Evaluating YOLO-based Object Detectors for Detecting Road-Killed Endangered Brazilian Animals
Research Square (Research Square)(2023)
摘要
Abstract Wildlife roadkill is a recurring, dangerous problem that affects both humans and animals and has received increasing attention from environmentalists worldwide. Addressing this problem is difficult due to the high investments required in road infrastructure to effectively reduce wildlife vehicle collisions. Despite recent applications of machine learning techniques in low-cost and economically viable detection systems, e.g., for alerting drivers about the presence of animals and collecting statistics on endangered animal species, the success and wide adoption of these systems depend heavily on the availability of data for system training. The lack of training data negatively impacts the feature extraction of machine learning models, which is crucial for successful animal detection and classification. In this paper, we evaluate the performance of several state-of-the-art object detection models on limited data for model training. The selected models are based on the YOLO architecture, which is well-suited for and commonly used in real-time object detection. These include the YoloV4, Scaled-YoloV4, YoloV5, YoloR, YoloX, and YoloV7 models. We focus on Brazilian endangered animal species and use the BRA-Dataset for model training. We also assess the effectiveness of data augmentation and transfer learning techniques in our evaluation. The models are compared using summary metrics such as precision, recall, mAP, and FPS and are qualitatively analyzed considering classic computer vision problems. The results show that the architecture with the best results against false negatives is Scaled-YoloV4, while the best FPS detection score is the nano version of YoloV5.
更多查看译文
关键词
object detectors,brazilian animals,yolo-based,road-killed
AI 理解论文
溯源树
样例
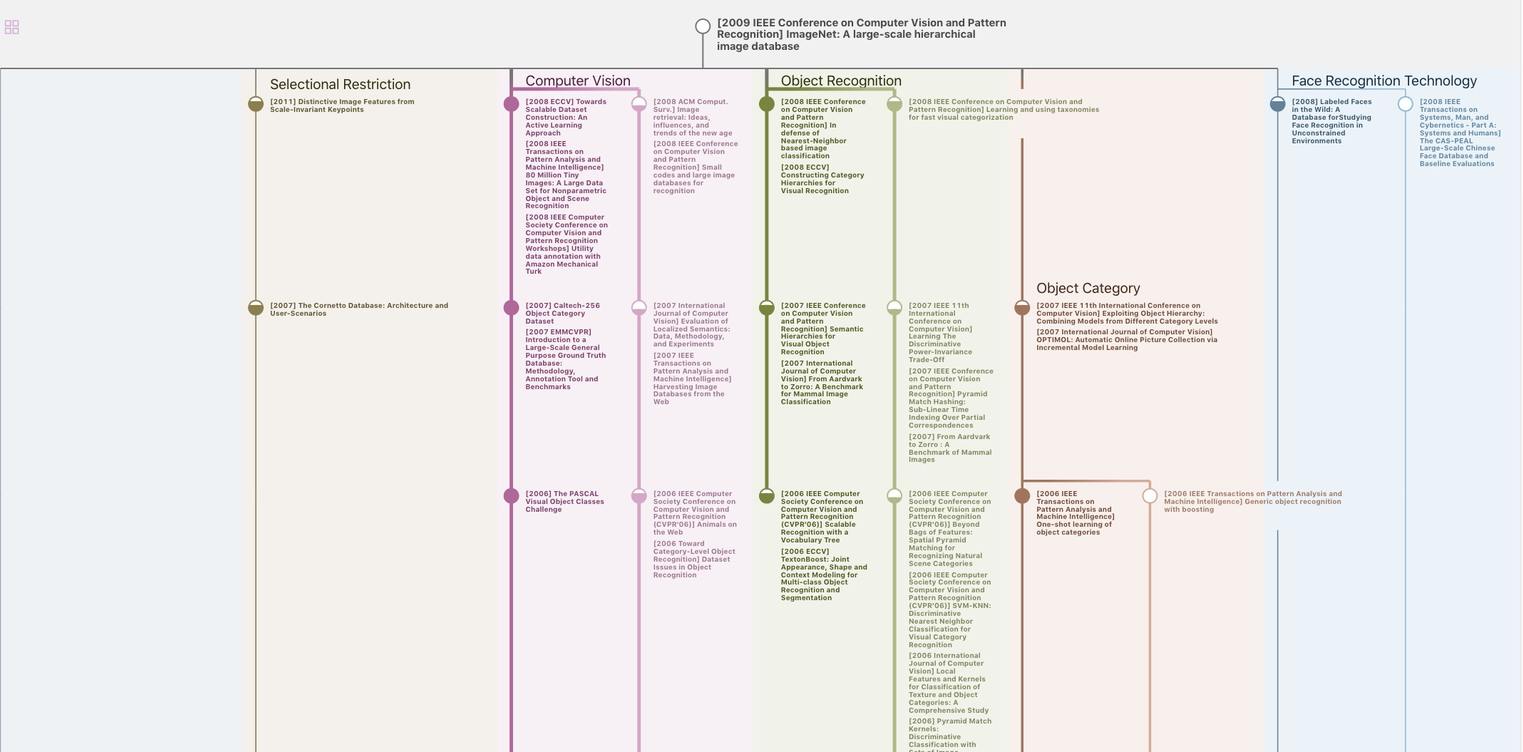
生成溯源树,研究论文发展脉络
Chat Paper
正在生成论文摘要