Lung Ventilation Modeling and Medical Assessment
Elsevier eBooks(2023)
摘要
Lung mechanics involves inhalation and exhalation pressure and volume changes. Figure 6.14 illustrates the lung ventilation model (LVM). It can be seen, from the figure, that three pressures are involved in the ventilatory function, namely atmospheric pressure or pressure at the mouth (Pm), alveolar pressure (Pa), and pleural pressure (Pp). The pressure gradient between the atmospheric pressure at the mouth (Pm) and alveolar pressures (Pa) causes respiration to occur. During inspiration, Pa < Pm, and air enters the lungs; during expiration, Pa > Pm, and air is expelled out of the lungs passively. This pressure differential between Pm and Pp provides the driving pressure (PL) for gas flow, in terms of the (1) elastic recoil pressure (Pa – Pp = Pel) of the lumped alveolar chamber, and (2) the pressure differential between Pm and Pa, expressed as R(dV/dt), where V is the lung volume and R is the resistance to flow. These relationships are expressed as: PL = (Pm – Pp) = (Pm – Pa) + (Pa – Pp) = R(dV/dt) + Pel = R(dV/dt) + V/C + Pel0 (at end of expiration), where C is the lung compliance. This then results in the lung ventilation model (LVM) governing differential Equation (6.52): R(dV/dt) + V/C = PL – Pel0 = PN(t), the driving pressure This differential equation has R and C as its parameters, which can then be employed to determine the lung disease states. Now, PN(t) is obtained from the monitored data in Figure 6.16A, and is represented by Equation (6.55). Based on that, the V solution of the governing differential Equation (6.52) is given by Equation (6.56), wherein the term (RaCa) is denoted by τa. By matching this V expression with the volume data in Figure 6.16B, we obtain the average values of the lung model parameters, as: Ca = 0.231 L(cm H2O)−1, Ra = 2.275 cm H2O sL−1, τa = 0.485 s, as given by Equation (6.59). In this way, the LVM enables evaluation of the lung compliance (C) and airway resistance-to-airflow (R), which are the parameters of the governing equation. We can also analytically determine the values of C and R from the volume curve, as shown by (6.60), (6.61), (6.62). Since lung disease will influence the values of R and C, these parameters can be employed to diagnose lung diseases. For instance, in the case of emphysema, the destruction of lung tissue between the alveoli produces a more compliant lung, and hence results in a larger value of C. In asthma, there is increased airway resistance (R) due to contraction of the smooth muscle around the airways. In lung fibrosis, the membranes between the alveoli thicken and hence lung compliance (C) decreases. Thus, by determining the normal and diseased lungs’ ranges of the parameters R and C, we can employ this simple lung-ventilation model for differential diagnosis. Now although R and C can in this way be usefully employed as diagnostic parameters for lung diseases, we can go one step further and formulate a novel nondimensional ventilatory performance index (VTI) to characterize ventilatory function. For this, let us look into “noninvasively determinable ventilatory index” in Section 6.8. In order to formulate a noninvasively determinable ventilatory index based on the governing Equation (6.53), we need to recognize that in this case PN(t) (and hence Pi, ω, and ci) will be unknowns, and we hence need to re-designate the model parameters and indicate their identification procedure. For that, we can make use of the volume–time data to facilitate evaluation of the following parameters: PiC, ωi, cj, and τa. So we can refer to the lung volume curve in Figure 6.16B, and fit into it the expression for V(t) given by Equation (6.56), to obtain the values of these four parameters as shown by (6.67), (6.68), (6.69). We then formulate the noninvasive ventilatory index, as(6.70)VTI2=(BR)τ[TV]2|P1C||P2C||P3C|=(BR)R[TV]2|P1P2P3C2| It is seen that VTI2 can in fact be expressed in terms of P1,P2,P3 and R, C. This VTI2 index can be evaluated by computing the values of BR and τ, along with (PiC), as given by (6.67), (6.68), (6.69). Then, after evaluating VTI2 for a number of patients, its distribution can enable us to categorize and differentially diagnose patients with various lung disorders and diseases. Then in Section 6.11, we have developed a method for noninvasive assessment of lung status, and determination of lung compliance (C) and resistance-to-airflow (R), based on Figure 6.18. In this method, we first analytically represent the lung pressure curve between its two inflection points, in terms of three parameters Pk, P0, and ωp. We then put down the governing differential equation of lung response to this pressure. In this equation, we substitute the values of V and V˙ at times related to the inflection points and maximum pressure. We thereby get equations involving the pressure parameters Pk and P0, and C and R. By matching these equations with the volume curve, we obtain their average values. We have then developed a novel nondimensional lung ventilatory index LVI2 involving BR, R, C, Pm and TV.(6.96)LVI2=RC(TV)2(Pm)2(BR)(60)2 By monitoring a wide range of subjects, we can determine the range of values of LVI2 for normal subjects, to distinctly diagnose subjects with disorders, such as obstructive lung disease (with increased value of Ra), emphysema (with enhanced value of Ca), and lung fibrosis (with decreased value of Ca). Now, it is possible that one lobe of the lung may be normal and the other lobe maybe diseased. For this purpose, in Section 6.12 we have developed a 2-lobe lung model, in terms of the response of their volumes to lung driving pressure. This 2-lobe lung model is formatted, by using our first-order differential equation model. The lung volume expression involves the compliance and flow-resistances of the two lobes. We have then demonstrated how this two-lobe lung volume model can be employed to evaluate the R and C parameters of the two lobes, to characterize the normality and diseased states of the two lobes separately. This is verily the crux of this expansive chapter on lung ventilation modeling and formulation of noninvasive lung ventilatory indices to properly distinguish diseased lungs.
更多查看译文
关键词
lung,modeling
AI 理解论文
溯源树
样例
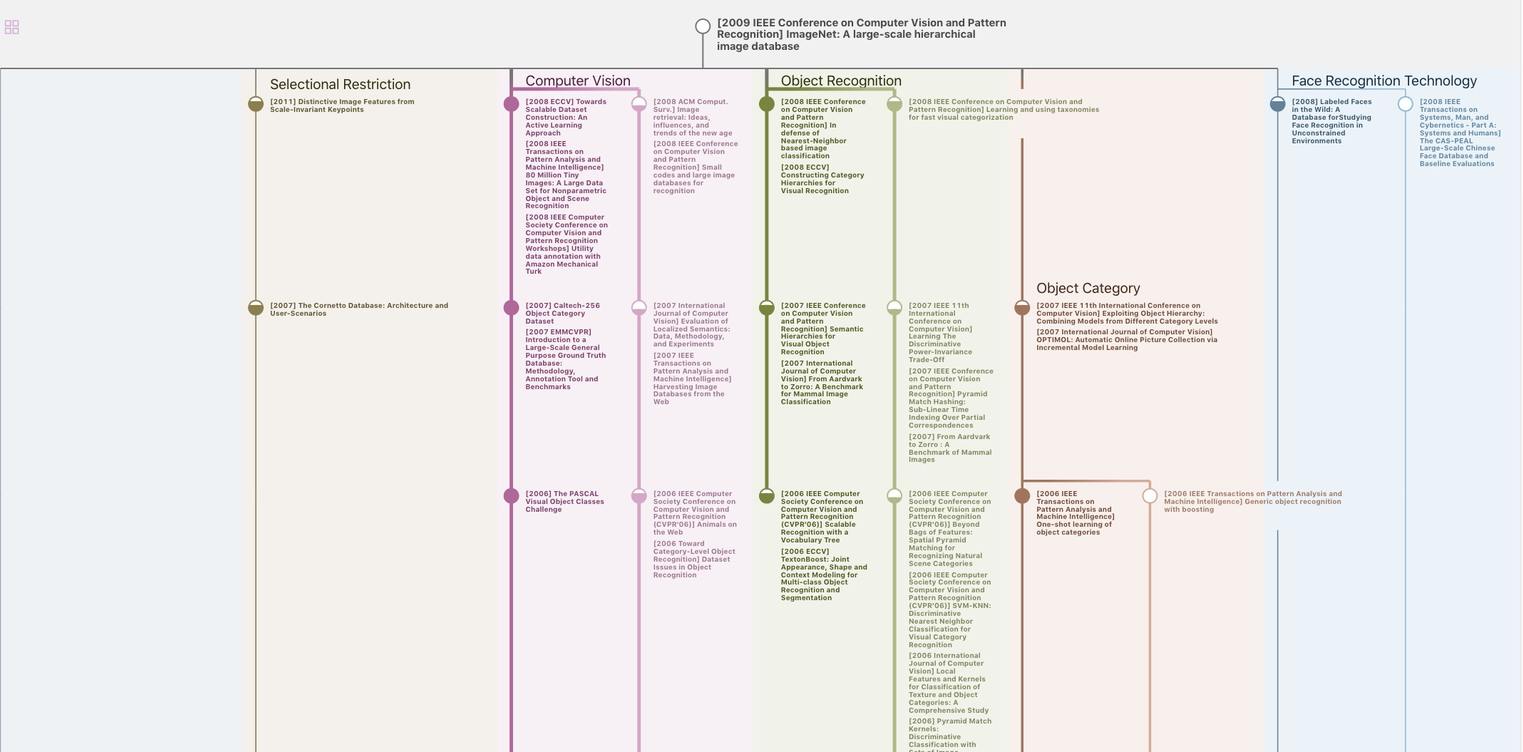
生成溯源树,研究论文发展脉络
Chat Paper
正在生成论文摘要