RNA-seq Parent-of-Origin Classification with Machine Learning applied to Alignment Features
Research Square (Research Square)(2023)
摘要
Abstract Background Parent-of-origin allele-specific gene expression (ASE) can be detected in interspecies hybrids by virtue of RNA sequence variants between the parental haplotypes. ASE is detectable by differential expression analysis (DEA) applied to the counts of RNA-seq read pairs aligned to parental references, but aligners do not always choose the correct parental reference. Results We used public data from four species pairs that are known to hybridize. For each pair, we obtained RNA-seq read pairs from both species and measured our ability to assign each read to its proper species by comparing reads to the transcriptome or genome references. We tested four software packages that assign each read pair to a reference position and found that they often favored the incorrect species reference. To address this problem, we introduce a post process that extracts alignment features and trains a random forest classifier to choose the better alignment. On each dataset tested, our machine-learning post-processor achieved higher accuracy than the aligner by itself at choosing the correct species per RNA-seq read pair. Conclusions For the parent-of-origin classification of RNA-seq, machine learning can improve the accuracy of alignment-based methods. This approach should be useful for enhancing ASE detection in interspecies hybrids. We believe this is the first application of machine learning to this problem domain.
更多查看译文
关键词
alignment,machine learning,classification,rna-seq,parent-of-origin
AI 理解论文
溯源树
样例
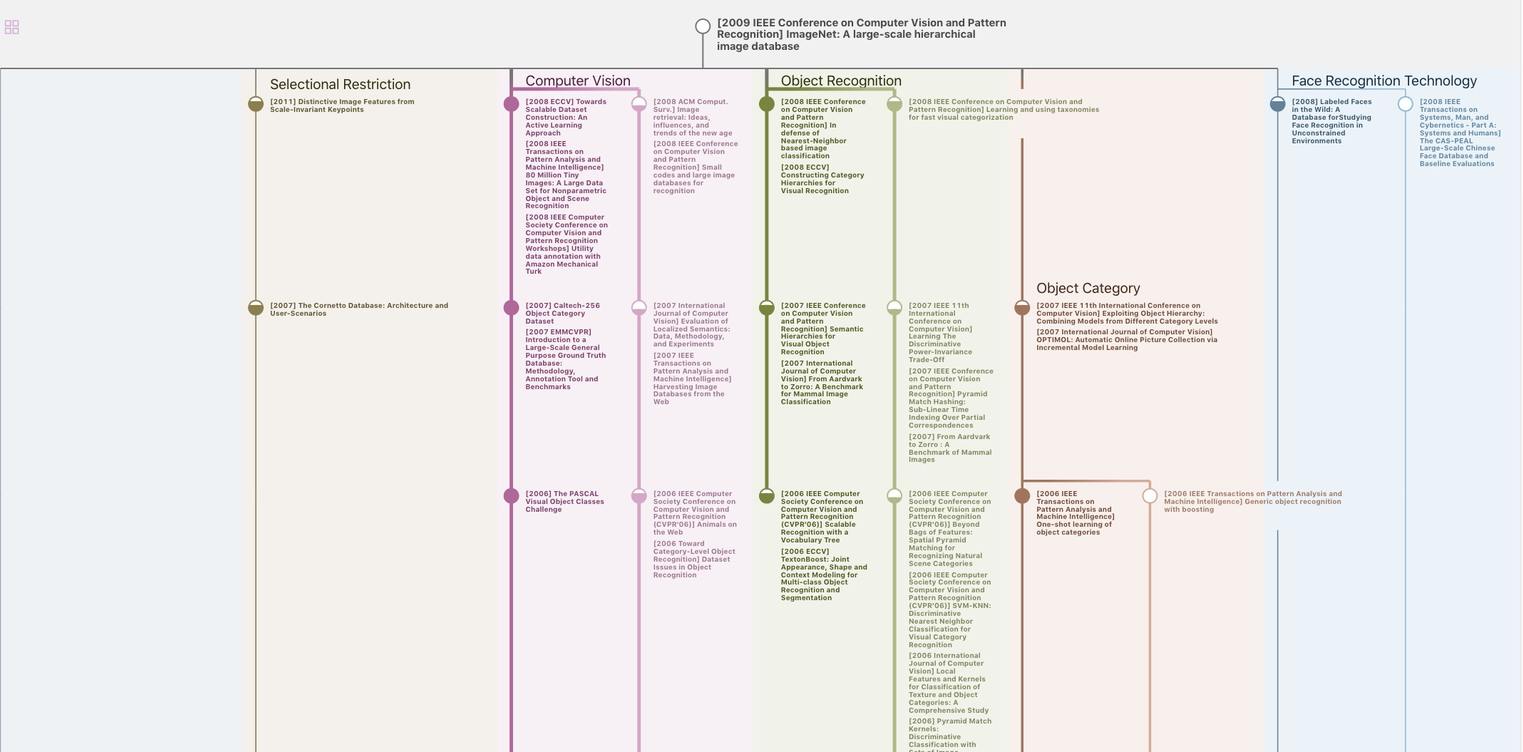
生成溯源树,研究论文发展脉络
Chat Paper
正在生成论文摘要