Domain independent post-processing with graph U-nets: Applications to Electrical Impedance Tomographic Imaging
arXiv (Cornell University)(2023)
摘要
Reconstruction of tomographic images from boundary measurements requires flexibility with respect to target domains. For instance, when the system equations are modeled by partial differential equations the reconstruction is usually done on finite element (FE) meshes, allowing for flexible geometries. Thus, any processing of the obtained reconstructions should be ideally done on the FE mesh as well. For this purpose, we extend the hugely successful U-Net architecture that is limited to rectangular pixel or voxel domains to an equivalent that works flexibly on FE meshes. To achieve this, the FE mesh is converted into a graph and we formulate a graph U-Net with a new cluster pooling and unpooling on the graph that mimics the classic neighborhood based max-pooling. We demonstrate effectiveness and flexibility of the graph U-Net for improving reconstructions from electrical impedance tomographic (EIT) measurements, a nonlinear and highly ill-posed inverse problem. The performance is evaluated for simulated data and from three measurement devices with different measurement geometries and instrumentations. We successfully show that such networks can be trained with a simple two-dimensional simulated training set and generalize to very different domains, including measurements from a three-dimensional device and subsequent 3D reconstructions.
更多查看译文
关键词
electrical impedance tomographic imaging,post-processing,u-nets
AI 理解论文
溯源树
样例
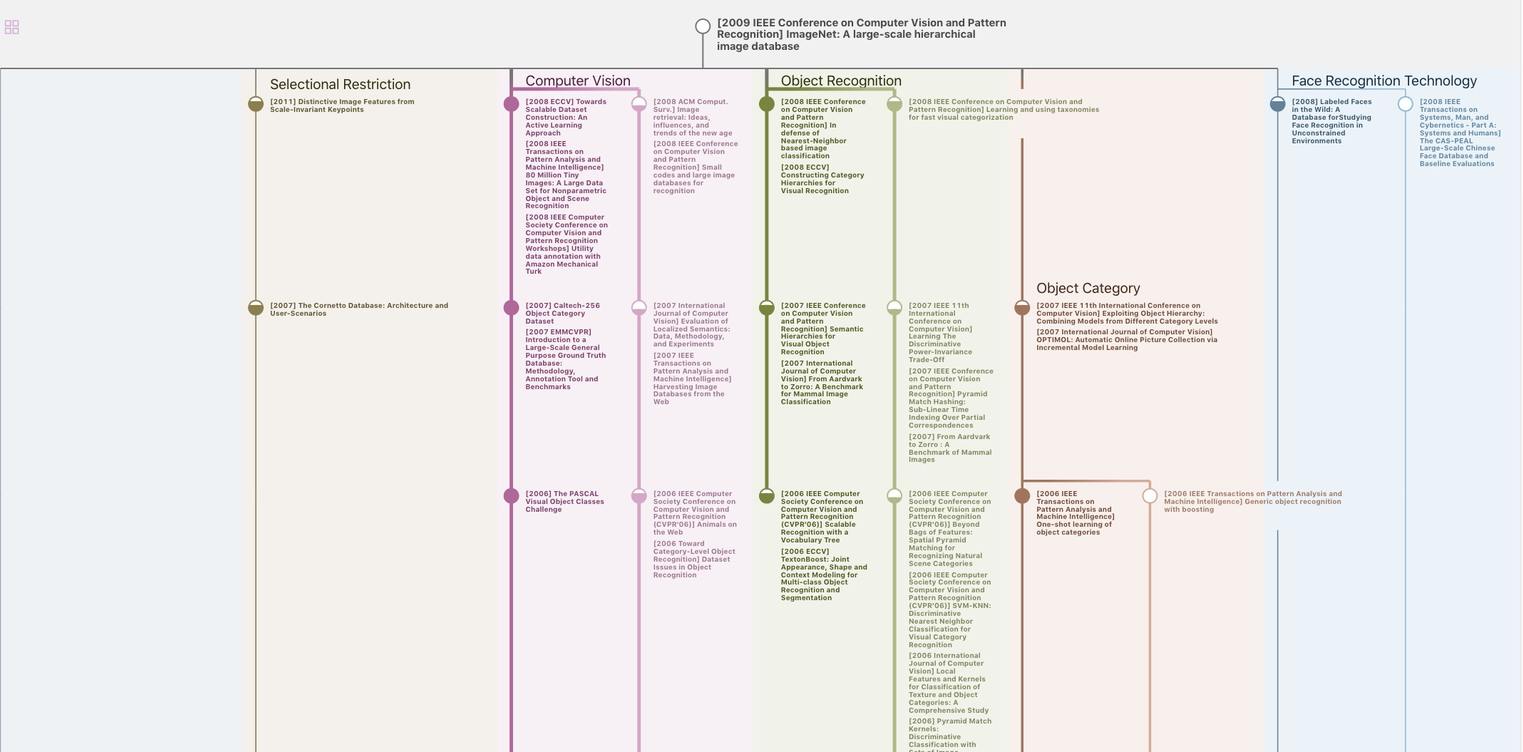
生成溯源树,研究论文发展脉络
Chat Paper
正在生成论文摘要