PEGG-Net: Pixel-Wise Efficient Grasp Generation in Complex Scenes
arXiv (Cornell University)(2022)
摘要
Vision-based grasp estimation is an essential part of robotic manipulation tasks in the real world. Existing planar grasp estimation algorithms have been demonstrated to work well in relatively simple scenes. But when it comes to complex scenes, such as cluttered scenes with messy backgrounds and moving objects, the algorithms from previous works are prone to generate inaccurate and unstable grasping contact points. In this work, we first study the existing planar grasp estimation algorithms and analyze the related challenges in complex scenes. Secondly, we design a Pixel-wise Efficient Grasp Generation Network (PEGG-Net) to tackle the problem of grasping in complex scenes. PEGG-Net can achieve improved state-of-the-art performance on the Cornell dataset (98.9%) and second-best performance on the Jacquard dataset (93.8%), outperforming other existing algorithms without the introduction of complex structures. Thirdly, PEGG-Net could operate in a closed-loop manner for added robustness in dynamic environments using position-based visual servoing (PBVS). Finally, we conduct real-world experiments on static, dynamic, and cluttered objects in different complex scenes. The results show that our proposed network achieves a high success rate in grasping irregular objects, household objects, and workshop tools. To benefit the community, our trained model and supplementary materials are available at https://github.com/HZWang96/PEGG-Net.
更多查看译文
关键词
pegg-net,pixel-wise
AI 理解论文
溯源树
样例
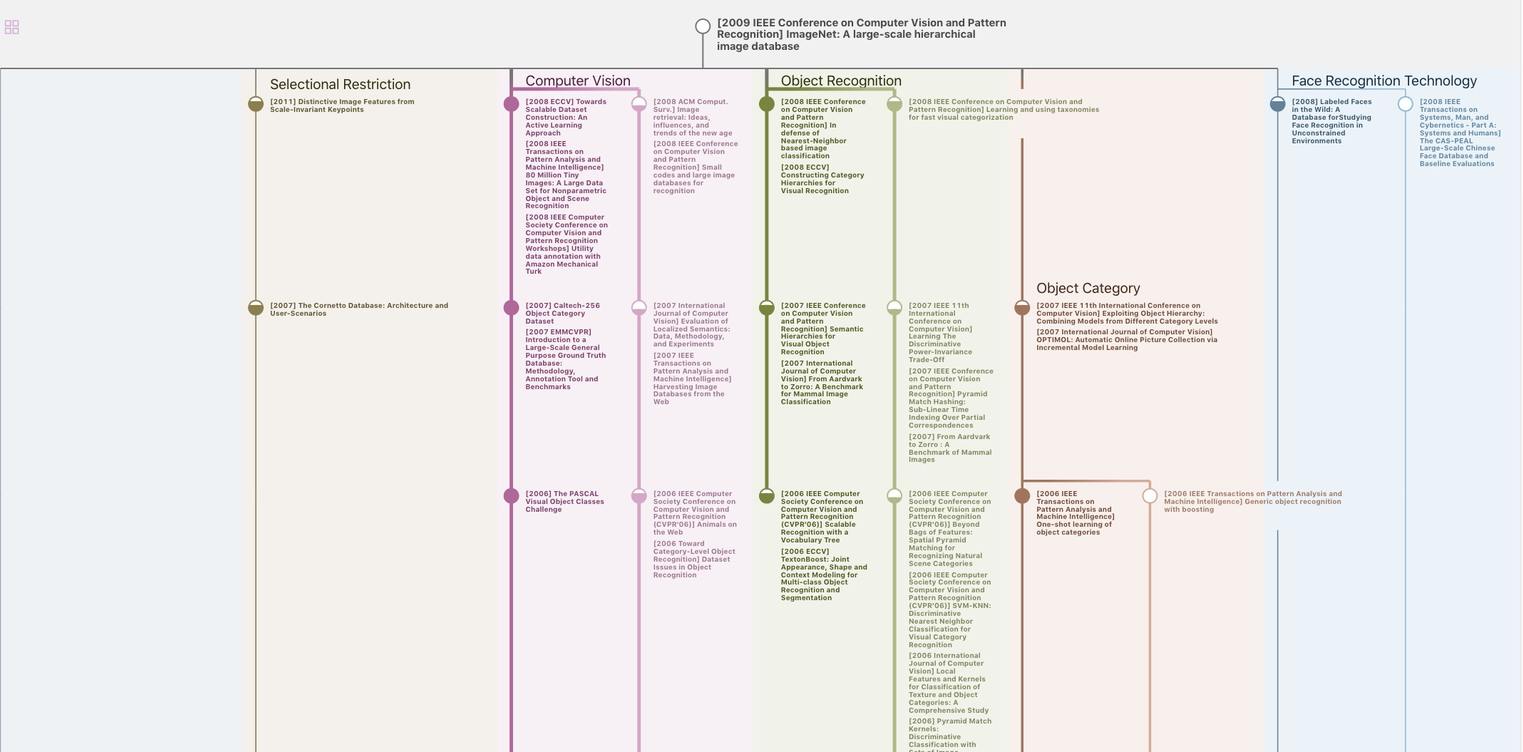
生成溯源树,研究论文发展脉络
Chat Paper
正在生成论文摘要