SERNA: A Stacking-Based Classification Algorithm for Early Prediction of ICU Needs for COVID-19 Patients
Research Square (Research Square)(2023)
摘要
Abstract The COVID-19 pandemic exerted an unprecedented strain on healthcare systems, with millions of hospitalizations and intensive care unit (ICU) admissions. The overwhelming demand for ICU beds necessitates the efficient allocation of resources and early prediction of patients’ ICU needs. In this paper, we introduce SERNA (Stacked Ensemble using Regional and Neighborhood Assessment), a novel stacking-based classification algorithm that predicts ICU needs within the first 2 hours of patient admission. The SERNA algorithm employs novel procedures to create new feature sets, establishing connections between data point locations and learners’ local performances. These generated features are then fed into a meta-learner, which strategically assigns higher weights to strong learners and lower weights to weaker ones. We evaluated the proposed algorithm using COVID-19 ICU admission data, comparing its performance against various baseline models. Remarkably, the SERNA algorithm achieved a recall of 90% and an AUC of 80%, surpassing all baseline models. It exhibited an improvement in accuracy by 3%, precision by 1%, recall by 14%, F1 score by 6%, and AUC by 4%. Notably, these results were achieved by utilizing data only from the first 2 hours after admission, enabling a crucial reduction in reaction time of 10–22 hours compared to previous studies.
更多查看译文
关键词
icu needs,early prediction,stacking-based
AI 理解论文
溯源树
样例
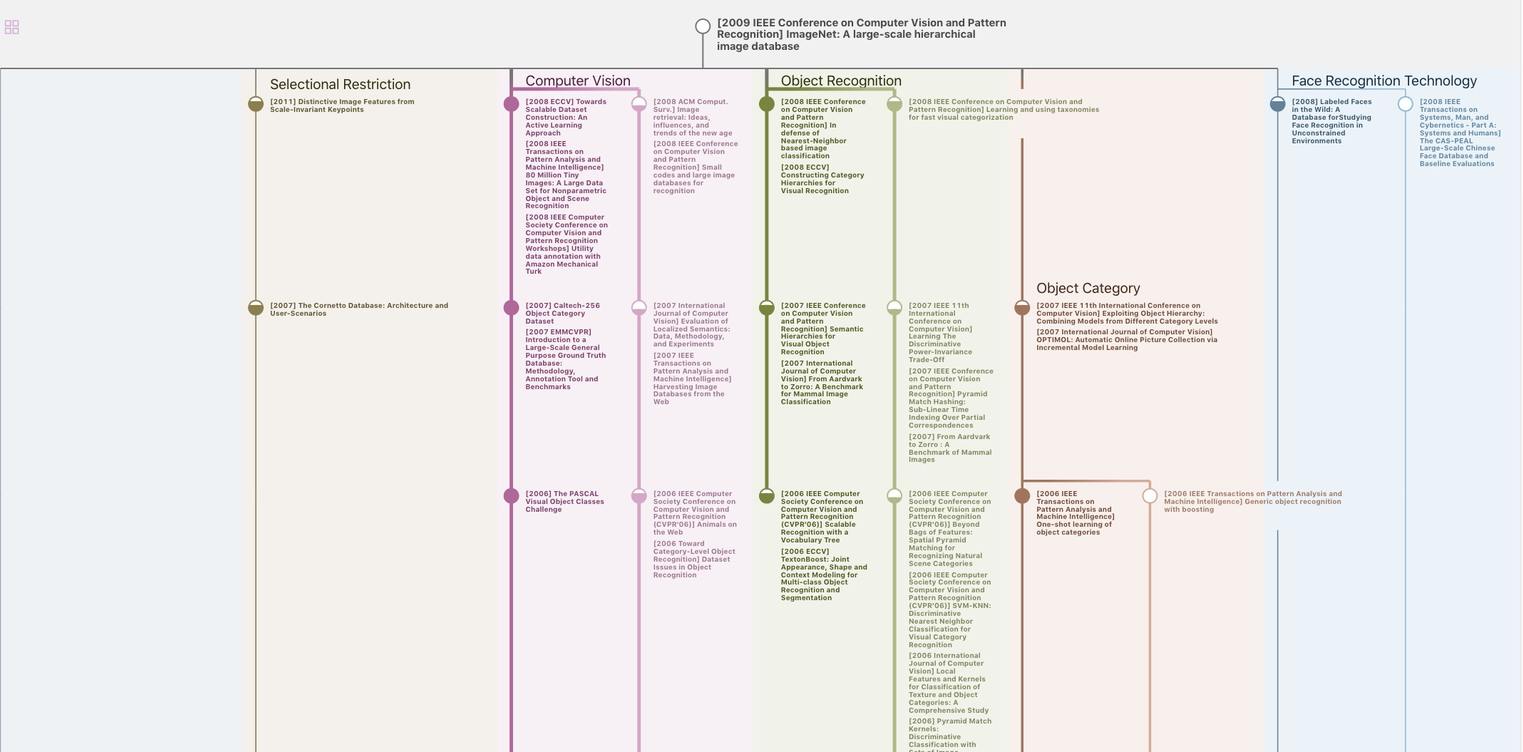
生成溯源树,研究论文发展脉络
Chat Paper
正在生成论文摘要