Ps-bpc01-6: deep learning is useful for the pathological analyses of human kidney biopsy images
Journal of Hypertension(2023)
摘要
Objective: The kidney plays a pivotal role in the maintenance of blood pressure. Non-neoplastic kidney biopsy images yield a great deal of diagnostic information, however, several studies have shown that there is room for improvement in pathological diagnostic reproducibility. Recently, deep learning has made major breakthroughs in various tasks. Therefore, we tried to analyze human kidney biopsy images using deep learning. Design and Method: We analyzed 5002 non-neoplastic kidney biopsy whole slide images (WSIs) obtained from 24 facilities in Japan using deep learning. Images of periodic acid-Schiff stained kidney sections were analyzed. Results: WSIs have billions of pixels, and deep neural networks (NNs) cannot accept such a huge image as an input. Therefore, we prepared 88 μm square 6,125,990 patch images (PIs) from the WSIs, and trained NNs with these PIs. We manually selected 259,682 typical PIs from the whole set of PIs, and annotated them into 56 classes. Using these annotated PIs, we trained EfficientNets B0-B7, whose network depth and width are carefully balanced for various input resolutions (224 to 600 pixels), and found that EfficientNetB4 (hereafter referred to CNN_Man) achieved the best F1 score. Using histological parameters obtained from renal biopsy reports made by nephrologists and pathologists at each facility, we confirmed the reliability of CNN_Man-based prediction. We also finely visualized histological features of WSIs based on CNN_Man prediction (Figure). In addition to these supervised histological analyses, we tried to extract disease features in a loosely supervised manner. In this loosely supervised learning, we tried to extract disease-related rather than histology-related glomerular features by training EfficientNetB4 using final diagnosis as true labels. It is noteworthy that the final-diagnosis-based training is not typical supervised learning because each disease class contains patch images with various histological features to varying degrees. We extracted glomerulus-related PIs by using CNN_Man, and then labeled these PIs into 20 final-diagnosis categories. EfficientNetB4 (hereafter CNN_Diag) was trained with the 20 class images in a 5-fold cross-validation manner. Using UMAP, we visualized the final layer parameters of CNN_Diag corresponding to each PI. This approach divided PIs from IgA nephropathy into many clusters, and each cluster reflected blood pressure, renal function, age, etc., even though they were not explicitly given as teacher labels. Conclusions: The analysis of kidney biopsy images using deep learning may be useful for the classification of renal diseases based on new perspectives.
更多查看译文
关键词
human kidney,deep learning,ps-bpc
AI 理解论文
溯源树
样例
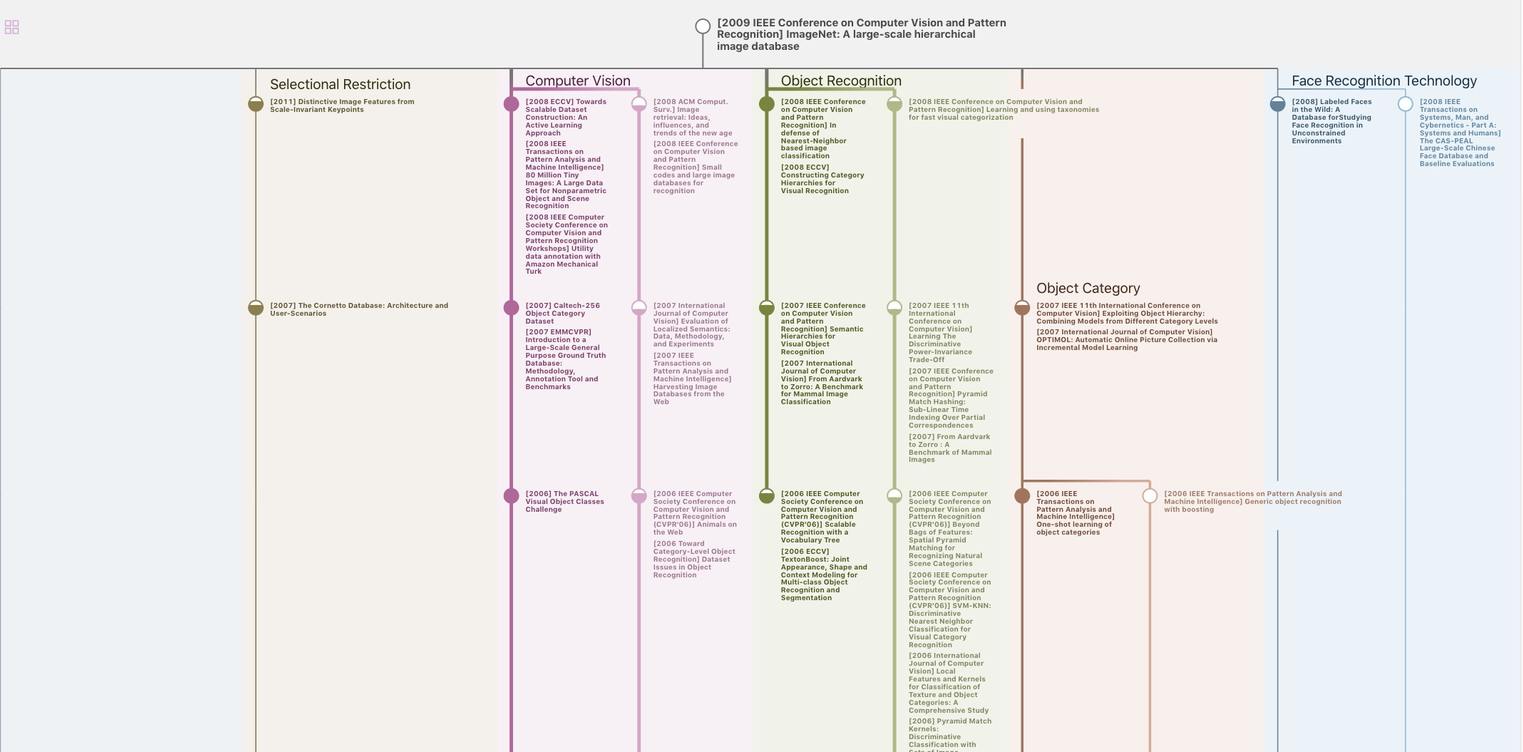
生成溯源树,研究论文发展脉络
Chat Paper
正在生成论文摘要