Performance of Machine Learning Models for Predicting High-Severity Symptoms in Multiple Sclerosis
Research Square (Research Square)(2023)
摘要
Abstract Current care in multiple sclerosis (MS) primarily relies on infrequently obtained data such as magnetic resonance imaging (MRI), clinical laboratory tests or clinical history, resulting in subtle changes that may occur between visits being missed. Mobile technology enables continual collection of data and can pave the path for predicting complex aspects of MS such as symptoms and disease courses. To this end, we conducted a first-of-its-kind observational study called MS Mosaic. First, we developed and publicly launched a mobile app for collecting longitudinal data from MS subjects in the United States. Second, we ran the study across 3 years in order to capture complex patterns for this slow progressing disease. Finally, we retrospectively developed three classical ML methods and two deep learning models to accurately and continually predict the incidence of five high-severity symptoms (fatigue, sensory disturbance, walking instability, depression or anxiety and cramps) three months in advance.
更多查看译文
关键词
multiple sclerosis,machine learning models,high-severity
AI 理解论文
溯源树
样例
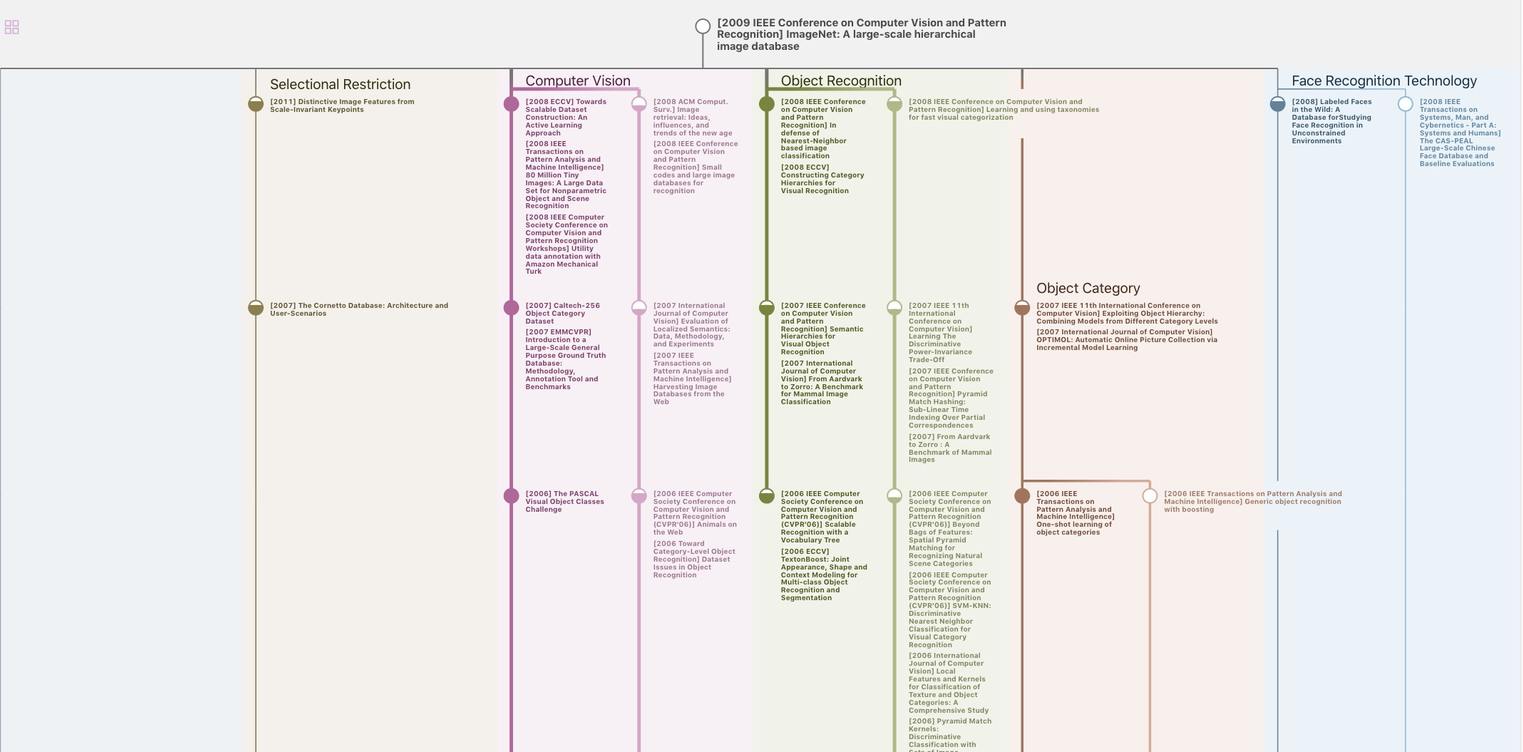
生成溯源树,研究论文发展脉络
Chat Paper
正在生成论文摘要