A framework for the metrification of input image quality in deep networks
IS&T International Symposium on Electronic Imaging Science and Technology(2023)
摘要
Deep Neural Networks (DNNs) are critical for real-time imaging applications including autonomous vehicles. DNNs are often trained and validated with images that originate from a limited number of cameras, each of which has its own hardware and image signal processing (ISP) characteristics. However, in most real-time embedded systems, the input images come from a variety of cameras with different ISP pipelines, and often include perturbations due to a variety of scene conditions. Data augmentation methods are commonly exploited to enhance the robustness of such systems. Alternatively, methods are employed to detect input images that are unfamiliar to the trained networks, including out of distribution detection. Despite these efforts DNNs remain widely systems with operational boundaries that cannot be easily defined. One reason is that, while training and benchmark image datasets include samples with a variety of perturbations, there is a lack of research in the areas of metrification of input image quality suitable to DNNs and a universal method to relate quality to DNN performance using meaningful quality metrics. This paper addresses this lack of metrification specific to DNNs systems and introduces a framework that uses systematic modification of image quality attributes and relate input image quality to DNN performance.
更多查看译文
关键词
input image quality,deep networks,metrification
AI 理解论文
溯源树
样例
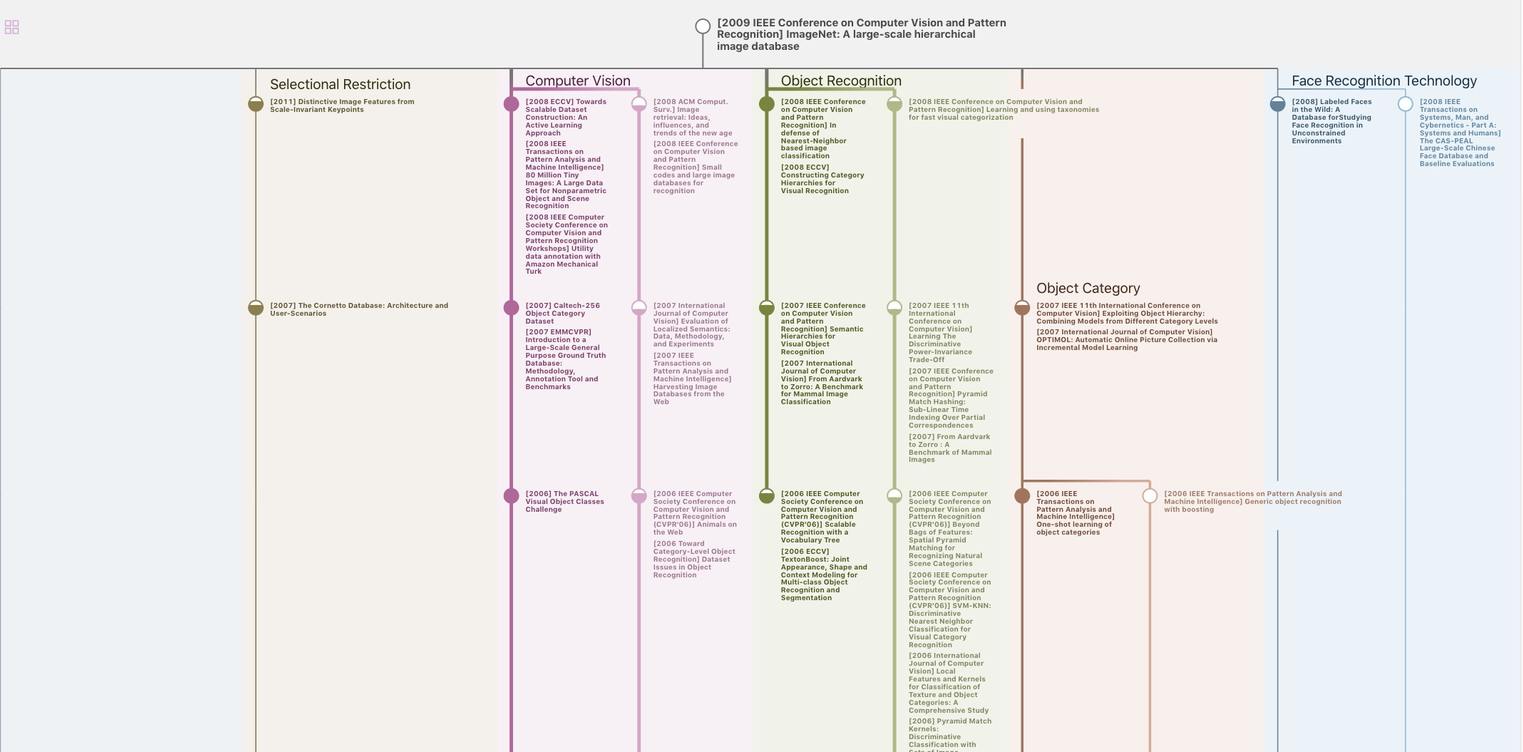
生成溯源树,研究论文发展脉络
Chat Paper
正在生成论文摘要