Abstract 15522: Segmentation Improves Deep Learning Accuracy for Differentiating Non-Ischemic and Ischemic Cardiomyopathy Using Cardiac Mri Cine Imaging
Circulation(2022)
摘要
Background: Deep learning (DL) models typically interpret images without prior knowledge of anatomic significance. However, pathophysiology is highly classified by human definitions according to underlying affected anatomy. Therefore, we examine the impact of introducing explicit knowledge of anatomy through cardiac contours on cardiac magnetic resonance images (CMR) to DL models. The DL models were then trained to differentiate between ischemic cardiomyopathy (ICM) and non-ischemic cardiomyopathy (NICM) using late gadolinium enhanced (LGE) CMR. Method: We evaluated 301 CMR studies; ICM (n=176) and NICM (n=125). Manual contouring of LGE images was performed using CVI42 (Circle, Ontario). We compared a radiomic and end-to-end DL approach to identify cardiomyopathy (CM) etiology from short axis LGE images. Patients are randomly assigned to training (70%) and testing (30%). Model performance was assessed with area under curve (AUC). Result: Table 1 shows the results of radiomic and deep learning approaches to differentiate NICM and ICM. Segmented/manually contoured images with removal of non-cardiac structures greatly improved classification accuracy across all deep learning models. The average improvement in AUC was 0.163 when using segmented images compared to the full images. Furthermore, the deep learning models outperformed the radiomics models. The best radiomic model and deep learning model achieved AUCs of 0.914 and 0.947, respectively. Both radiomic based models achieved AUCs above 0.874 while all 2D deep learning models with segmented images achieved AUCs above 0.875. The two 3D deep learning models which utilize 3D convolutions provided lower AUCs ranging between 0.743 and 0.900. Conclusion: Manual segmentation of LGE images improved the ability to train DL models with fairly small volumes of labeled data, resulting in higher classification accuracy. Table1 AUCs of various models.
更多查看译文
关键词
non-ischemic cardiomyopathy,deep learning,deep learning accuracy,segmentation,imaging
AI 理解论文
溯源树
样例
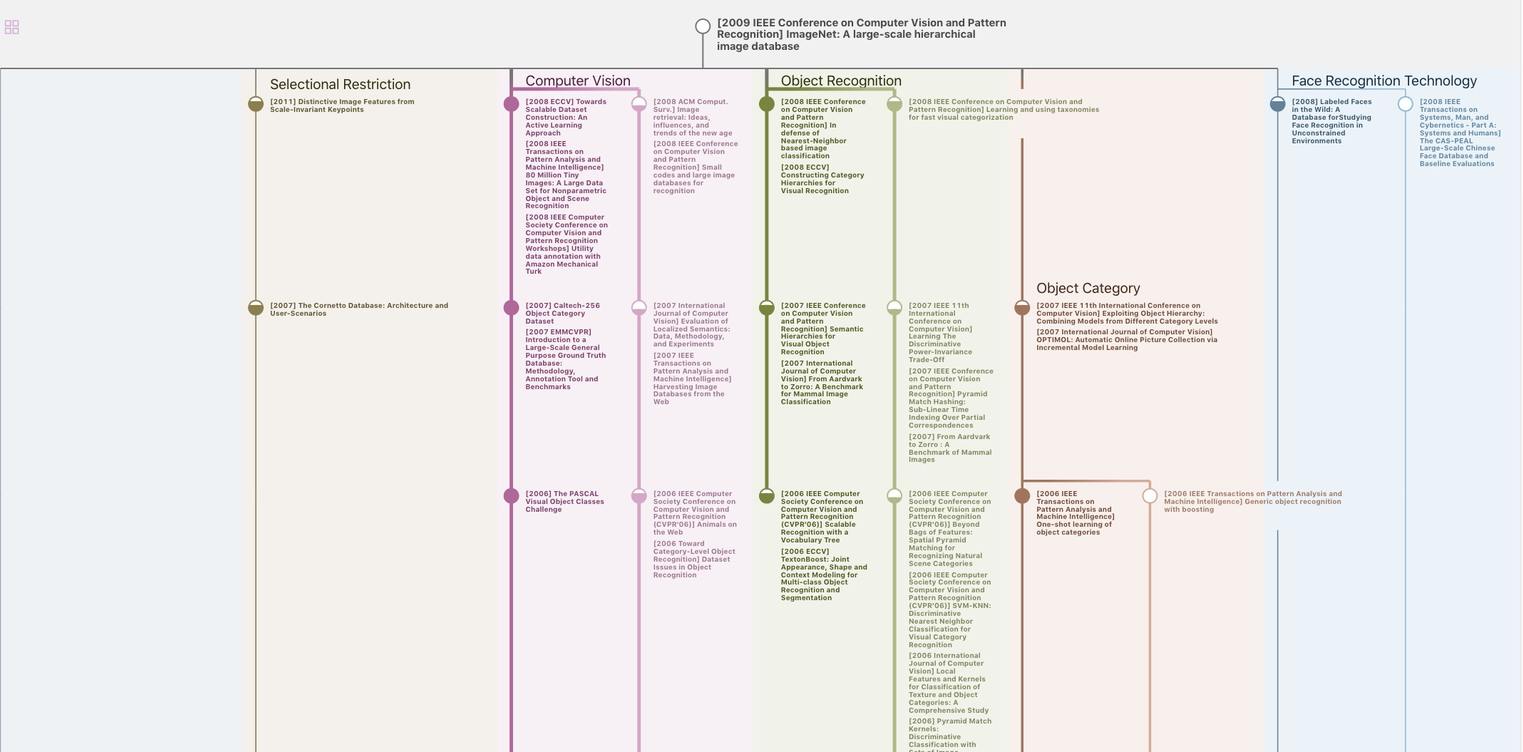
生成溯源树,研究论文发展脉络
Chat Paper
正在生成论文摘要