CA-FER: Mitigating Spurious Correlation with Counterfactual Attention in Facial Expression Recognition
IEEE Transactions on Affective Computing(2023)
摘要
Although facial expression recognition based on deep learning has become a major trend, existing methods have been found to prefer learning spurious statistical correlations and non-robust features during training. This degenerates the model's generalizability in practical situations. One of the research fields mitigating such misperception of correlations as causality is causal reasoning. In this paper, we propose a learnable counterfactual attention mechanism, CA-FER, that uses causal reasoning to simultaneously optimize feature discrimination and diversity to mitigate spurious correlations in expression datasets. To the best of our knowledge, this is the first work to study the spurious correlations in facial expression recognition from a counterfactual attention perspective. Extensive experiments on a synthetic dataset and four public datasets demonstrate that our method outperforms previous methods, which shows the effectiveness and generalizability of our learnable counterfactual attention mechanism for the expression recognition task.
更多查看译文
关键词
Causal learning,facial expression recognition,spurious correlation
AI 理解论文
溯源树
样例
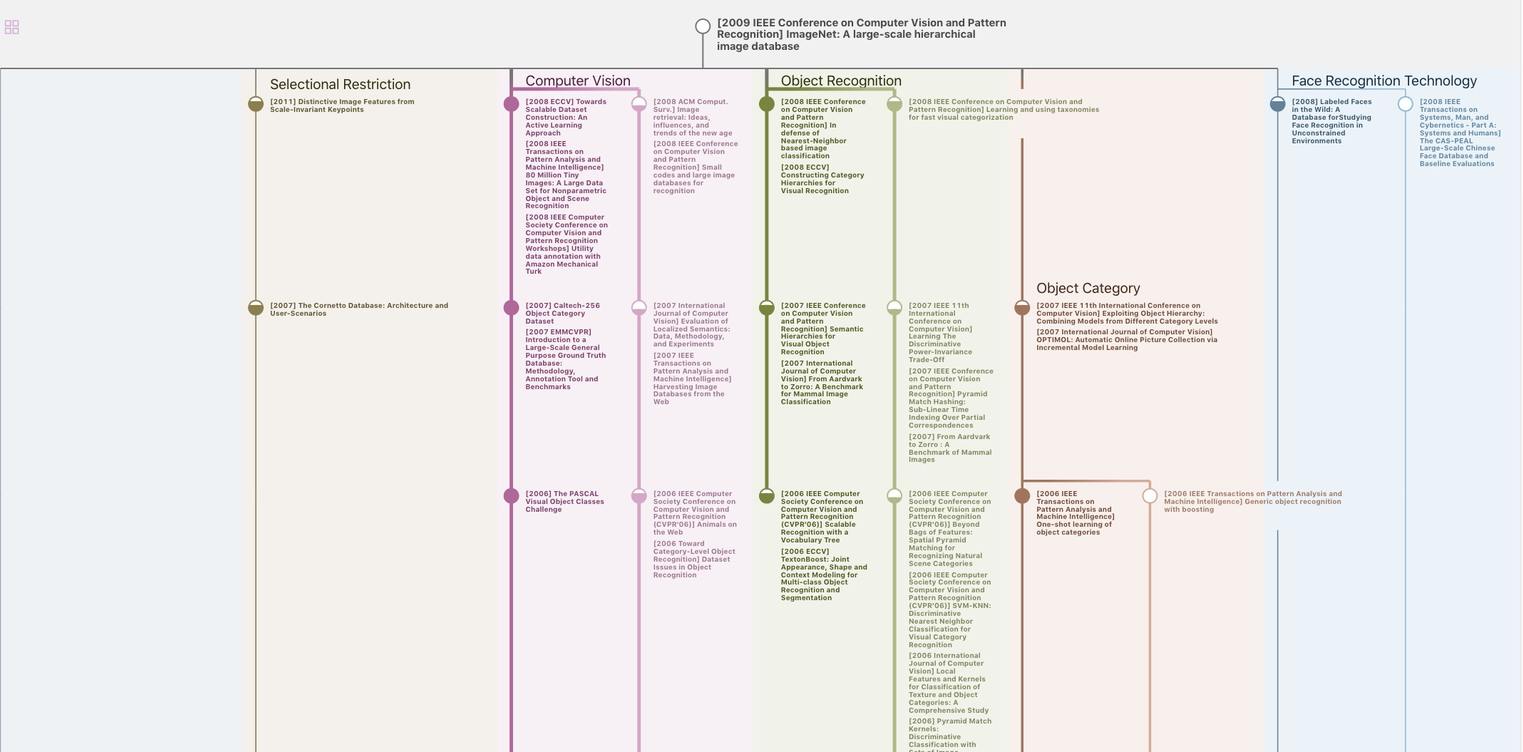
生成溯源树,研究论文发展脉络
Chat Paper
正在生成论文摘要