OYOLO: An Optimized YOLO Method for Complex Objects in Remote Sensing Image Detection
IEEE Geoscience and Remote Sensing Letters(2023)
摘要
There have been significant advancements in deep learning based object detection algorithms, which have found widespread applications across various fields, including remote sensing. However, existing algorithms often fall short in detecting complex objects in remote sensing images, resulting in suboptimal overall performance. To address this issue, this letter proposes a novel object detection algorithm called OYOLO, which builds upon the YOLOv4 network and incorporates several optimization techniques. Firstly, a novel feature enhancement network is designed to better learn the contextual information and the object feature. Specifically, this section introduces Adaptive Spatial Feature Fusion and proposes an optimized Spatial Pyramid Pooling method,
i.e
., SPPCPC. Furthermore, the Effective Intersection over Union loss function is introduced to refine the bounding box regression, thereby minimizing the interference of non-essential features. Lastly, this letter proposes an improved backbone network,
i.e
., DSCDarknet53 to enhance the detection speed of the model. Verified through experiments, OYOLO demonstrates an increase of 2.1% and 1.6% in mean Average Precision (mAP) values on the TGRS-HRRSD and RSOD datasets, respectively, compared to the original YOLOv4 algorithm. The detection speeds of the two datasets are also enhanced by 5.3 Frame Per Second (FPS) and 5.3 FPS, respectively. Specifically, OYOLO exhibits remarkable improvements on a dataset with complex objects, with an mAP gain of 7.9%. Moreover, experimental results demonstrate that OYOLO outperforms other YOLO methods.
更多查看译文
关键词
remote sensing images,complex object,YOLO,optimization,detection
AI 理解论文
溯源树
样例
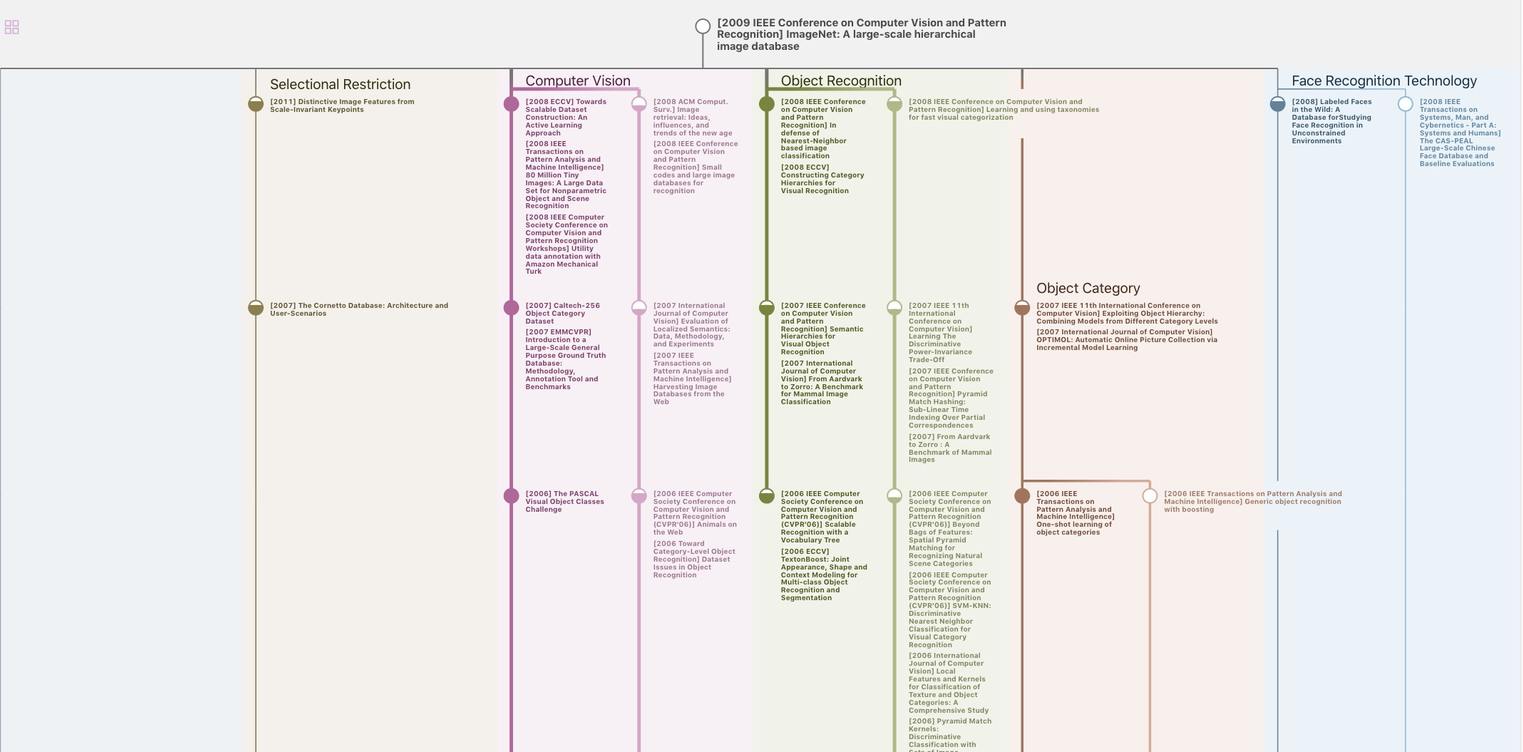
生成溯源树,研究论文发展脉络
Chat Paper
正在生成论文摘要