Road Side Unit-Assisted Learning-Based Partial Task Offloading for Vehicular Edge Computing System.
IEEE Trans. Veh. Technol.(2024)
摘要
The rapid development of vehicular networks creates diverse ultra-low latency constrained and computation-intensive applications, which bring challenges to both communication and computation capabilities of the vehicles and their transmission. By offloading tasks to the edge servers or vehicles in the neighbourhood, vehicular edge computing (VEC) provides a cost-efficient solution to this problem. However, the channel state information and network structure in the vehicular network varies fast because of the inherent mobility of vehicle nodes, which brings an extra challenge to task offloading. To address this challenge, we formulate the task offloading in vehicular network as a multi-armed bandit (MAB) problem and propose a novel road side unit (RSU)-assisted learning-based partial task offloading (RALPTO) algorithm. The algorithm enables vehicle nodes to learn the delay performance of the service provider while offloading tasks. Specifically, the RSU could assist the learning process by sharing the learning information among vehicle nodes, which improves the adaptability of the algorithm to the time-varying networks. Simulation results demonstrate that the proposed algorithm achieves lower delay and better learning performance compared with the benchmark algorithms.
更多查看译文
关键词
Multi-armed bandit,online learning,task offloading,vehicular edge computing,
AI 理解论文
溯源树
样例
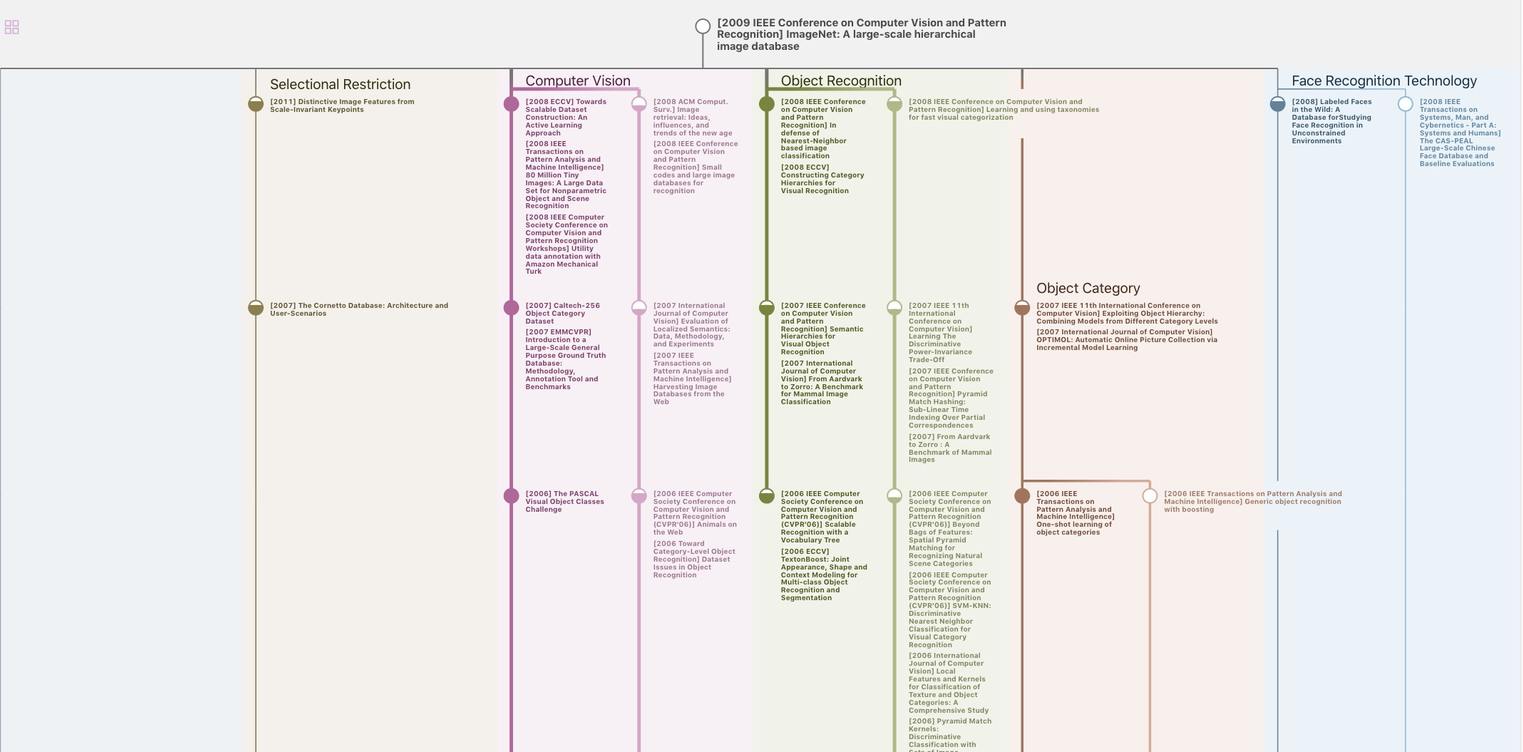
生成溯源树,研究论文发展脉络
Chat Paper
正在生成论文摘要