Preventing Inferences through Data Dependencies on Sensitive Data
arXiv (Cornell University)(2023)
摘要
Simply restricting the computation to non-sensitive part of the data may lead to inferences on sensitive data through data dependencies. Prior work on preventing inference control through data dependencies detect and deny queries which may lead to leakage, or only protect against exact reconstruction of the sensitive data. These solutions result in poor utility, and poor security respectively. In this paper, we present a novel security model called
full deniability
. Under this stronger security model, any information inferred about sensitive data from non-sensitive data is considered as a leakage. We describe algorithms for efficiently implementing full deniability on a given database instance with a set of data dependencies and sensitive cells. Using experiments on two different datasets, we demonstrate that our approach protects against realistic adversaries while hiding only minimal number of additional non-sensitive cells and scales well with database size and sensitive data.
更多查看译文
关键词
Inference Control,Data Dependencies,Inference Protection,Security & Privacy,Access Control
AI 理解论文
溯源树
样例
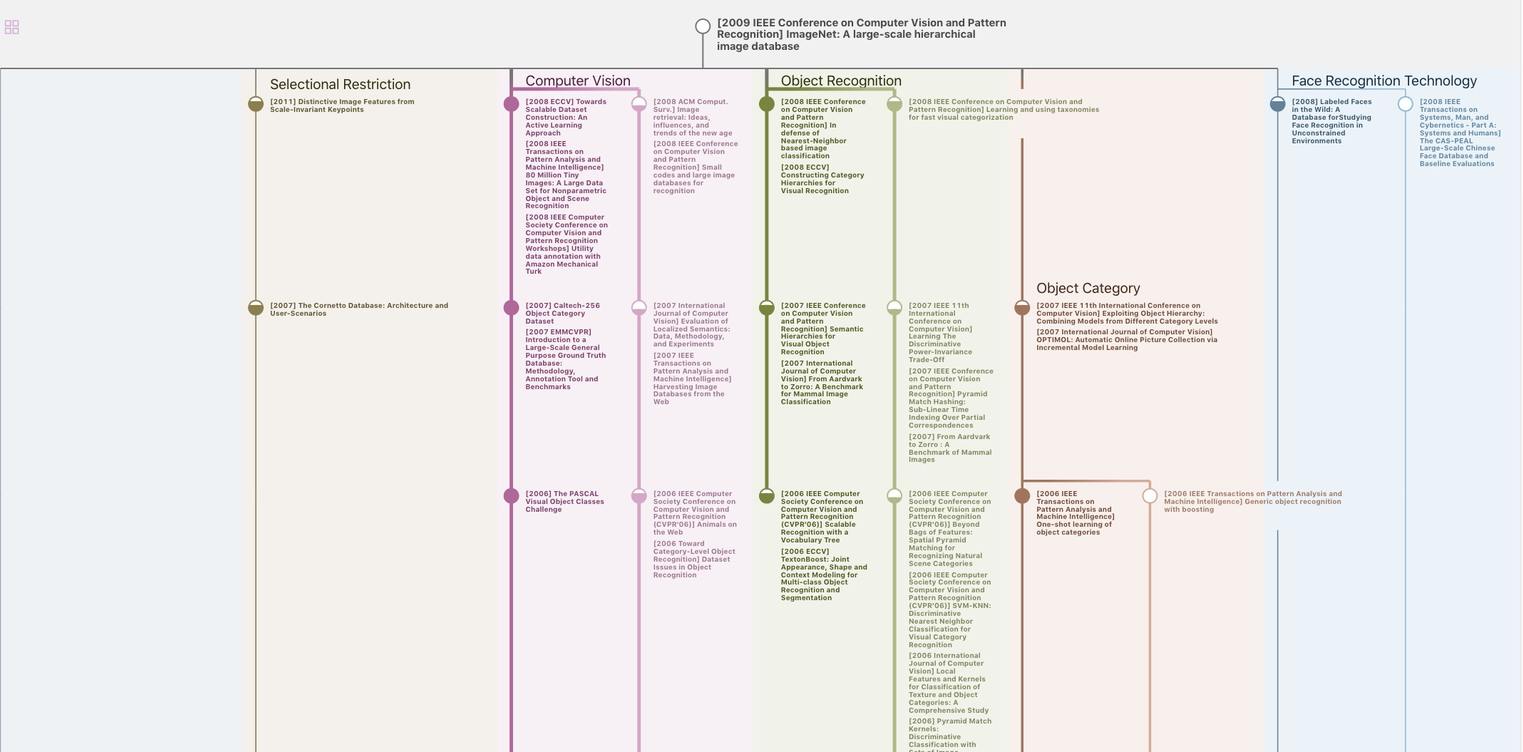
生成溯源树,研究论文发展脉络
Chat Paper
正在生成论文摘要