Identifying hypokalemia risk in hypertensive people: using machine learning in the united states national health and nutrition examination survey 1999-2018
Journal of Hypertension(2023)
摘要
Objective: Hypokalemia is a side-effect of diuretics. We aimed to use machine learning to identify features predicting hypokalemia risk in hypertensive patients. Design and method: Participants with hypertension in the United States National Health and Nutrition Examination Survey 1999-2018 were included for analysis. To choose the optimal algorithm, we tested five machine learning algorithms (Logistic Regression, k-Nearest Neighbor, Random Forest, Recursive Partitioning and Regression Trees, and eXtreme Gradient Boosting) with 38 screened features. We selected the most appropriate model according to the area under the receiver operating characteristic curve (AUROC). We then selected the key hypokalemia-associated features in the hypertension group and their cardiovascular diseases (CVD) subgroup using the SHapley Additive exPlanations (SHAP) values. Using SHAP values, the key features and their impact pattern on hypokalemia risk were determined. Results: 21,096 hypertensive participants, of whom 3,540 had known CVD, were included for analysis. The Random Forest algorithm had the highest AUROC (hypertension dataset: 0.73 [95%CI, 0.70-0.75], P<0.001; CVD subgroup: 0.76 [95%CI, 0.70-0.82], P<0.001) and sensitivity (hypertension dataset: 0.62; CVD subgroup: 0.72) based on 38 features screened. Moreover, the nomogram based on the top fifteen key features screened by random forest retained good performance (hypertension dataset: 0.73 [95%CI, 0.70-0.75], P<0.001; CVD subgroup: 0.74 [95%CI, 0.68-0.81], P<0.001): gender, race, age, poverty income ratio, smoking status, body mass index, systolic and diastolic blood pressure, non-potassium-sparing diuretics use and duration, duration of renin-angiotensin blockers, potassium-sparing diuretics use, polypharmacy, and comorbid arthritis and diabetes in hypertension dataset; while in CVD subgroup, renin-angiotensin blockers use replaced potassium-sparing diuretics use. Conclusions: Our predictive model shows good performance, and hypokalemia-associated key features have been identified in hypertensive patients and the subgroup with CVD. These findings from machine learning facilitate the development of artificial intelligence to highlight hypokalemia risk in hypertension patients.
更多查看译文
关键词
hypertensive people,nutrition examination,machine learning
AI 理解论文
溯源树
样例
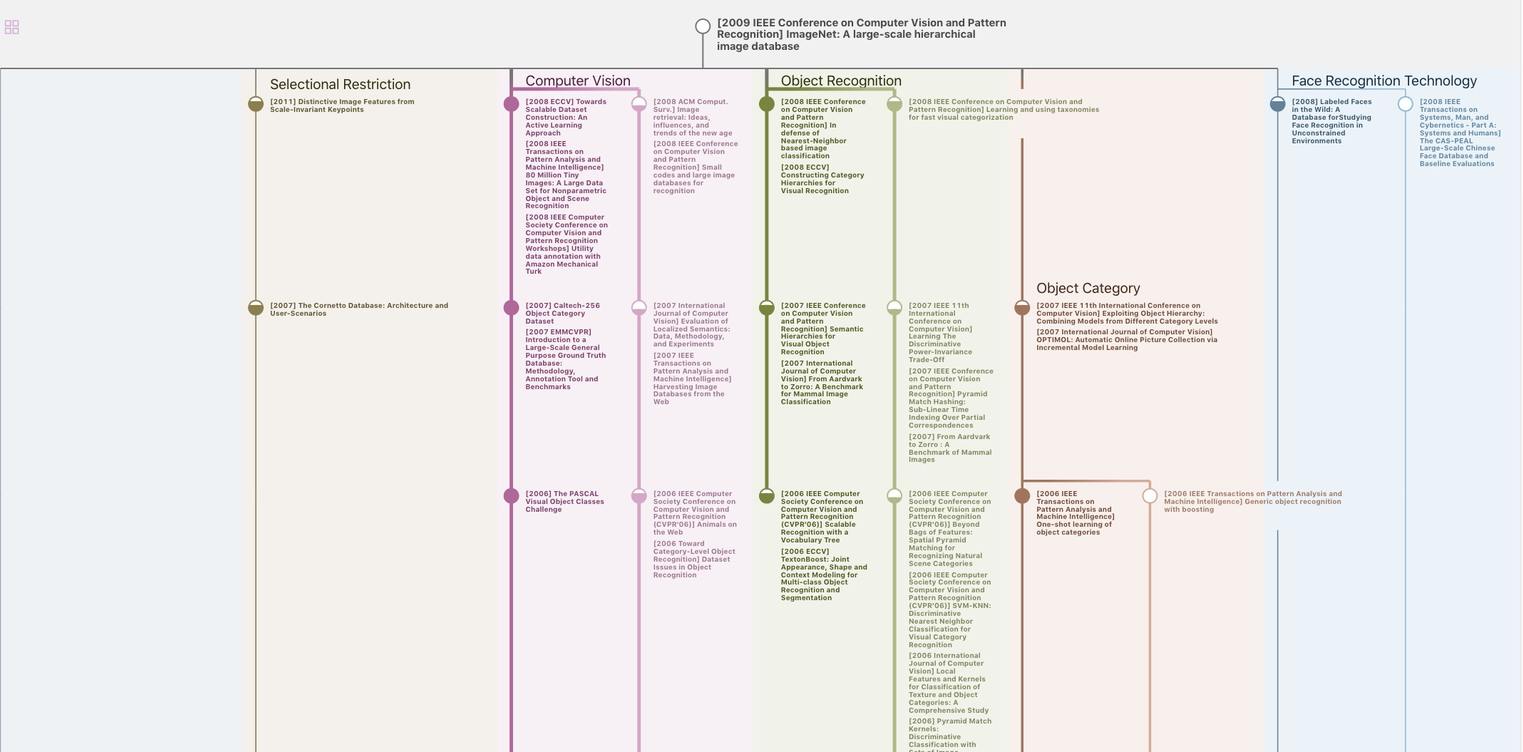
生成溯源树,研究论文发展脉络
Chat Paper
正在生成论文摘要