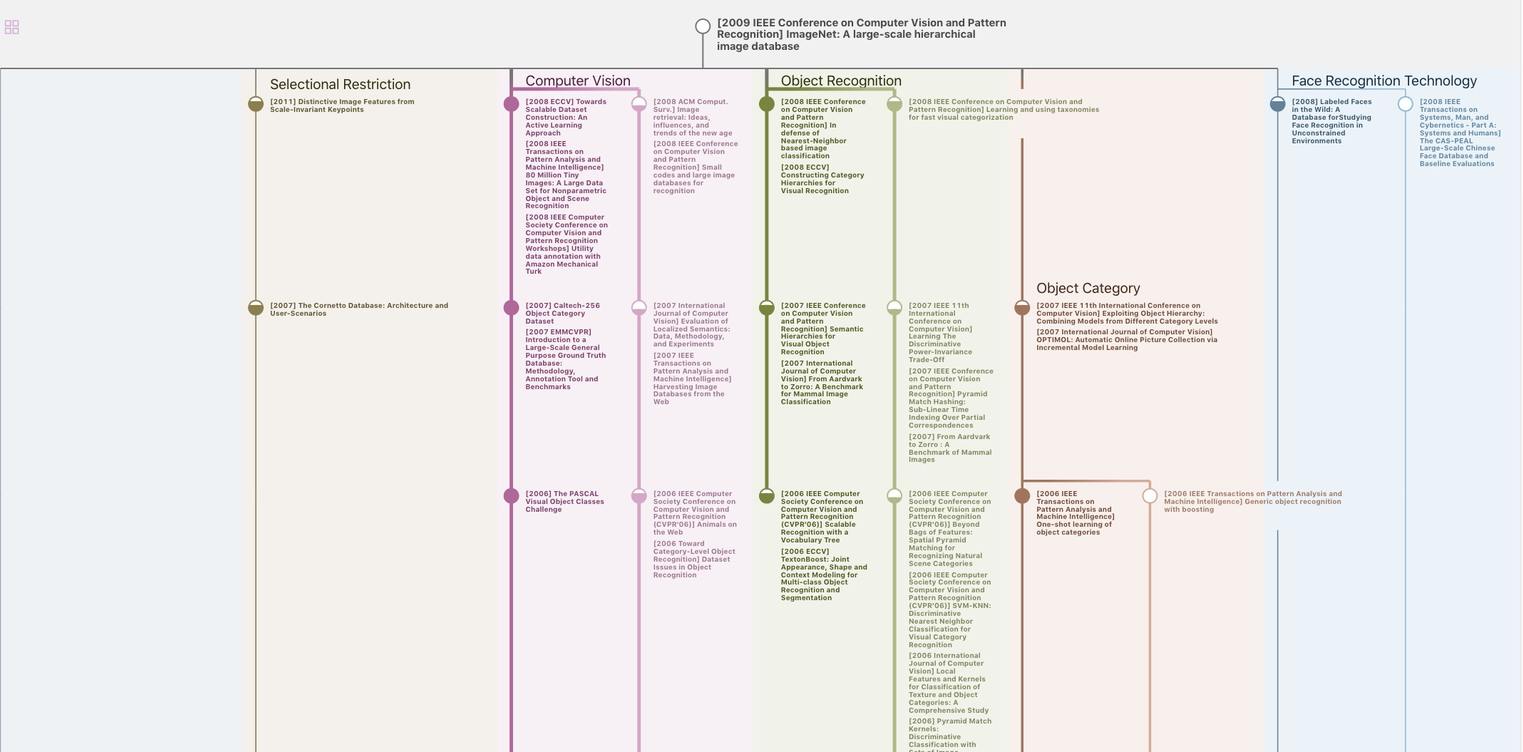
生成溯源树,研究论文发展脉络
Chat Paper
正在生成论文摘要