Abstract 4321: Clinical-grade early detection of esophageal cancer on data from a non-endoscopic device using deep learning
Cancer Research(2023)
摘要
Abstract Aim: Our goal was to evaluate a novel deep learning system for integrated human-AI review of whole-slide images (WSIs) from the Cytosponge to predict progression from Barrett's esophagus (BE) to esophageal adenocarcinoma (EAC). Background: Fewer than 1% of BE patients advance to EAC, therefore delineating the patients at elevated risk is crucial for prioritizing their surveillance and treatment. Previous work has shown that the Cytosponge, a non-endoscopic test, coupled with biomarkers (TFF3, atypia, p53) can be used to identify and risk-stratify individuals with BE. However, the Cytosponge workflow to date has required a time consuming pathology review. Methods: We collected 1,700 BE Cytosponge WSIs from over 800 patients. We hand-drew over 30,000 digital annotations on the WSIs, a 300-hour collaborative effort between a computer scientist and 4 pathologists. Using these data for training, validation, and testing, we developed a deep learning system to determine whether cellular atypia and/or p53 overexpression is present. We used this system in a machine-assisted (MA) review process to highlight the most diagnostically interesting regions of WSIs for pathologist review. In our proposed system, patients are automatically triaged into high or low confidence positive, and high confidence negative classes for both atypia and p53 status, and only low confidence cases are sent for MA review. Results: Out of 23 architectures, the best atypia model achieves an accuracy of 0.90, and the best p53 model 0.96. The model was concordant with pathologists in 342/425 (80.5%) validation and 301/330 (91.2%) test-set patients. The system also identified 83 validation cases with atypia and/or p53 that were not previously reported by the pathologists. Of these, 29 (35%) were confirmed abnormal with the ground truth endoscopy biopsies (11 high grade dysplasia or intramucosal carcinoma, 14 low grade dysplasia, 5 indefinite for dysplasia). In the test set 29 results were discordant with pathology including 6 (21%) confirmed abnormal on endoscopy biopsies (3 HGD/IMC, 2 LGD, 1 IND). We used the models’ outputs to localize where these “missed” regions lie in the WSIs, and pathologist MA review confirmed the presence of focal abnormalities (cellular atypia +/- p53 aberrance) correctly identified by the algorithm. If pathologists only review cases for which the model had low confidence, this preserves overall performance while reducing pathologist workload over twenty-fold. MA review on the remaining WSIs is 4.7 times faster than standard review for atypia screening and 16.0 times faster for p53. Conclusion: This work shows the potential of an integrated human-AI WSI review process to drastically expedite a time consuming task and improve on pathologist performance when abnormal regions are easy for human eyes to miss. This work therefore lays the groundwork for similar machine-doctor approaches in other areas of medicine. Citation Format: Adam G. Berman, Ahmad Miremadi, Maria O'Donovan, Shalini Malhotra, Monika Tripathi, Rebecca C. Fitzgerald, Florian Markowetz. Clinical-grade early detection of esophageal cancer on data from a non-endoscopic device using deep learning. [abstract]. In: Proceedings of the American Association for Cancer Research Annual Meeting 2023; Part 1 (Regular and Invited Abstracts); 2023 Apr 14-19; Orlando, FL. Philadelphia (PA): AACR; Cancer Res 2023;83(7_Suppl):Abstract nr 4321.
更多查看译文
关键词
esophageal cancer,deep learning,early detection,clinical-grade,non-endoscopic
AI 理解论文
溯源树
样例
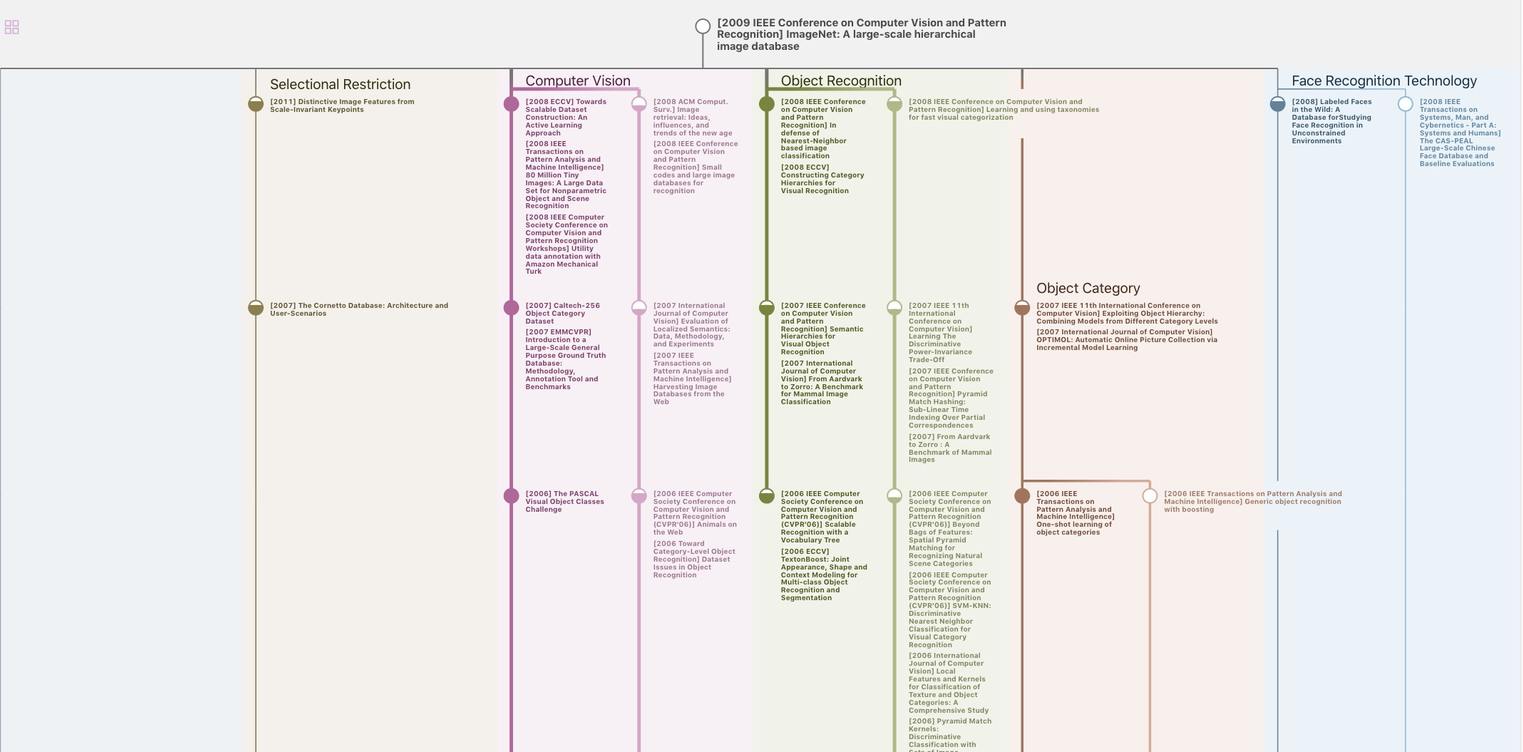
生成溯源树,研究论文发展脉络
Chat Paper
正在生成论文摘要