PROMPT: Learning Dynamic Resource Allocation Policies for Edge-Network Applications
arXiv (Cornell University)(2022)
摘要
A growing number of service providers are exploring methods to improve server utilization, reduce power consumption, and reduce total cost of ownership by co-scheduling high-priority latency-critical workloads with best-effort workloads. This practice requires strict resource allocation between workloads to reduce resource contention and maintain Quality of Service (QoS) guarantees. Prior resource allocation works have been shown to improve server utilization under ideal circumstances, yet often compromise QoS guarantees or fail to find valid resource allocations in more dynamic operating environments. Further, prior works are fundamentally reliant upon QoS measurements that can, in practice, exhibit significant transient fluctuations, thus stable control behavior cannot be reliably achieved. In this paper, we propose a novel framework for dynamic resource allocation based on proactive QoS prediction. These predictions help guide a reinforcement-learning-based resource controller towards optimal resource allocations while avoiding transient QoS violations due to fluctuating workload demands. Evaluation shows that the proposed method incurs 4.3x fewer QoS violations, reduces severity of QoS violations by 3.7x, improves best-effort workload performance, and improves overall power efficiency compared with prior work.
更多查看译文
关键词
dynamic resource allocation policies,edge-network
AI 理解论文
溯源树
样例
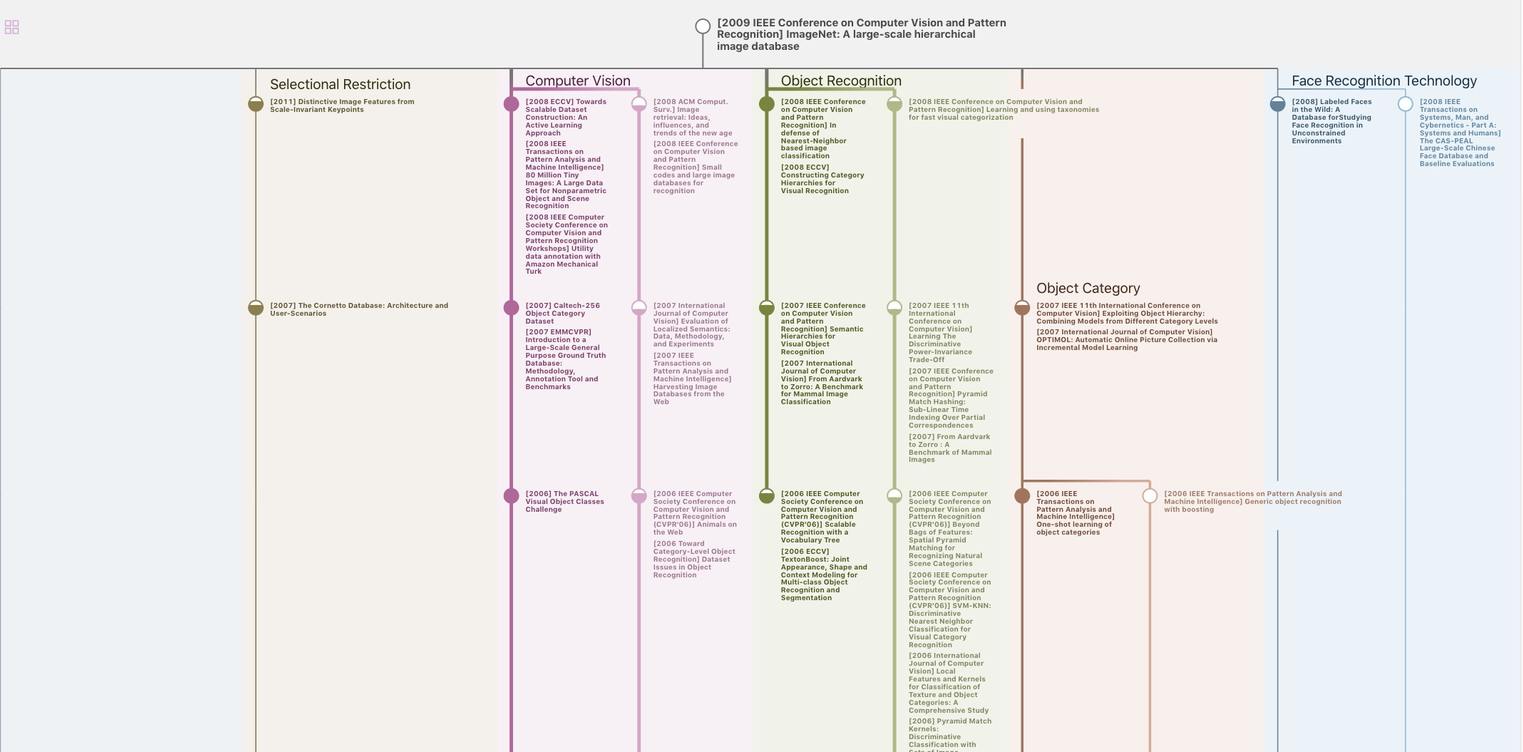
生成溯源树,研究论文发展脉络
Chat Paper
正在生成论文摘要