AI/ML for Network Security
Proceedings of the 2022 ACM SIGSAC Conference on Computer and Communications Security(2022)
摘要
Several recent research efforts have proposed Machine Learning (ML)-based solutions that can detect complex patterns in network traffic for a wide range of network security problems. However, without understanding how these black-box models are making their decisions, network operators are reluctant to trust and deploy them in their production settings. One key reason for this reluctance is that these models are prone to the problem of underspecification, defined here as the failure to specify a model in adequate detail. Not unique to the network security domain, this problem manifests itself in ML models that exhibit unexpectedly poor behavior when deployed in real-world settings and has prompted growing interest in developing interpretable ML solutions (e.g., decision trees) for "explaining'' to humans how a given black-box model makes its decisions. However, synthesizing such explainable models that capture a given black-box model's decisions with high fidelity while also being practical (i.e., small enough in size for humans to comprehend) is challenging.
更多查看译文
关键词
network security,ai/ml
AI 理解论文
溯源树
样例
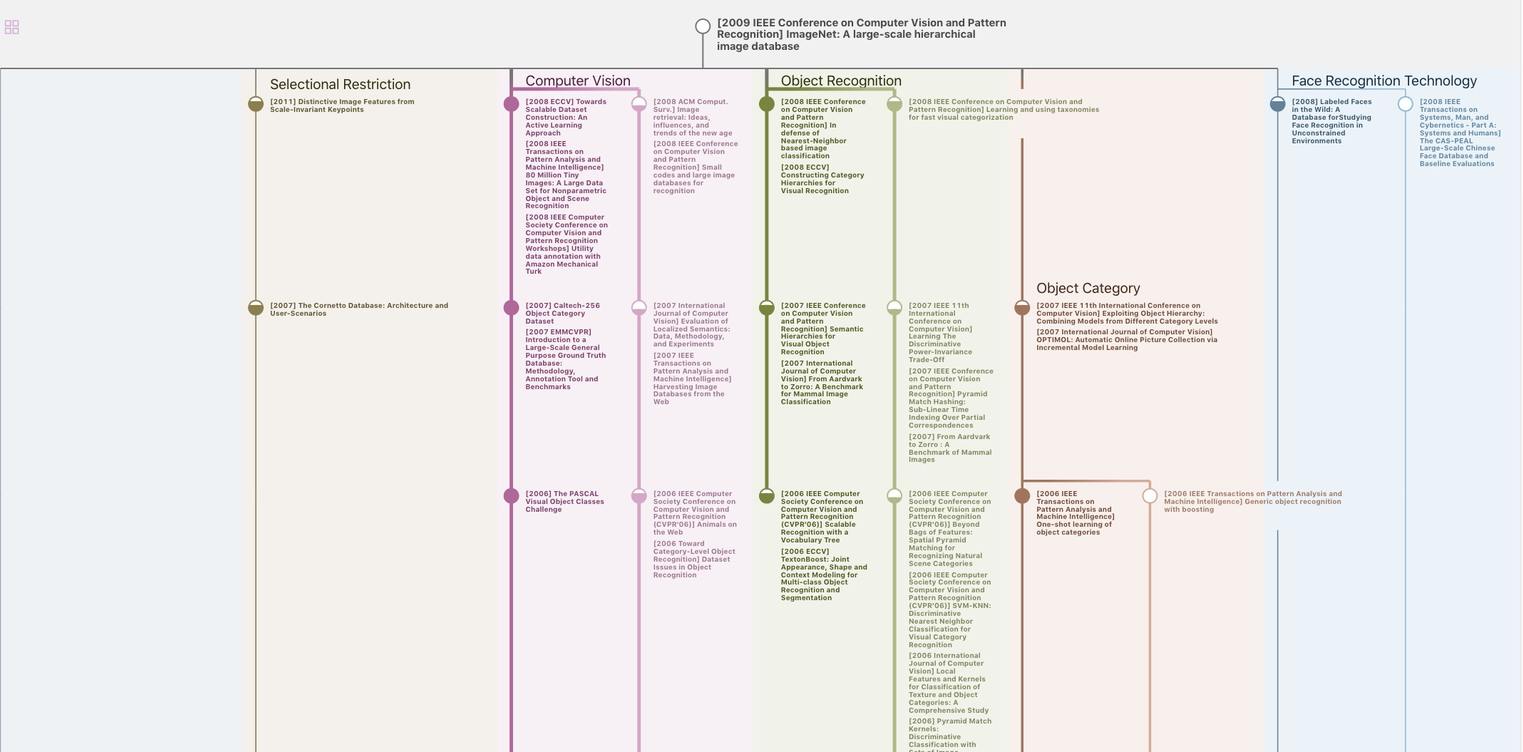
生成溯源树,研究论文发展脉络
Chat Paper
正在生成论文摘要