Deep Dual Attention Network for Precise Diagnosis of COVID-19 From Chest CT Images
IEEE transactions on artificial intelligence(2022)
摘要
Automatic diagnosis of COVID-19 using chest CT images is of great significance for preventing its spread. However, it is difficult to precisely identify COVID-19 due to the following problems: 1) the location and size of lesions can vary greatly in CT images; 2) its unique characteristics are often imperceptible in imaging findings. To solve these problems, a Deep Dual Attention Network ( $\textrm {D}^{\textrm {2}}\textrm {ANet}$ ) is proposed for accurate diagnosis of COVID-19 by integrating dual attention modules (DAMs) with different scales of the feature extractor, where DAM can adaptively detect relevant lesion regions to extract discriminative imaging features of COVID-19. Specifically, DAM is implemented by two parallel blocks: global attention block (GAB) and local attention block (LAB), in which GAB is designed to roughly locate the infected regions from the entire image by modeling global contexts, while LAB is developed to explicitly highlight subtle differences of COVID-19 from other viral pneumonia in the infected regions by learning detailed lesion information. Experimental results on several public datasets show that $\textrm {D}^{\textrm {2}}\textrm {ANet}$ outperforms the state-of-the-art approaches in various performance metrics.
更多查看译文
关键词
deep dual attention network,precise diagnosis
AI 理解论文
溯源树
样例
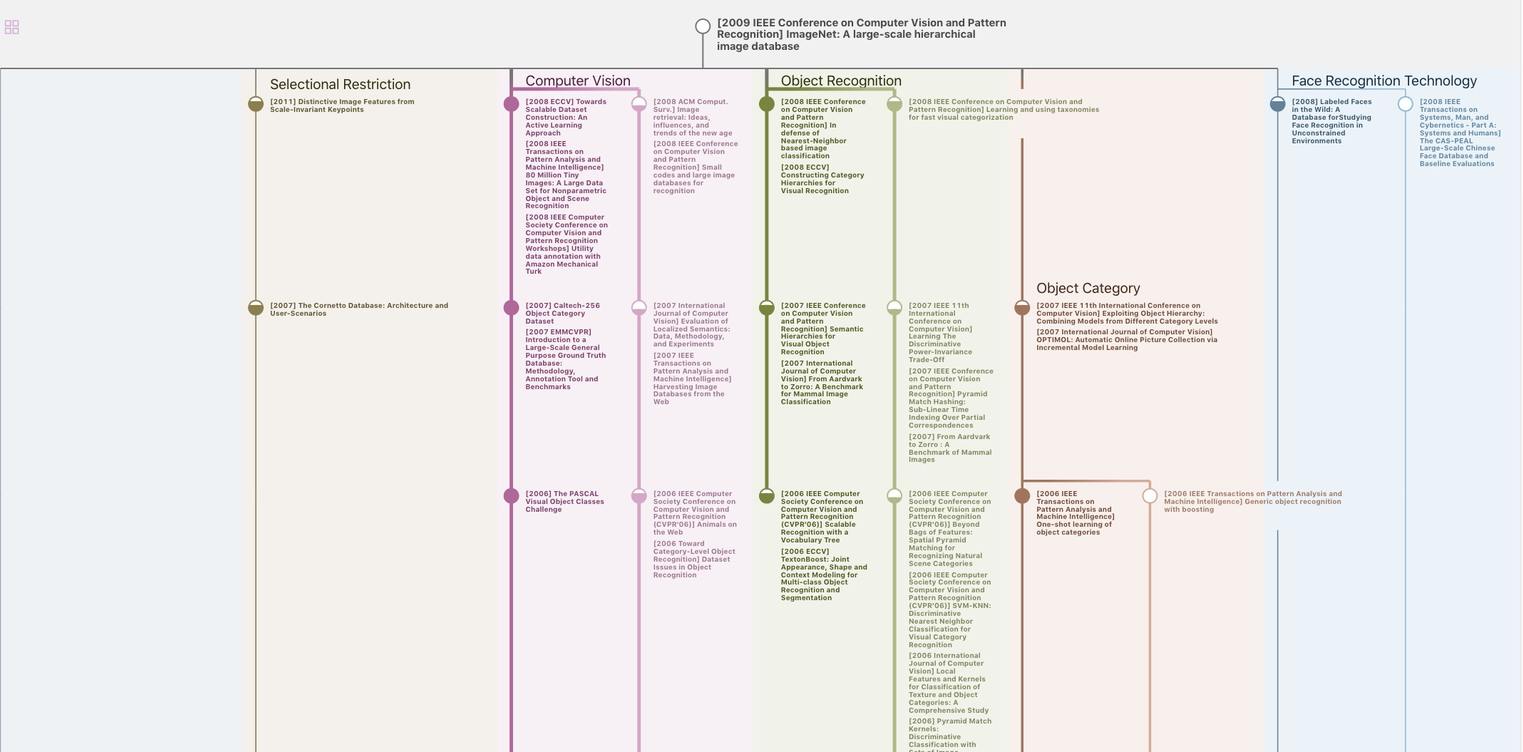
生成溯源树,研究论文发展脉络
Chat Paper
正在生成论文摘要