Distributed Prediction-Correction Algorithms for Time-Varying Nash Equilibrium Tracking
arXiv (Cornell University)(2022)
摘要
This paper focuses on a time-varying Nash equilibrium trajectory tracking problem, that is applicable to a wide range of non-cooperative game applications arising in dynamic environments. To solve this problem, we propose a distributed prediction correction algorithm, termed DPCA, in which each player predicts future strategies based on previous observations and then exploits predictions to effectively track the NE trajectory by using one or multiple distributed gradient descent steps across a network. We rigorously demonstrate that the tracking sequence produced by the proposed algorithm is able to track the time-varying NE with a bounded error. We also show that the tracking error can be arbitrarily close to zero when the sampling period is small enough. Furthermore, we achieve linear convergence for the time-invariant Nash equilibrium seeking problem as a special case of our results. Finally, a numerical simulation of a multi-robot surveillance scenario verifies the tracking performance and the prediction necessary for the proposed algorithm.
更多查看译文
关键词
nash
AI 理解论文
溯源树
样例
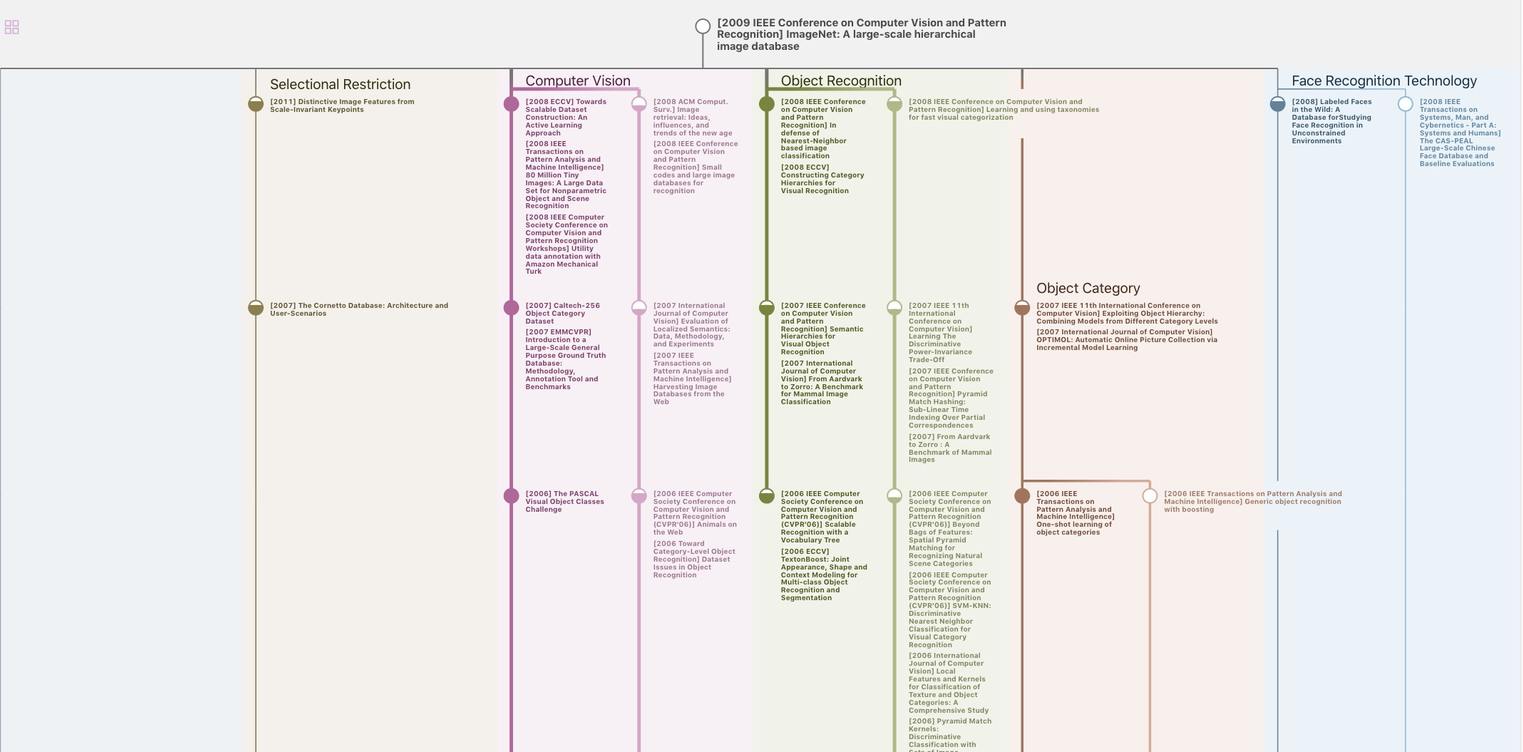
生成溯源树,研究论文发展脉络
Chat Paper
正在生成论文摘要