Hybrid Framework for Genomic Data Classification Using Deep Learning: QDeep_SVM
Algorithms for intelligent systems(2023)
摘要
Background: In the analysis of genomic data, feature analysis is a crucial step before classification. Most of the feature filtering methods are based on the ranking score of the standalone machine learning models. Due to the curse of dimensionality, the classification of the features with the ranking methods affects over highly expressed genes. Methodology: The Quantum-Inspired Immune Clone Optimization method reduces the feature vector by grouping the genes that are closely bounded. It filters the optimized features close to the interested gene population. In addition, the gene filtering methods and feature relevance can improve the classification system's accuracy. Once the essential features have been established, a hybrid “Deep Convolutional Neural Network (DCNN) with Support Vector Machine (SVM)” is used to classify the data. The proposed model efficiently filters noise and gene groups in normal and tumour benchmarking datasets with reduced processing time and improved accuracy. The results of numerical tests on RNA-Seq gene expression datasets show that our proposed technique outperforms existing classification methods. Additionally, the features selected by the hybrid model are reviewed for relevance to the gene ontology terms. Furthermore, due to the efficiency of the RBF kernel, DCNN–SVM has the best classifier performance (80.45 per cent) among the standalone methods. We suggested DCNN and SVM combination improves the DCNN, SVM, and RF separately. Our results on large RNA-Seq gene expression datasets suggest that DCNN–SVM is the optimum classification method.
更多查看译文
关键词
genomic data classification,qdeep_svm,deep learning
AI 理解论文
溯源树
样例
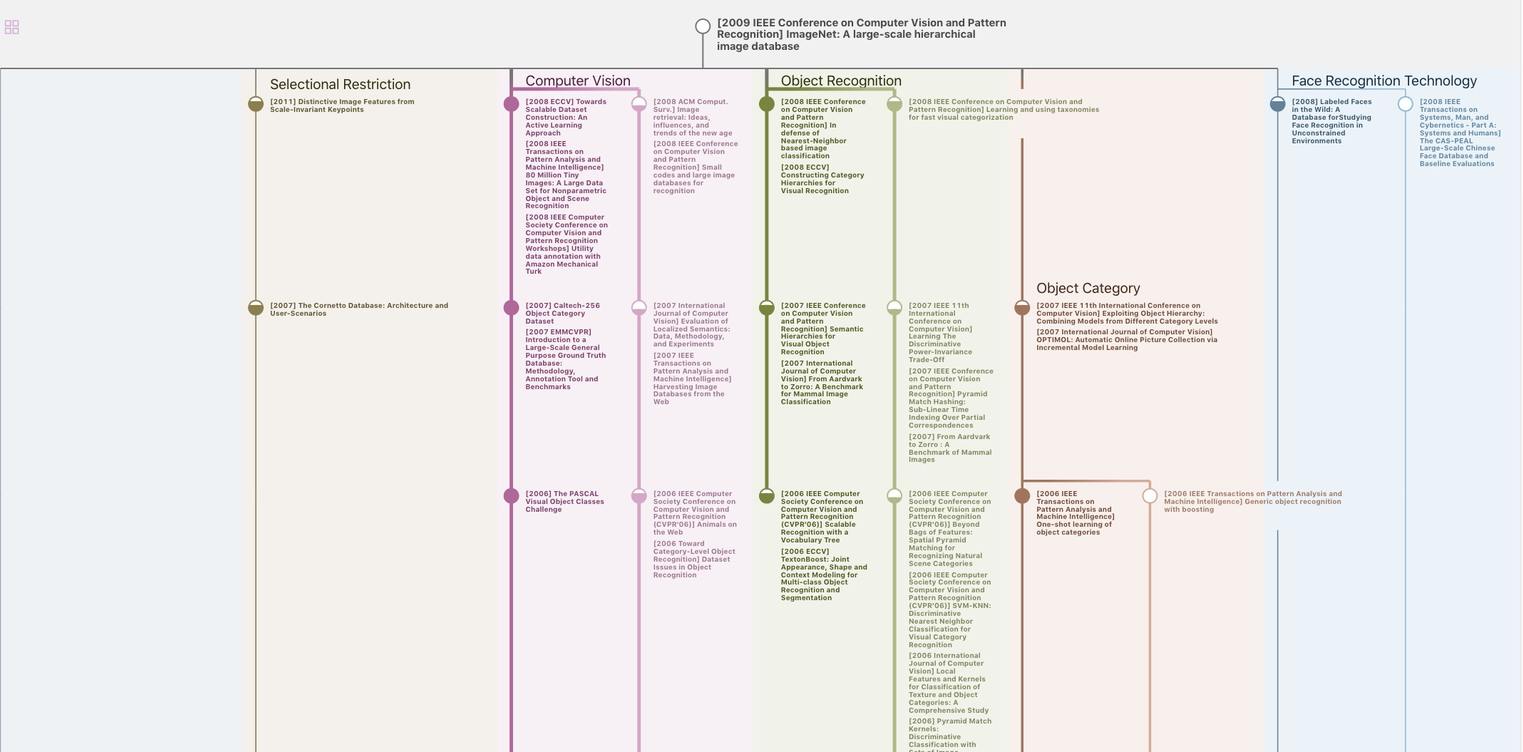
生成溯源树,研究论文发展脉络
Chat Paper
正在生成论文摘要