Random Utterance Concatenation Based Data Augmentation for Improving Short-video Speech Recognition
arXiv (Cornell University)(2022)
摘要
One of limitations in end-to-end automatic speech recognition (ASR) framework is its performance would be compromised if train-test utterance lengths are mismatched. In this paper, we propose an on-the-fly random utterance concatenation (RUC) based data augmentation method to alleviate train-test utterance length mismatch issue for short-video ASR task. Specifically, we are motivated by observations that our human-transcribed training utterances tend to be much shorter for short-video spontaneous speech (~3 seconds on average), while our test utterance generated from voice activity detection front-end is much longer (~10 seconds on average). Such a mismatch can lead to suboptimal performance. Empirically, it's observed the proposed RUC method significantly improves long utterance recognition without performance drop on short one. Overall, it achieves 5.72% word error rate reduction on average for 15 languages and improved robustness to various utterance length.
更多查看译文
关键词
speech,concatenation,short-video
AI 理解论文
溯源树
样例
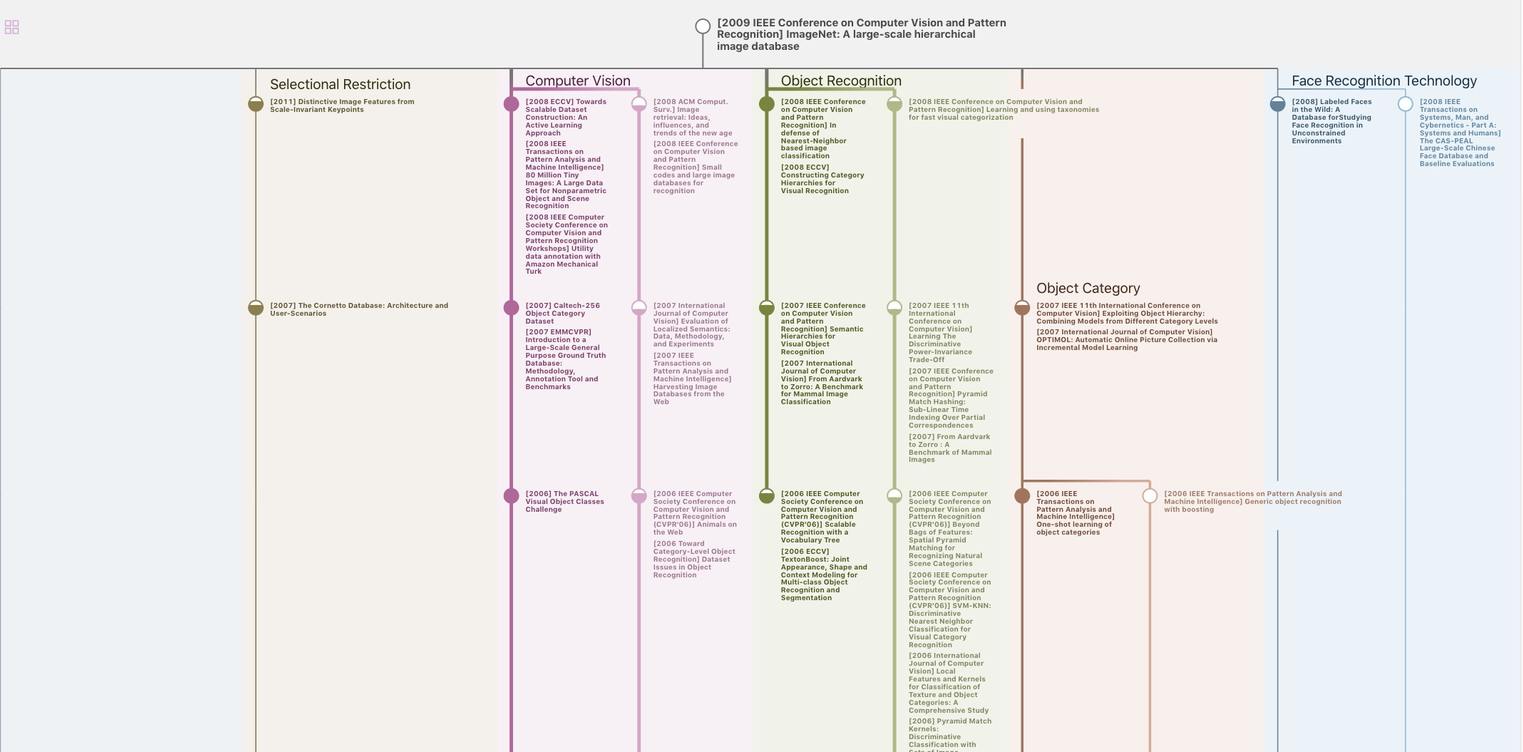
生成溯源树,研究论文发展脉络
Chat Paper
正在生成论文摘要