Molecule Design by Latent Space Energy-Based Modeling and Gradual Distribution Shifting
arXiv (Cornell University)(2023)
摘要
Generation of molecules with desired chemical and biological properties such as high drug-likeness, high binding affinity to target proteins, is critical for drug discovery. In this paper, we propose a probabilistic generative model to capture the joint distribution of molecules and their properties. Our model assumes an energy-based model (EBM) in the latent space. Conditional on the latent vector, the molecule and its properties are modeled by a molecule generation model and a property regression model respectively. To search for molecules with desired properties, we propose a sampling with gradual distribution shifting (SGDS) algorithm, so that after learning the model initially on the training data of existing molecules and their properties, the proposed algorithm gradually shifts the model distribution towards the region supported by molecules with desired values of properties. Our experiments show that our method achieves very strong performances on various molecule design tasks.
更多查看译文
关键词
energy-based
AI 理解论文
溯源树
样例
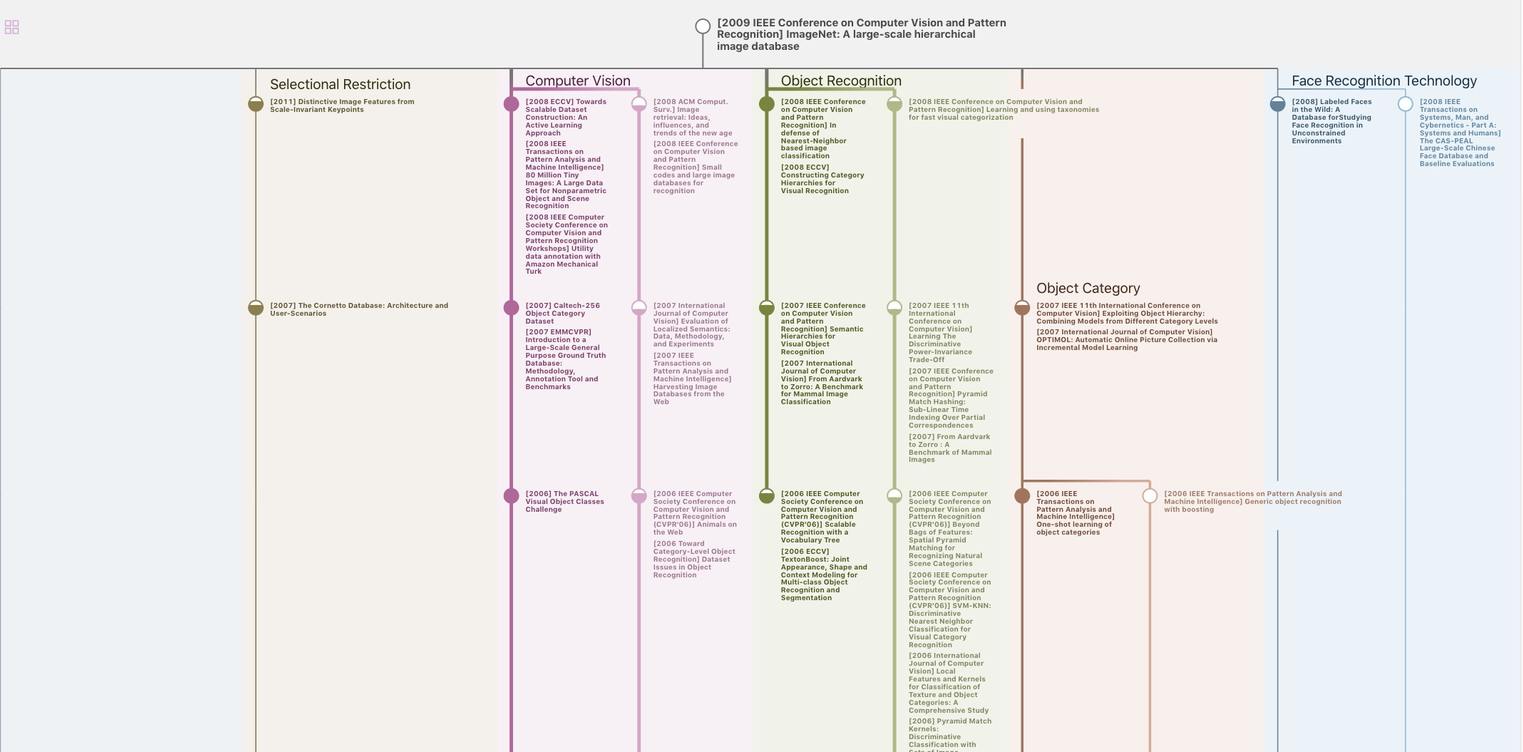
生成溯源树,研究论文发展脉络
Chat Paper
正在生成论文摘要