Tailoring the microstructure and mechanical properties of (CrMnFeCoNi)100-C high-entropy alloys: Machine learning, experimental validation, and mathematical modeling
Current Opinion in Solid State & Materials Science(2023)
摘要
As a common thermomechanical treatment route, “cold rolling and annealing” is widely used for the processing and grain refinement of interstitial-containing high-entropy alloys (HEAs). The interrelationship between the parameters of this process, the content of interstitial elements, and their interactions are outstanding challenges and areas of open discussion. Accordingly, the data-driven machine learning approach is a favorable choice for tuning the microstructure and mechanical properties, which needs to be systematically investigated. In the present work, these subjects were addressed in terms of correlating the thermomechanical processing parameters and chemical composition with the recrystallization and grain growth behaviors, grain size, carbide precipitation, and the resulting tensile yield stress for the model (CrMnFeCoNi)100-xCx HEAs. For this purpose, machine learning models based on adaptive neuro-fuzzy inference system (ANFIS), backpropagation artificial neural network (BP-ANN), and support network machine (SVM), as well as mathematical relationships and equations for the contribution of each strengthening mechanism were proposed and verified by extensive experimental work, which shed light on the design and prediction of the microstructure and properties of HEAs.
更多查看译文
关键词
alloys,microstructure,mechanical properties,high-entropy
AI 理解论文
溯源树
样例
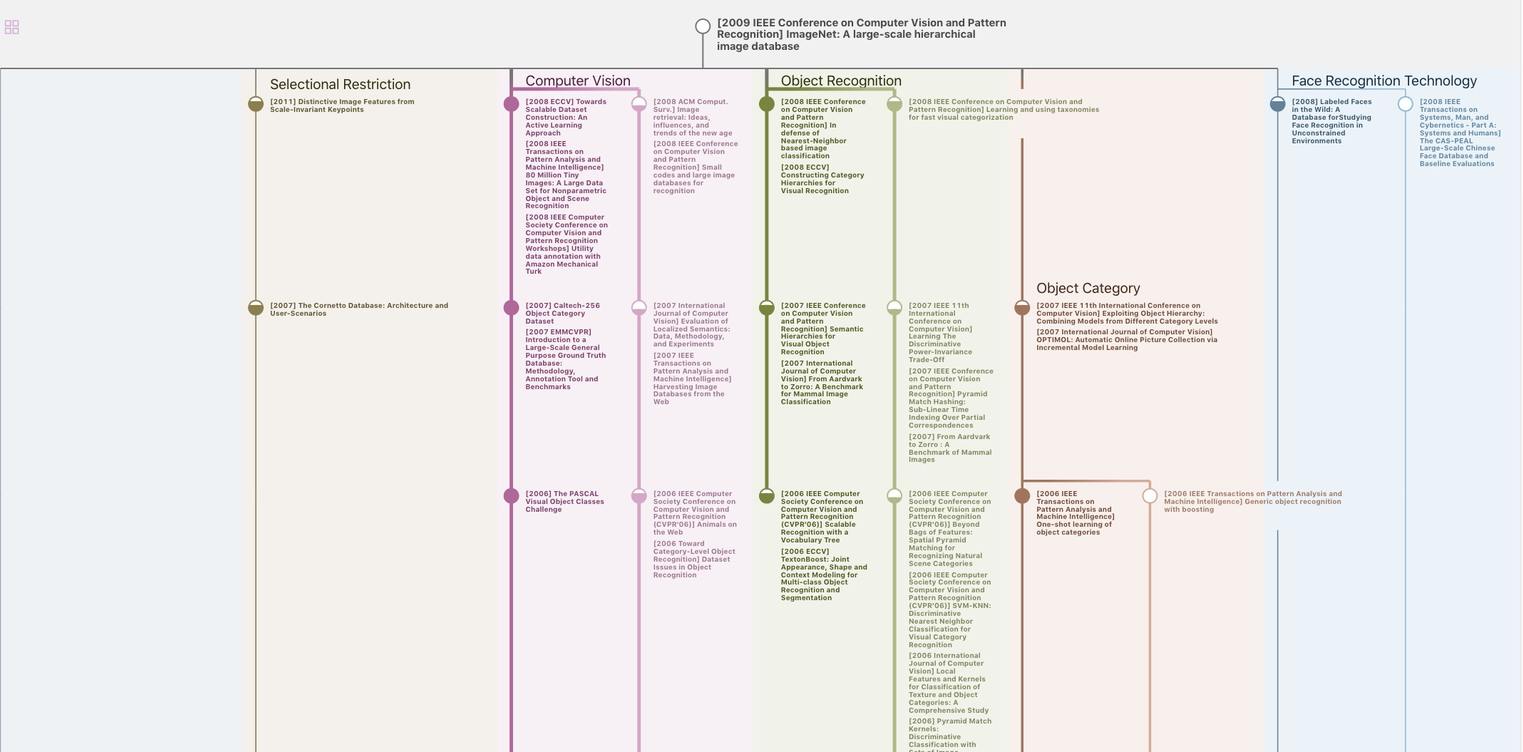
生成溯源树,研究论文发展脉络
Chat Paper
正在生成论文摘要