Compensation of Disturbance Induced Estimation Bias in Adaptive Dynamic Programming Based Optimal Control
Society for Industrial and Applied Mathematics eBooks(2023)
摘要
In this paper, we present a bias compensating adaptive dynamic programming (ADP) learning scheme to address the estimation bias issue encountered in ADP based learning control methods. We consider the classic case of model-free linear quadratic regulator (LQR) augmented with integral control to show that the integral action alone may not be sufficient to prevent estimation bias induced by unmeasurable disturbances in the associated learning equation. It is shown that the presence of unmeasurable disturbances can lead to bias in the estimates of the optimal control parameters, and in extreme cases, the divergence of the algorithm and instability of the system. To address this difficulty, we present a bias compensating ADP learning equation that learns a lumped bias term as a result of disturbances (and possibly other sources) in conjunction with the optimal control parameters. An extension of the standard ADP LQR policy iteration algorithm is presented based on this compensated learning equation. We demonstrate by numerical examples that compared to the uncompensated algorithm, the proposed scheme learns the optimal control parameters without incurring bias.
更多查看译文
关键词
disturbance induced estimation bias,optimal control,dynamic
AI 理解论文
溯源树
样例
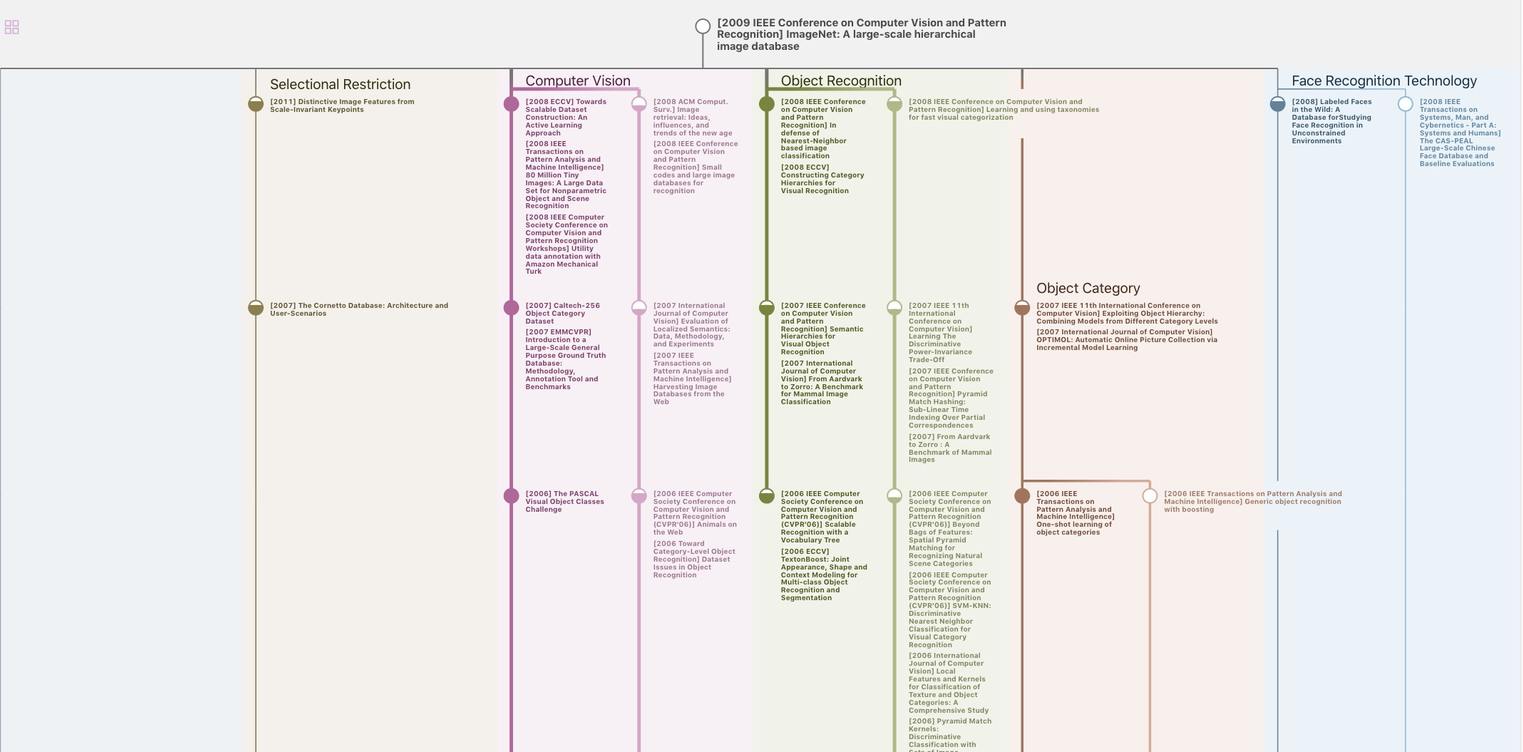
生成溯源树,研究论文发展脉络
Chat Paper
正在生成论文摘要