Improving the prediction of white matter hyperintensities evolution in brain MRI of patients with small vessel disease using stroke lesions information
bioRxiv (Cold Spring Harbor Laboratory)(2022)
摘要
ABSTRACT Predicting the evolution of white matter hyperintensities (WMH), a common feature in brain magnetic resonance imaging (MRI) scans of older adults (i.e., whether WMH will grow, remain stable, or shrink with time) is important for personalised therapeutic interventions. However, this task is difficult mainly due to the myriad of vascular risk factors and comorbidities that influence it, and the low specificity and sensitivity of the image intensities and textures alone for predicting WMH evolution. Given the predominantly vascular nature of WMH, in this study, we evaluate the impact of incorporating stroke lesion information to a probabilistic deep learning model to predict the evolution of WMH 1-year after the baseline image acquisition, taken soon after a mild stroke event, using T2-FLAIR brain MRI. The Probabilistic U-Net was chosen for this study due to its capability of simulating and quantifying the uncertainties involved in the prediction of WMH evolution. We propose to use an additional loss called volume loss to train our model, and incorporate stroke lesions information, an influential factor in WMH evolution. Our experiments showed that jointly segmenting the disease evolution map (DEM) of WMH and stroke lesions, improved the accuracy of the DEM representing WMH evolution. The combination of introducing the volume loss and joint segmentation of DEM of WMH and stroke lesions outperformed other model configurations with mean volumetric absolute error of 0.0092 ml (down from 1.7739 ml ) and 0.47% improvement on average Dice similarity coefficient in shrinking, growing and stable WMH.
更多查看译文
关键词
stroke lesions information,brain mri,white matter hyperintensities evolution,small vessel disease
AI 理解论文
溯源树
样例
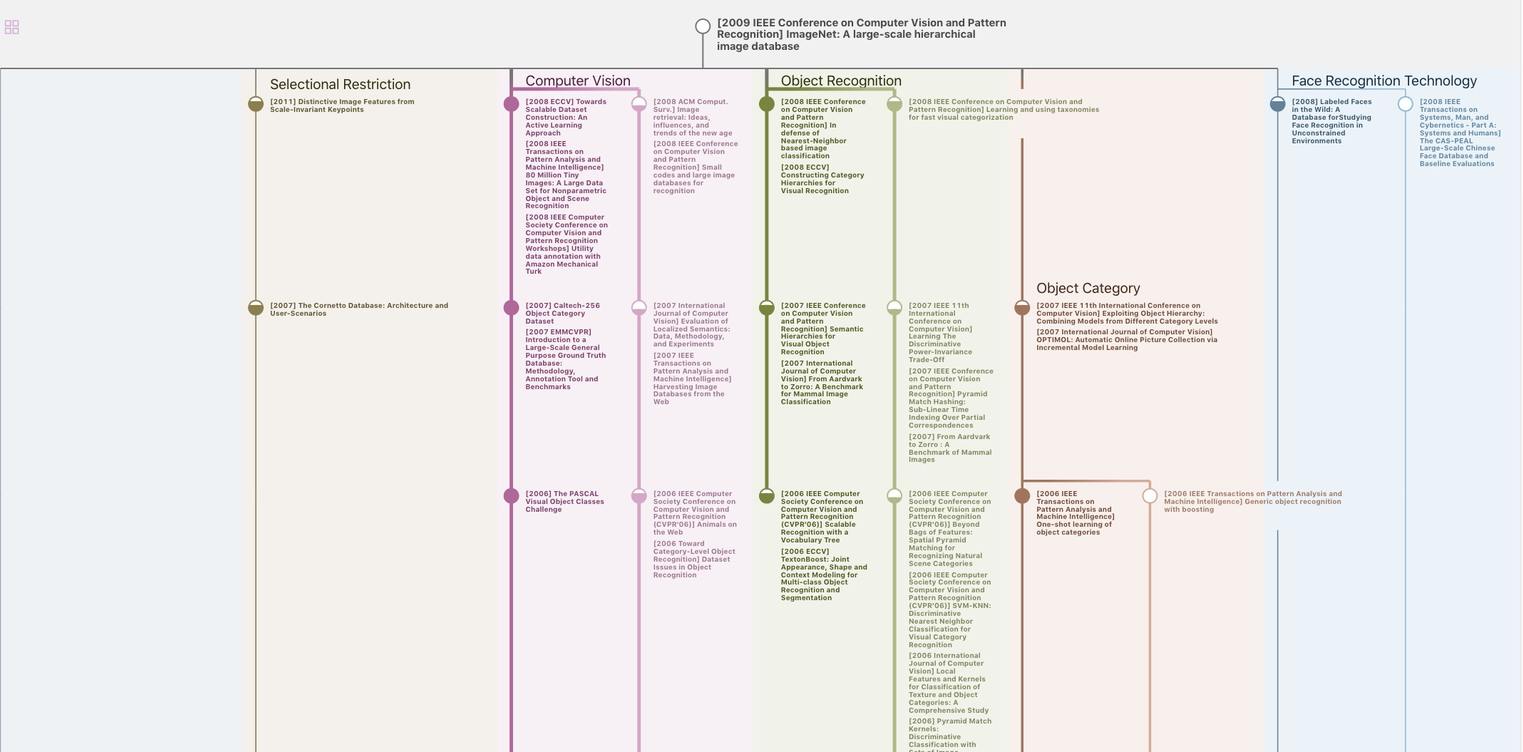
生成溯源树,研究论文发展脉络
Chat Paper
正在生成论文摘要