Inference of Galaxy Clusters’ Mass Radial Profiles from Compton-y Maps with Deep Learning Techniques
Astrophysics and space science proceedings(2023)
摘要
Clusters of galaxies are crucial tracers of the history of structure formation, and their mass function at different epochs is of fundamental importance for constraining cosmological parameters. Therefore, it is essential to infer the mass of observed clusters, which unfortunately is not directly observable and is affected by several biases related to applied observational estimates. To overcome these obstacles, we developed a machine learning algorithm capable of inferring the 3D cumulative radial profiles of the total mass and gas mass in galaxy clusters from the Sunyaev-Zel’dovich effect heat maps. In recent work, convolutional neural networks (CNNs) were applied to full-sky Compton-y maps to estimate cluster masses defined at a fixed aperture radius corresponding to 500 times the critical overdensity. We now extend this study to estimate the radial profiles of the total mass and gas mass of the clusters. We produced about 73,000 images along various lines of sight using 2522 clusters simulated by project at redshift $$z < 0.12$$ and trained a model combining an autoencoder and a random forest. We selected a samples with masses in the range $$10^{13.5} \leq M_{200}(h^{-1}M\odot ) \leq 10^{15.5}$$ and different dynamical states. The obtained model was able to reconstruct unbiased profiles with a dispersion of about $$10\%$$ , slightly incremental toward the core and periphery of the cluster. We show that both the accuracy and precision of this method show a slight dependence on the dynamical state, but not on the mass of the cluster.
更多查看译文
关键词
galaxy clusters,mass radial profiles,deep learning techniques
AI 理解论文
溯源树
样例
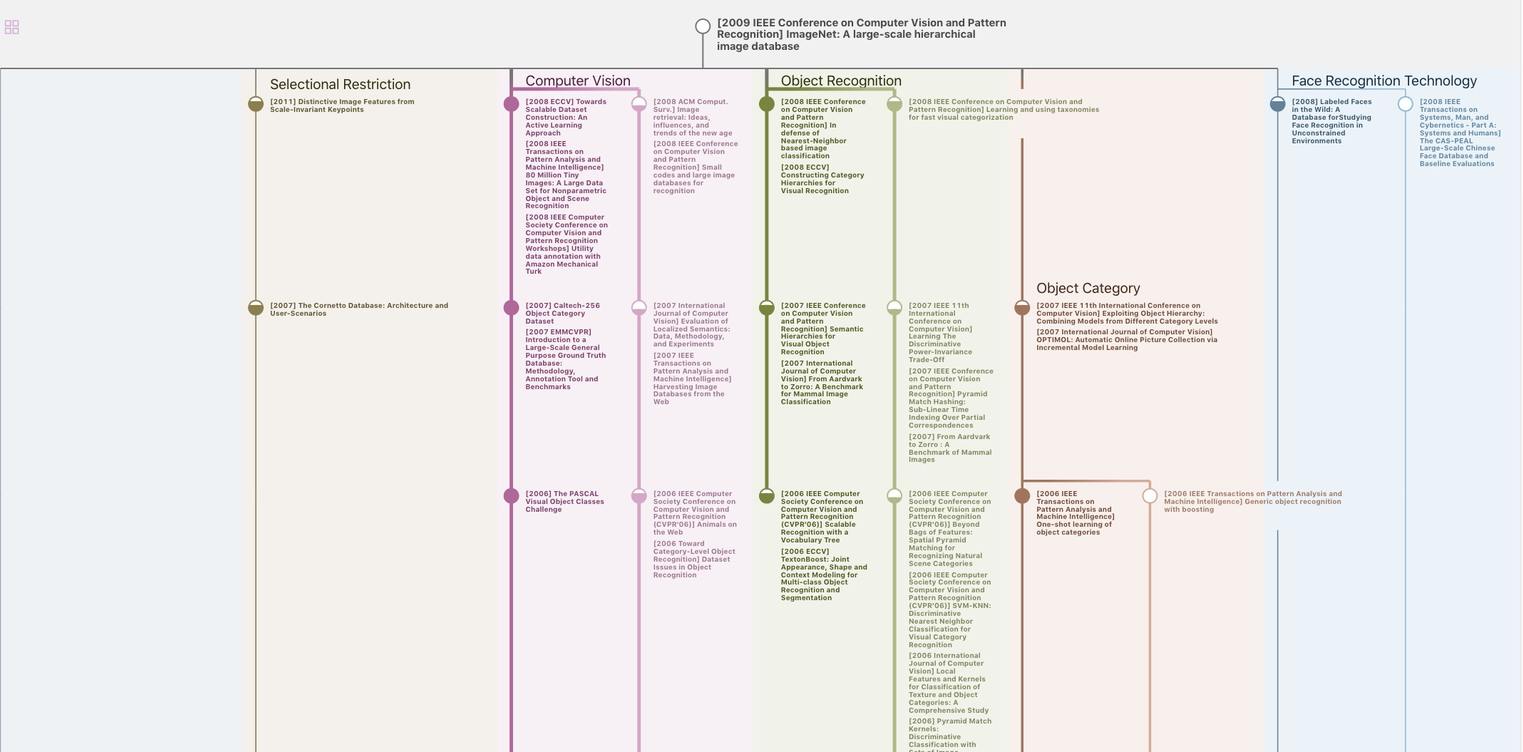
生成溯源树,研究论文发展脉络
Chat Paper
正在生成论文摘要