Routine blood test markers for predicting liver disease post HBV infection: precision pathology and pattern recognition
Diagnosis(2023)
摘要
Background Early stages of hepatitis B virus (HBV) infection usually involve inflammation of the liver. Patients with chronic infection have an increased risk of progressive liver fibrosis, cirrhosis, and life-threatening clinical complications of end-stage hepatocellular carcinoma (HCC). Content Early diagnosis of hepatic fibrosis and timely clinical management are critical to controlling disease progression and decreasing the burden of end-stage liver cancer. Fibrosis staging, through its current gold standard, liver biopsy, improves patient outcomes, but the clinical procedure is invasive with unpleasant post-procedural complications. Routine blood test markers offer promising diagnostic potential for early detection of liver disease without biopsy. There is a plethora of candidate routine blood test markers that have gone through phases of biomarker validation and have shown great promise, but their current limitations include a predictive ability that is limited to only a few stages of fibrosis. However, the advent of machine learning, notably pattern recognition, presents an opportunity to refine blood-based non-invasive models of hepatic fibrosis in the future. Summary In this review, we highlight the current landscape of routine blood-based non-invasive models of hepatic fibrosis, and appraise the potential application of machine learning (pattern recognition) algorithms to refining these models and optimising clinical predictions of HBV-associated liver disease. Outlook Machine learning via pattern recognition algorithms takes data analytics to a new realm, and offers the opportunity for enhanced multi-marker fibrosis stage prediction using pathology profile that leverages information across patient routine blood tests.
更多查看译文
关键词
routine blood test markers,liver disease,hbv,precision pathology
AI 理解论文
溯源树
样例
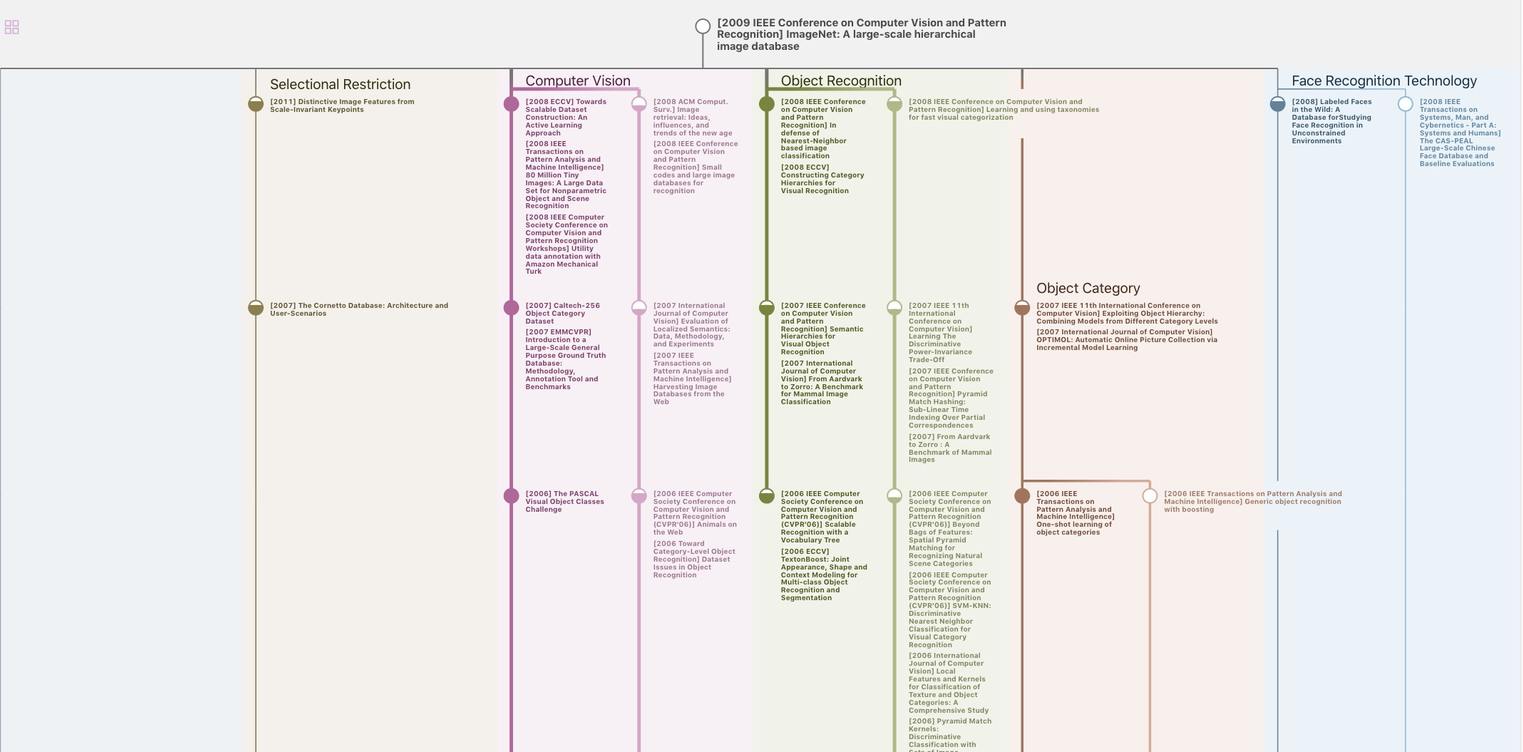
生成溯源树,研究论文发展脉络
Chat Paper
正在生成论文摘要