Prediction of Spherical Equivalent Refraction and Annual Myopia Progression Rate in Chinese Schoolchildren Based on Random Forest Model
Research Square (Research Square)(2023)
摘要
Abstract Purpose To establish a machine learning (ML) model for predicting future spherical equivalent refraction (SER) and its annual progression rate in Chinese schoolchildren. Methods A total of 23,832 participants were analyzed, including 12,514 females and 11,318 males. The follow-up visits were conducted between February 2008 and June 2021. The SER value at a specific future time point was predicted based on the results of at least two cycloplegic refraction measurements. The prediction model was established using a random forest (RF) model. A five-fold cross-validation was used to select the parameters and train an optimal RF model for prediction. Axial position, SER values, and age were selected as predictors. The performance of the algorithm was evaluated using the coefficient of determination (R 2 ), mean squared error (MSE), and mean absolute error (MAE). Results The RF algorithm had a great advantage in the detection of high myopia, with an R 2 value of 0.944 in the training datasets. The established model achieved clinically acceptable predictions of SER values at a specific future time point. For the performance of the algorithm, the R 2 achieved 0.994 in the training datasets, and 0.963 in the validation datasets, respectively. For the testing datasets, the MAE, MSE, and R 2 achieved 0.489, 0.502, and 0.964 for the entire population, respectively. Conclusions Our ML model performs well in the prediction of SER and myopia progression in Chinese schoolchildren. Random forest algorithm has potential advantages in the detection of high myopia and thus provides evidence for precise individual intervention and control of myopia.
更多查看译文
关键词
annual myopia progression rate,spherical equivalent refraction
AI 理解论文
溯源树
样例
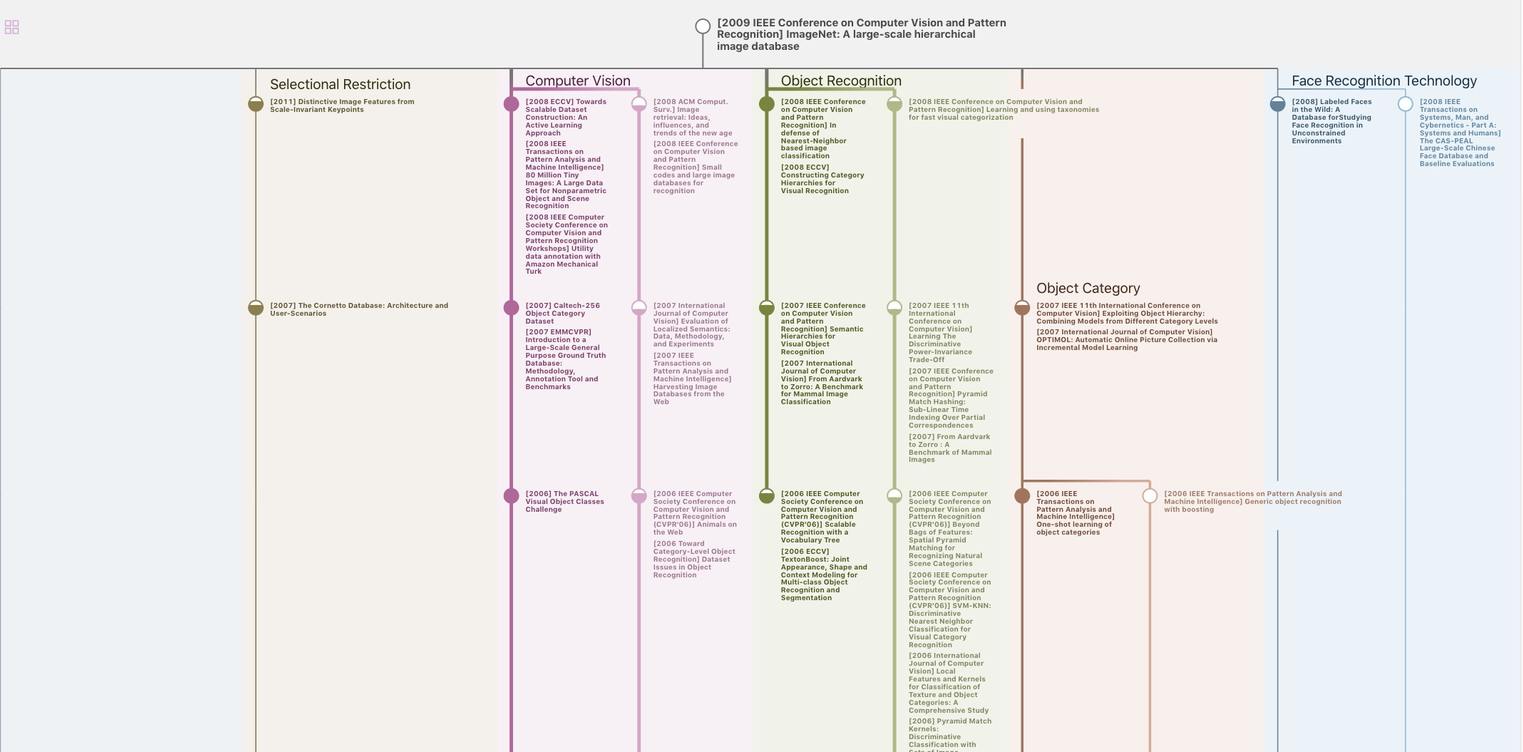
生成溯源树,研究论文发展脉络
Chat Paper
正在生成论文摘要