A Unified Approach to Reinforcement Learning, Quantal Response Equilibria, and Two-Player Zero-Sum Games
arXiv (Cornell University)(2022)
摘要
This work studies an algorithm, which we call magnetic mirror descent, that is inspired by mirror descent and the non-Euclidean proximal gradient algorithm. Our contribution is demonstrating the virtues of magnetic mirror descent as both an equilibrium solver and as an approach to reinforcement learning in two-player zero-sum games. These virtues include: 1) Being the first quantal response equilibria solver to achieve linear convergence for extensive-form games with first order feedback; 2) Being the first standard reinforcement learning algorithm to achieve empirically competitive results with CFR in tabular settings; 3) Achieving favorable performance in 3x3 Dark Hex and Phantom Tic-Tac-Toe as a self-play deep reinforcement learning algorithm.
更多查看译文
关键词
reinforcement learning,equilibria,two-player,zero-sum
AI 理解论文
溯源树
样例
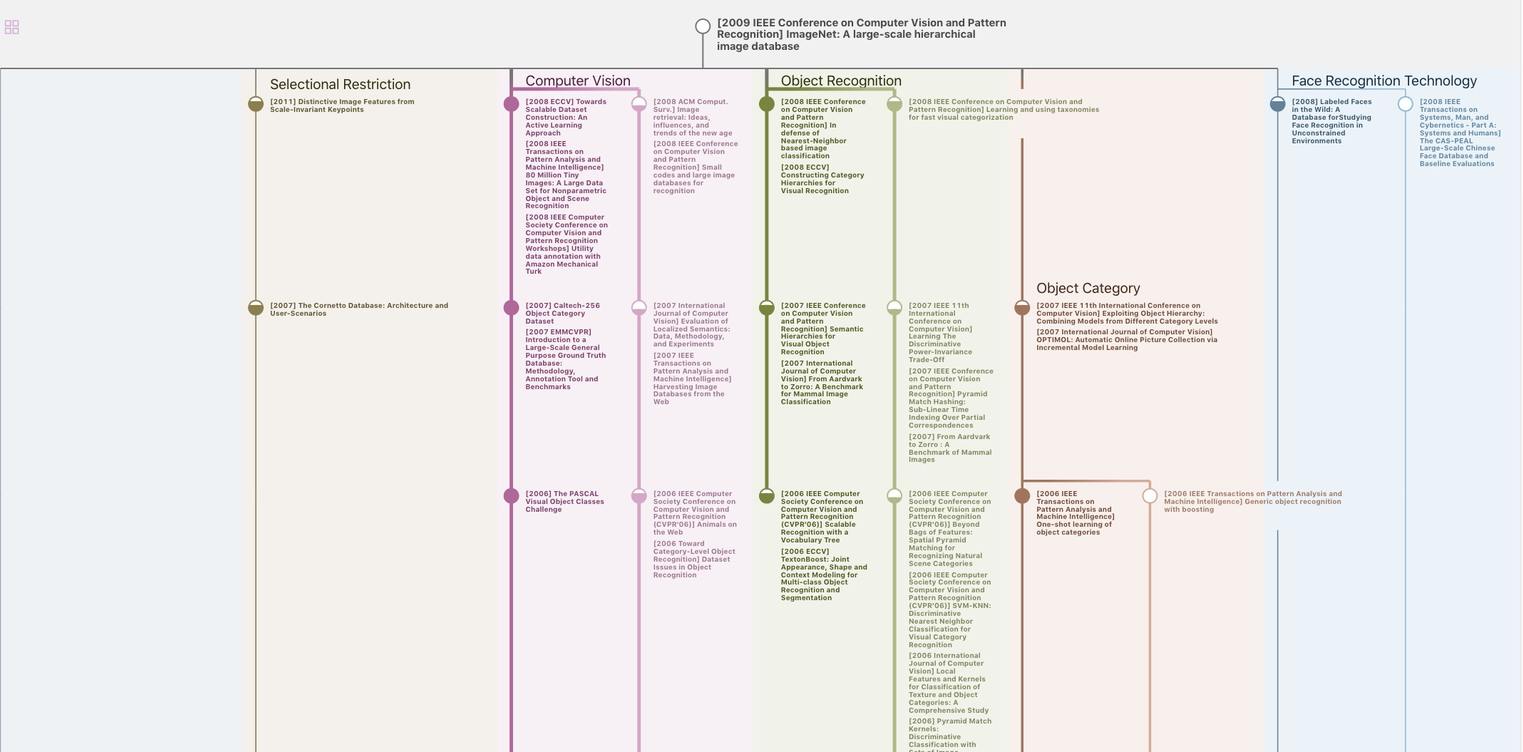
生成溯源树,研究论文发展脉络
Chat Paper
正在生成论文摘要