Asynchronous Multithreading Reinforcement Control Decision Method for Unmanned Surface Vessel
IEEE INTERNET OF THINGS JOURNAL(2023)
摘要
Unmanned surface vehicles (USVs) serve as crucial data acquisition nodes within the ocean observation network, enabling the collection of various types of data from the ocean-atmosphere interface and below the sea surface. However, USVs face environmental challenges, such as tides, ocean currents, and winds, in complex marine environments. These factors introduce uncertainty into task decision-making and motion control, significantly affecting the real-time operation, stability, and effectiveness of the USV system. This article proposes an asynchronous multithreading reinforcement control decision method to address these issues for USVs. By establishing a task environment model, this method captures and analyzes the dynamic characteristics of the marine environment while decomposing the tasks accordingly. Based on this, the method enables collaborative control for path planning, emergency collision avoidance, and path tracking by mapping the state space of each task to the action space of the USV using a deep neural network. To improve the real-time performance of this method, experience replay is integrated with the target network technology, which strengthens the proximal policy optimization algorithm under the asynchronous multithreading framework. To enhance the method's accuracy, effective reward functions are designed based on task objectives and constraints. These functions evaluate the strategies generated by the deep neural network, ensuring that the USV always adopts optimal strategies. The simulation results validate the effectiveness and superiority of the proposed method, highlighting its substantial potential for engineering applications.
更多查看译文
关键词
Asynchronous multithreading,control decision,proximal policy optimization (PPO),unmanned surface vehicle (USV)
AI 理解论文
溯源树
样例
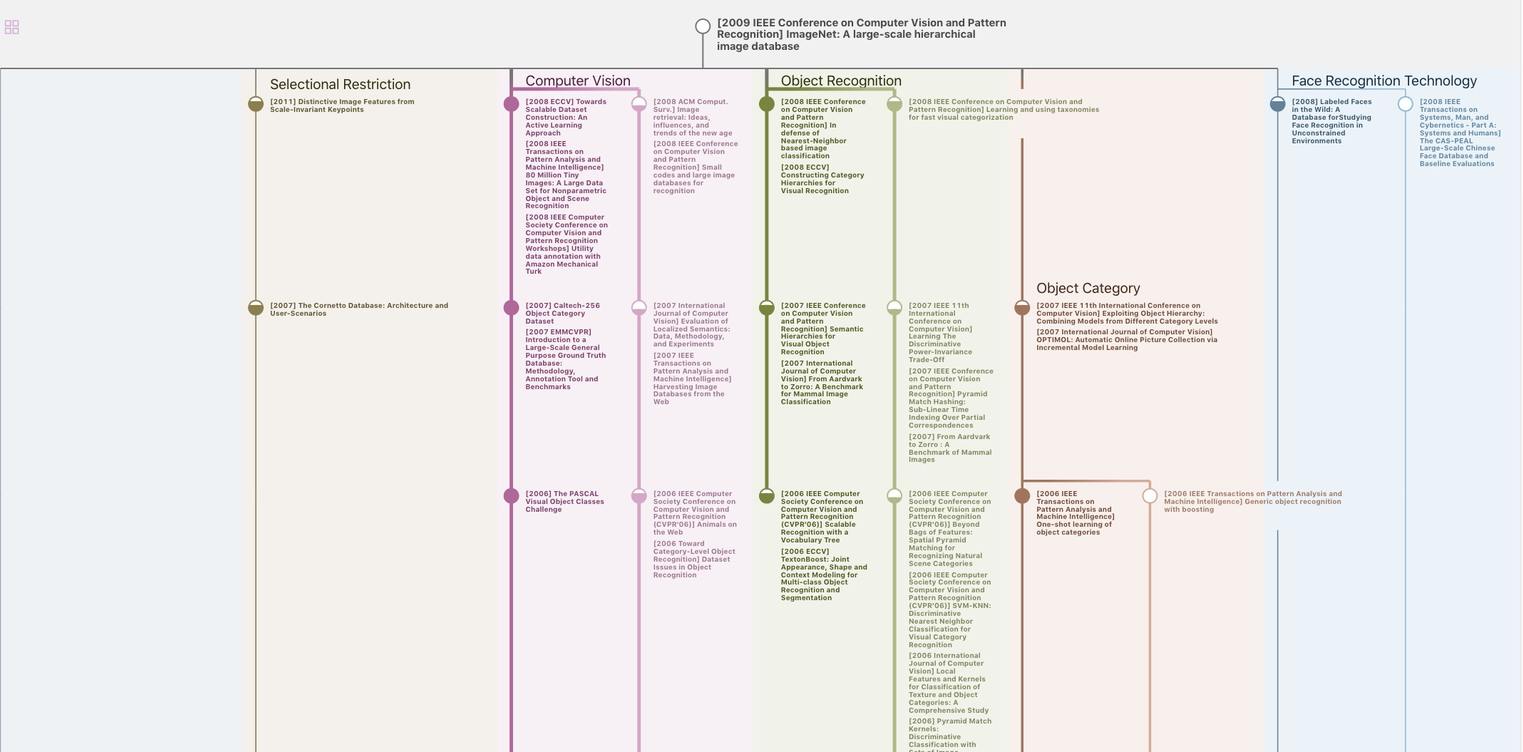
生成溯源树,研究论文发展脉络
Chat Paper
正在生成论文摘要